Transformer Networks of Human Conceptual Knowledge.
PSYCHOLOGICAL REVIEW(2024)
摘要
We present a computational model capable of simulating aspects of human knowledge for thousands of real-world concepts. Our approach involves a pretrained transformer network that is further fine-tuned on large data sets of participant-generated feature norms. We show that such a model can successfully extrapolate from its training data, and predict human knowledge for new concepts and features. We apply our model to stimuli from 25 previous experiments in semantic cognition research and show that it reproduces many findings on semantic verification, concept typicality, feature distribution, and semantic similarity. We also compare our model against several variants, and by doing so, establish the model properties that are necessary for good prediction. The success of our approach shows how a combination of language data and (laboratory-based) psychological data can be used to build models with rich world knowledge. Such models can be used in the service of new psychological applications, such as the modeling of naturalistic semantic verification and knowledge retrieval, as well as the modeling of real-world categorization, decision-making, and reasoning. (PsycInfo Database Record (c) 2024 APA, all rights reserved).
更多查看译文
关键词
Interpretable Models,Model Interpretability,Machine Learning Interpretability,Topic Modeling,Pretrained Models
AI 理解论文
溯源树
样例
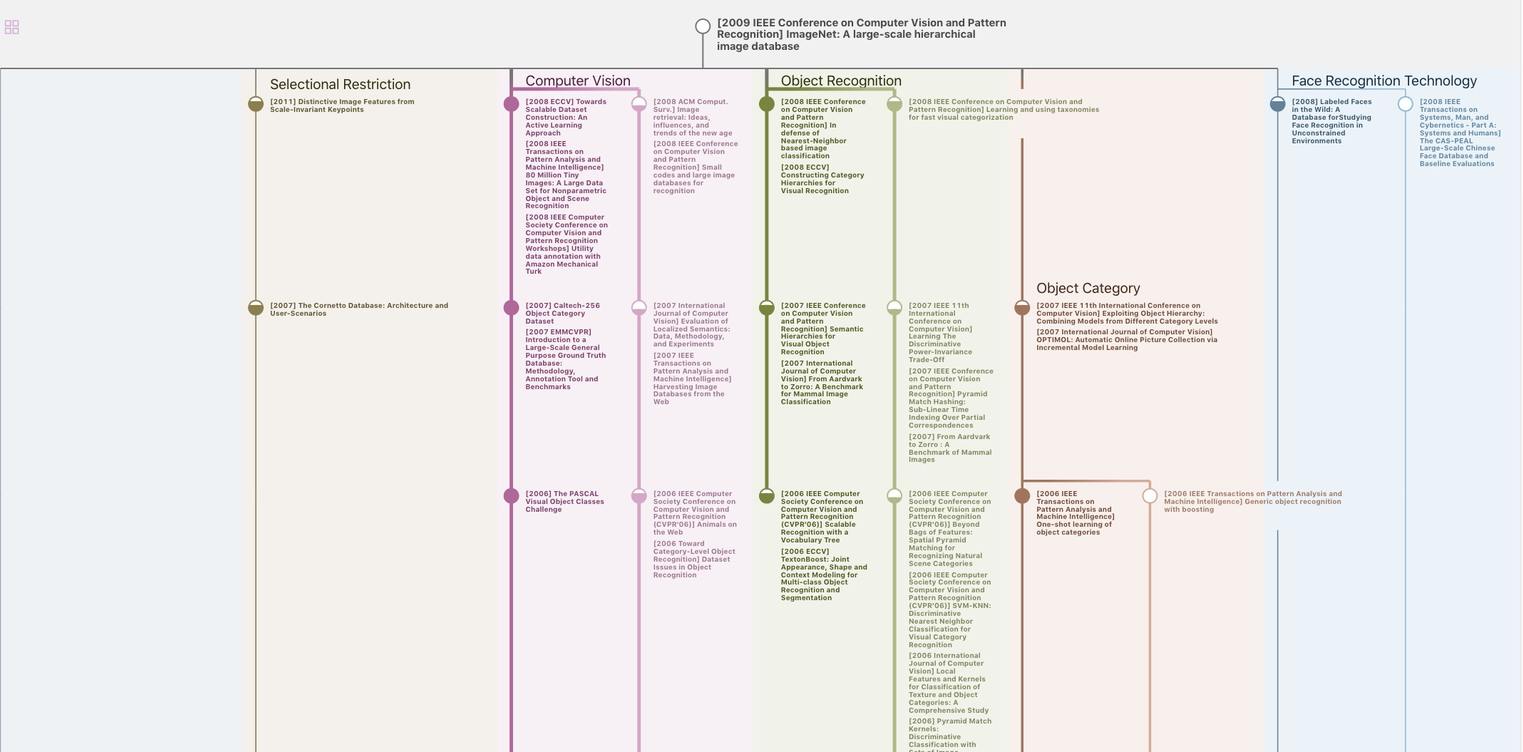
生成溯源树,研究论文发展脉络
Chat Paper
正在生成论文摘要