Editorial for “multi‐sequence and Multi‐Regional MRI‐Based Radiomics Nomogram for the Preoperative Assessment of Muscle Invasion in Bladder Cancer”
Journal of magnetic resonance imaging(2022)
Abstract
As stated by Wang et al, 1 bladder cancer is the fourth most common tumor in men and the eighth most lethal. This kind of tumor can be subdivided into invasive or noninvasive of the muscle tissue surrounding the bladder. The difference in treatments between each kind of tumor, especially the 5-year survival rate, is considerable (90% for noninvasive vs. 50% for invasive). Even if tissue biopsy remains the golden standard to classify these tumors, differences in tissue obtention techniques as well as the limited number of samples acquired per patient make it a solution open to improvement. Developing a classification tool for these tumors is of high clinical interest, especially if this tool is noninvasive, nonpainful, covers the whole area of study, and shows a specificity over 90%. Plenty of work in this field has been performed to combine MR imaging techniques that are non-painful, noninvasive, and able to cover all the different tissues involved in diagnostics. The use of these techniques alone has allowed researchers and clinicians to develop diagnostic algorithms with a success rate between 64.7% and 87%. On top of them, radiomic analyses are a set of up-and-coming methodologies, able to obtain image features that can later be used to perform differential diagnostics. In fact, in the past, radiomics has been applied in this field to distinguish the two subtypes of cancer and to grade the tumor stage with different rates of success, usually under 80%. In this issue of JMRI, Wang et al obtained radiomic features of MR images, to assess the stage of bladder tumors and their muscle infiltration. The difference with previous works resides in the fact that here authors use a larger database of MR images obtained with different sequences and contrasts, leading to also to a larger body of radiomic features/variables. Also, and on top of this, they develop a nomogram that could further complement the diagnosis and staging of these illnesses, as it combined not just the radiomic features described before, but extra clinic-radiological variables. One of the strengths of this paper is the amount of data and statistical strength of its implementation. The authors use data from 234 patients, of which 103 are used to validate models and 239 to build them. Almost 7900 radiomic features were extracted from each one of the three volumes of interest used in this analysis, 23 different clinic-radiological features for each volunteer. Also, the authors use appropriately strong mathematical and statistical methodology to reduce the number of variables in their models (i.e., LASSO, multivariate logistic regression, recursive feature elimination, etc.). After all this statistical analysis, only nine radiomic features were used for the radiomic model and five for the clinicradiological counterpart. Wang et al produce, at the end of this project, three models to predict tumor muscle infiltration. The radiomic model is based on nine features, which are presented in Table 2 of the manuscript. This is a strong point of this work as it will allow readers to see the coefficients and replicate the findings. This is not usual in other publications in the field. The sensitivity of this model was 87.50%, and the specificity was 82.28%. The area under the curve (AUC) for the training data was 0.933 and 0.916 for the testing data. The clinical model on its own was based on five parameters, which are presented in Table 3. Training model specificity AUC scores were 0.876 and 0.840 for the testing data. When the radiomic-clinical nomogram was built, receiver operating characteristic curve was 0.955 for the training data and 0.924 for the testing data. This shows that both the radiomic and the radiomic-clinical models outperformed the clinical model. Secondary conclusions also derived from this work are that high-density protein content was negatively correlated with myometrial invasion in these kinds of tumors. Tumor volume and tumor stalk structure were also two variables of special interest and weight in the models developed. The authors present the built nomogram in the last figure of this paper. Finally, the authors explain the limitations of this study. They include as future improvements the use of multicenter data,
MoreTranslated text
Key words
bladder cancer,magnetic resonance imaging,radiomics,muscle invasion,tumor staging
AI Read Science
Must-Reading Tree
Example
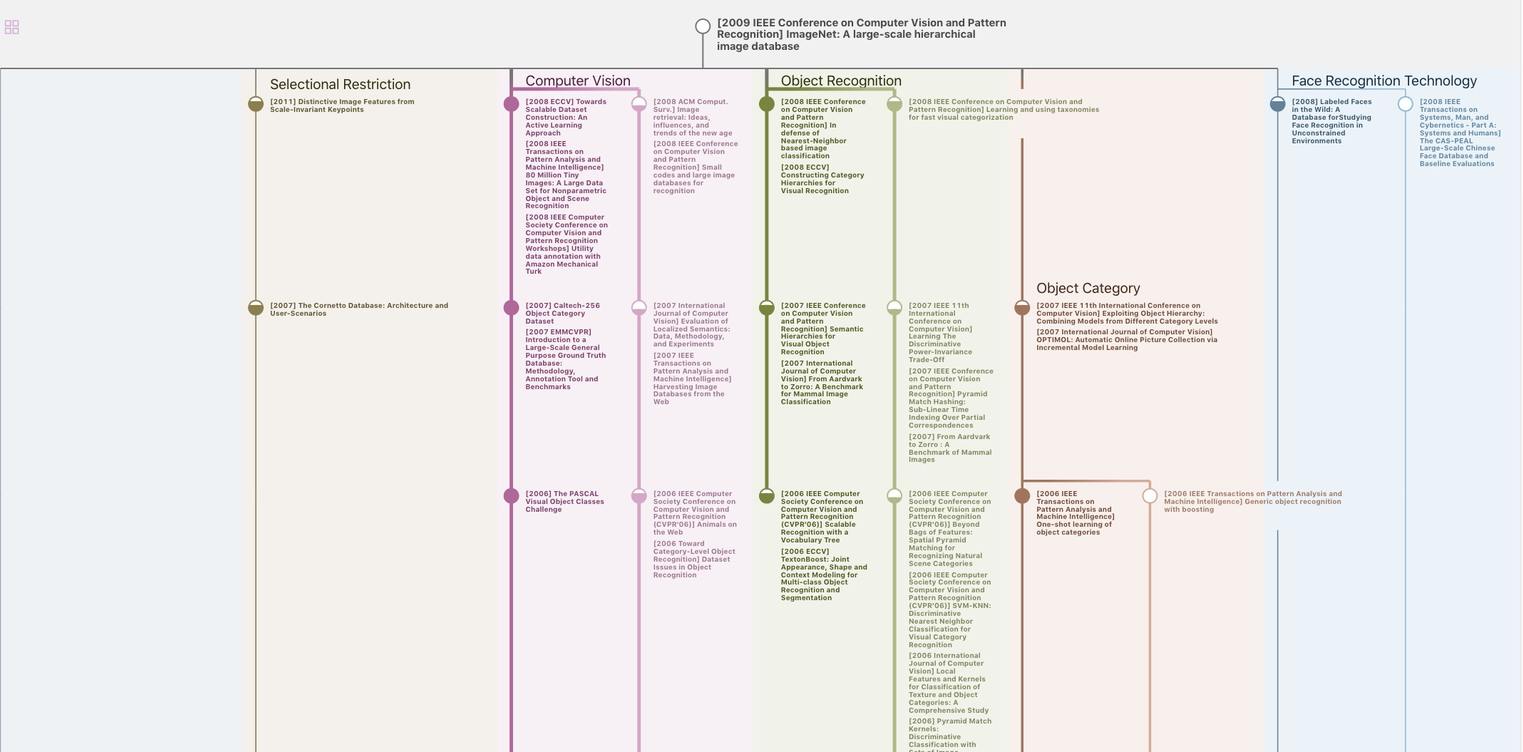
Generate MRT to find the research sequence of this paper
Chat Paper
Summary is being generated by the instructions you defined