Relative Attention-based One-Class Adversarial Autoencoder for Continuous Authentication of Smartphone Users
arxiv(2022)
摘要
Behavioral biometrics-based continuous authentication is a promising authentication scheme, which uses behavioral biometrics recorded by built-in sensors to authenticate smartphone users throughout the session. However, current continuous authentication methods suffer some limitations: 1) behavioral biometrics from impostors are needed to train continuous authentication models. Since the distribution of negative samples from diverse attackers are unknown, it is a difficult problem to solve in real-world scenarios; 2) most deep learning-based continuous authentication methods need to train two models to improve authentication performance. A deep learning model for deep feature extraction, and a machine learning-based classifier for classification; 3) weak capability of capturing users' behavioral patterns leads to poor authentication performance. To solve these issues, we propose a relative attention-based one-class adversarial autoencoder for continuous authentication of smartphone users. First, we propose a one-class adversarial autoencoder to learn latent representations of legitimate users' behavioral patterns, which is trained only with legitimate smartphone users' behavioral biometrics. Second, we present the relative attention layer to capture richer contextual semantic representation of users' behavioral patterns, which modifies the standard self-attention mechanism using convolution projection instead of linear projection to perform the attention maps. Experimental results demonstrate that we can achieve superior performance of 1.05 1.09 public datasets.
更多查看译文
AI 理解论文
溯源树
样例
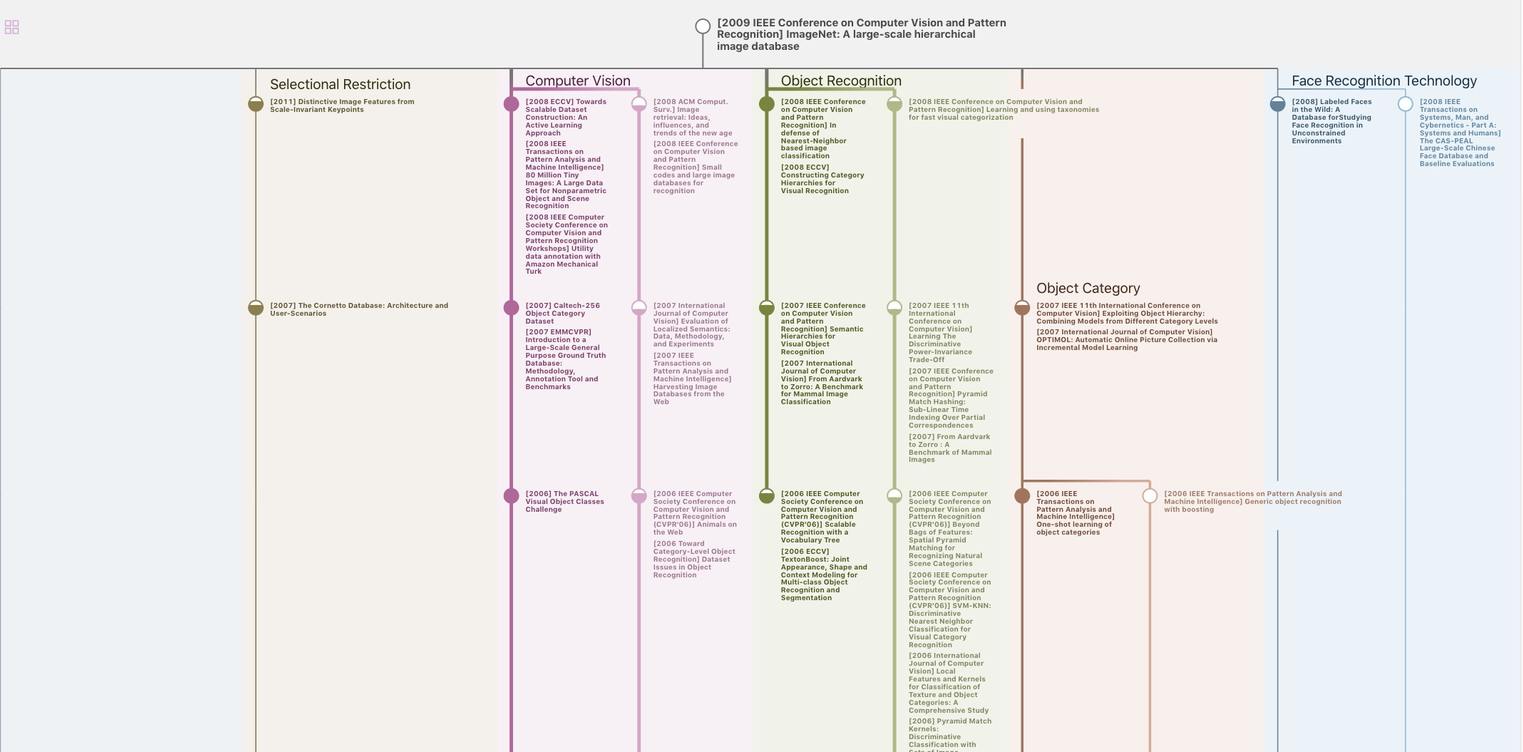
生成溯源树,研究论文发展脉络
Chat Paper
正在生成论文摘要