Multitasking optimization via an adaptive solver multitasking evolutionary framework
Information Sciences(2023)
摘要
Multitasking optimization (MTO) aims to solve multiple tasks simultaneously in a single run. Many multitasking evolutionary algorithms (MTEAs) have been developed in recent years for solving MTO problems. Existing MTEAs typically use a single solver to handle multiple optimi-zation tasks. However, different tasks have distinct characteristics, such as convex, nonconvex, and multimodal. If one could automatically find a best-fitting solver for each task, it would be more efficient to solve different tasks. To this end, we propose a multitasking evolutionary framework based on adaptive solver selection, namely MTEA-SaO, where a suitable knowledge transfer strategy is embedded. The proposed method can be featured as 1) It explicitly assigns several solver subpopulations to each task and adaptively picks a best-fitting solver for each task, and 2) It enables knowledge transfer using the implicit similarities between tasks. The experi-mental results have demonstrated the effectiveness of solver adaptation and knowledge transfer strategies and the overall superior performance of MTEA-SaO.
更多查看译文
关键词
Evolutionary multitasking,Multitasking optimization,Solver adaptation,Evolutionary transfer optimization
AI 理解论文
溯源树
样例
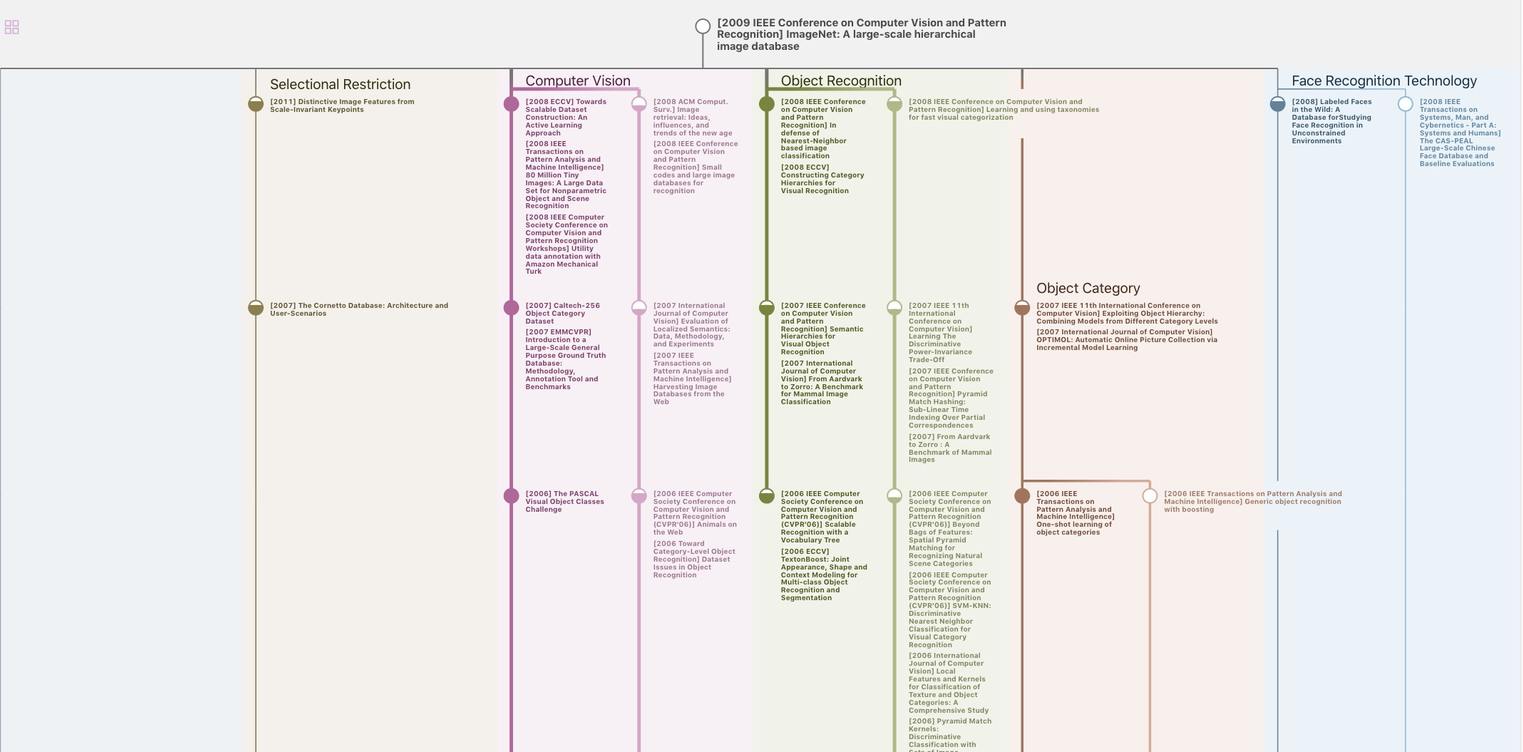
生成溯源树,研究论文发展脉络
Chat Paper
正在生成论文摘要