FO-PINNs: A First-Order formulation for Physics Informed Neural Networks
CoRR(2022)
摘要
We present FO-PINNs, physics-informed neural networks that are trained using the first-order formulation of the Partial Differential Equation (PDE) losses. We show that FO-PINNs offer significantly higher accuracy in solving parameterized systems compared to traditional PINNs, and reduce time-per-iteration by removing the extra backpropagations needed to compute the second or higher-order derivatives. Additionally, unlike standard PINNs, FO-PINNs can be used with exact imposition of boundary conditions using approximate distance functions, and can be trained using Automatic Mixed Precision (AMP) to further speed up the training. Through two Helmholtz and Navier-Stokes examples, we demonstrate the advantages of FO-PINNs over traditional PINNs in terms of accuracy and training speedup.
更多查看译文
关键词
neural networks,physics,fo-pinns,first-order
AI 理解论文
溯源树
样例
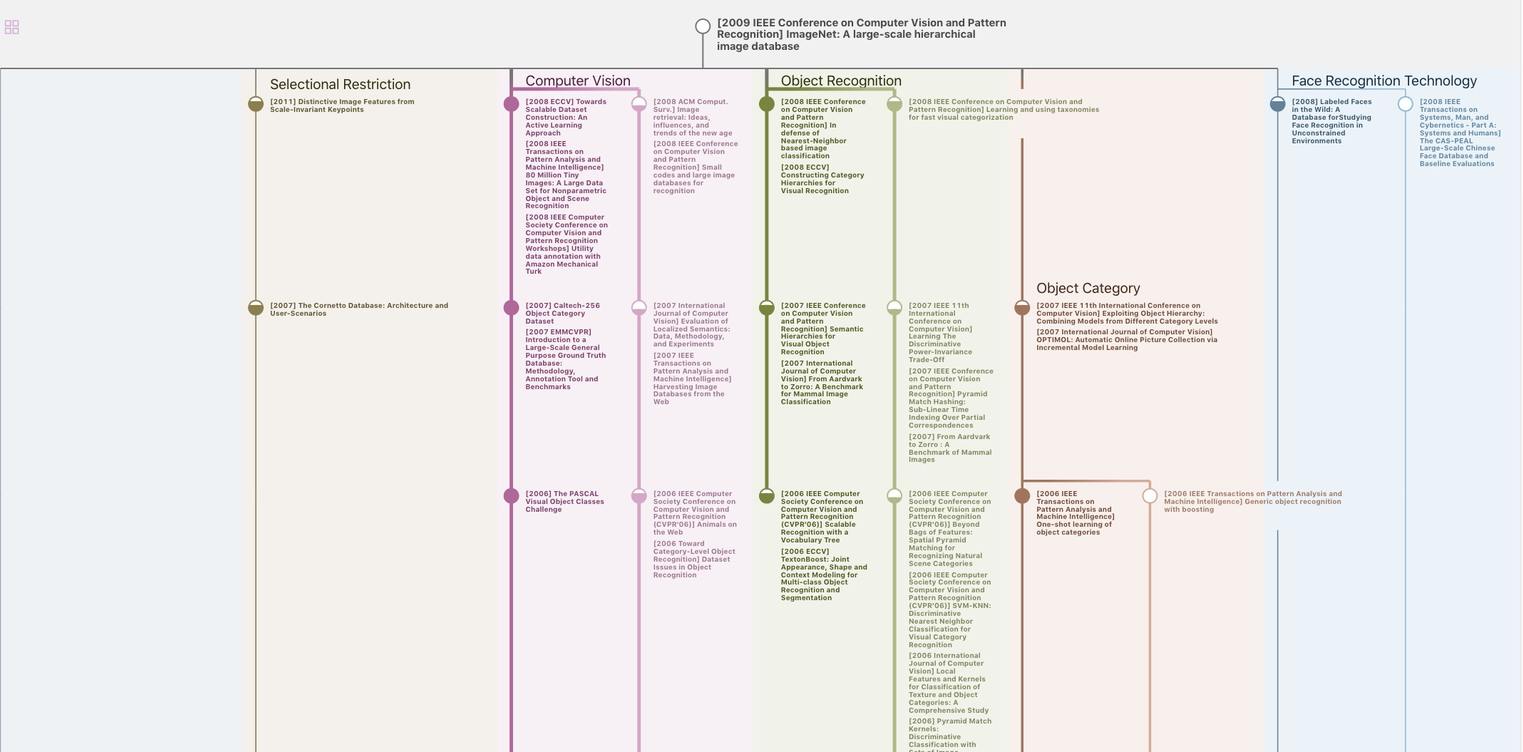
生成溯源树,研究论文发展脉络
Chat Paper
正在生成论文摘要