DOMAINDESC: Learning Local Descriptors with Domain Adaptation.
ICASSP 2022 - 2022 IEEE International Conference on Acoustics, Speech and Signal Processing (ICASSP)(2022)
摘要
Robust and efficient local descriptor is crucial in a wide range of applications. In this paper, we propose a novel descriptor DomainDesc which is invariant as much as possible by learning local Descriptor with Domain adaptation. We design the feature-level domain adaptation loss to improve robustness of our DomainDesc by punishing inconsistent high-level feature distributions of different images, while we present the pixel-level cross-domain consistency loss to compensate for the inconsistency between the descriptors corresponding to the keypoints at the pixel level. Besides, we adopt a new architecture to make the descriptor contain as much information as possible, and combine triplet loss and cross-domain consistency loss for descriptor supervision to ensure the distinguished ability of our descriptor. Finally, we give a cross-domain dataset generation strategy to quickly construct our training dataset for diverse domains to adapt to complex application scenarios. Experiments validate that our DomainDesc achieves state-of-the-art performances on HPatches image matching benchmark and Aachen-Day-Night localization benchmark.
更多查看译文
关键词
local descriptors,domain adaptation,cross-domain data,consistency loss
AI 理解论文
溯源树
样例
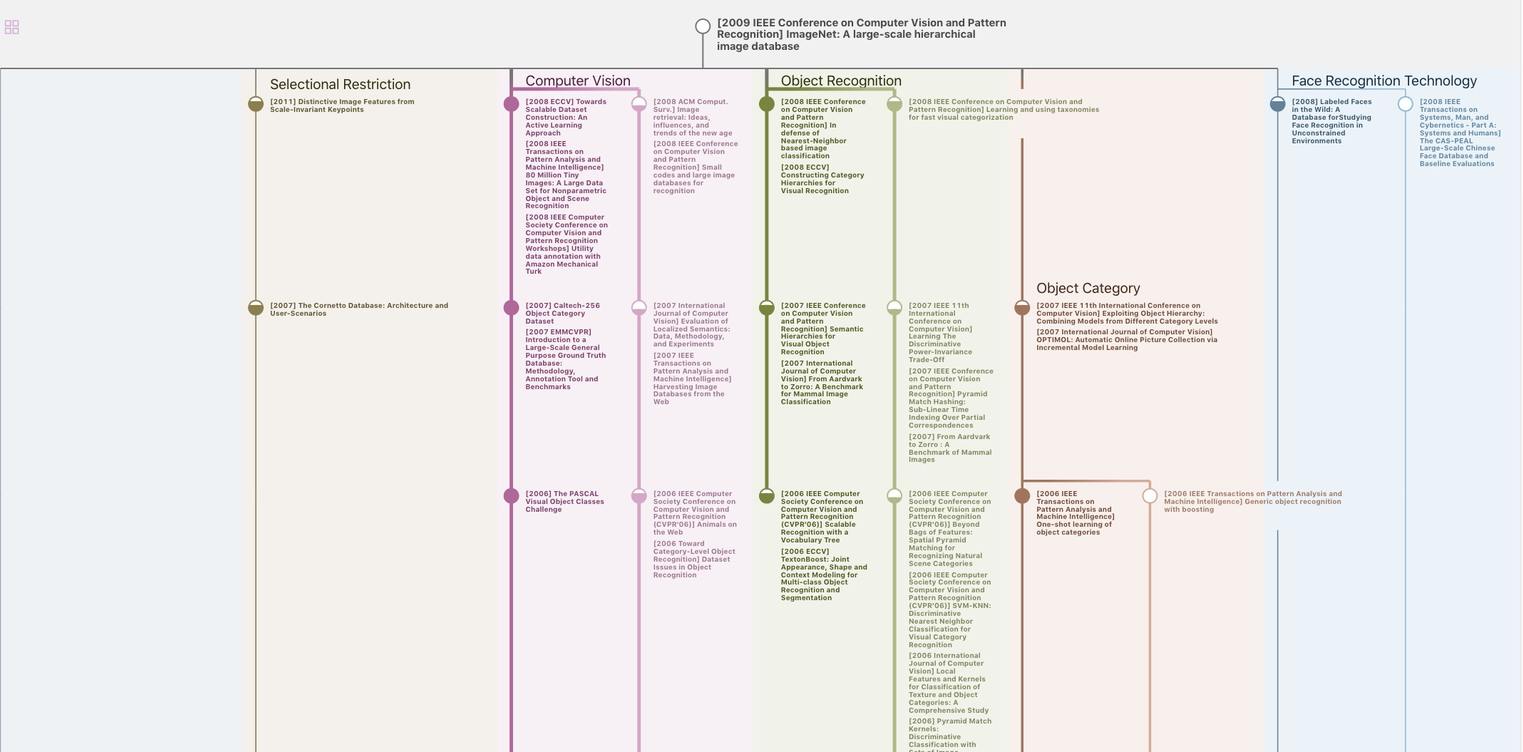
生成溯源树,研究论文发展脉络
Chat Paper
正在生成论文摘要