Hybrid Quantum–classical Generative Adversarial Networks for Image Generation Via Learning Discrete Distribution
Signal Processing: Image Communication(2023)CCF CSCI 2区SCI 3区
Shanghai Univ Engn Sci | Nanchang Univ
Abstract
It has been reported that quantum generative adversarial networks have a potential exponential advantage over classical generative adversarial networks. However, quantum machine learning is difficult to find real applications in the near future due to the limitation of quantum devices. The structure of quantum generator is optimized to reduce the required parameters and make use of quantum devices to a greater extent. And an image generation scheme is designed based on quantum generative adversarial networks. Two structures of quantum generative adversarial networks are simulated on Bars and Stripes dataset, and the results corroborate that the quantum generator with reduced parameters has no visible performance loss. The original complex multimodal distribution of an image can be converted into a simple unimodal distribution by the remapping method. The MNIST images and the Fashion-MNIST images are successfully generated by the optimized quantum generator with the remapping method, which verified the feasibility of the proposed image generation scheme.
MoreTranslated text
Key words
Quantum machine learning,Quantum generative adversarial network,Quantum computation,Image generation,Hybrid quantum–classical algorithms
求助PDF
上传PDF
View via Publisher
AI Read Science
AI Summary
AI Summary is the key point extracted automatically understanding the full text of the paper, including the background, methods, results, conclusions, icons and other key content, so that you can get the outline of the paper at a glance.
Example
Background
Key content
Introduction
Methods
Results
Related work
Fund
Key content
- Pretraining has recently greatly promoted the development of natural language processing (NLP)
- We show that M6 outperforms the baselines in multimodal downstream tasks, and the large M6 with 10 parameters can reach a better performance
- We propose a method called M6 that is able to process information of multiple modalities and perform both single-modal and cross-modal understanding and generation
- The model is scaled to large model with 10 billion parameters with sophisticated deployment, and the 10 -parameter M6-large is the largest pretrained model in Chinese
- Experimental results show that our proposed M6 outperforms the baseline in a number of downstream tasks concerning both single modality and multiple modalities We will continue the pretraining of extremely large models by increasing data to explore the limit of its performance
Upload PDF to Generate Summary
Must-Reading Tree
Example
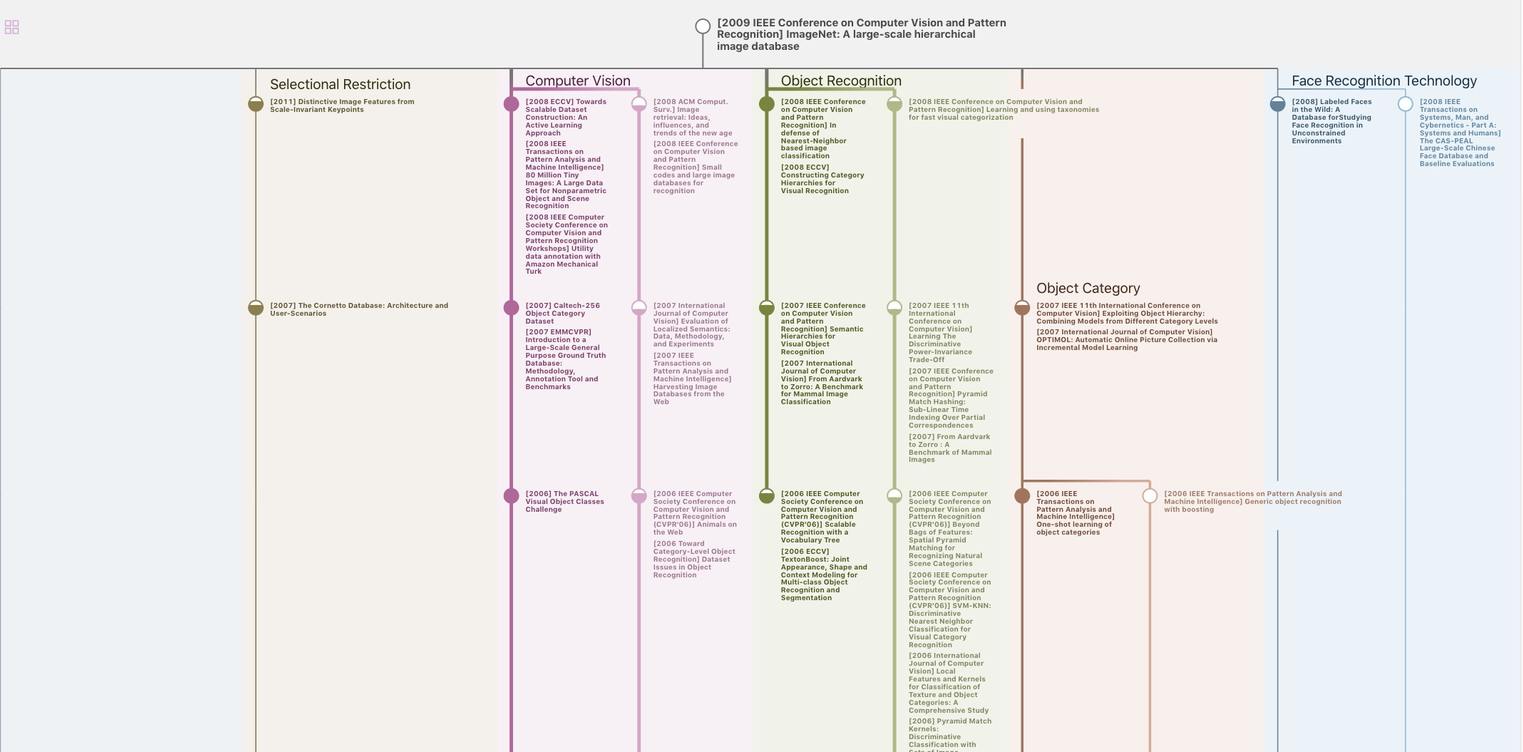
Generate MRT to find the research sequence of this paper
Related Papers
2017
被引用646 | 浏览
2021
被引用12 | 浏览
2021
被引用11 | 浏览
Data Disclaimer
The page data are from open Internet sources, cooperative publishers and automatic analysis results through AI technology. We do not make any commitments and guarantees for the validity, accuracy, correctness, reliability, completeness and timeliness of the page data. If you have any questions, please contact us by email: report@aminer.cn
Chat Paper