An Opponent-Aware Reinforcement Learning Method for Team-to-Team Multi-Vehicle Pursuit Via Maximizing Mutual Information Indicator.
International Conference on Mobile Ad-hoc and Sensor Networks(2022)
摘要
The pursuit-evasion game in Smart City brings a profound impact on the Multi-vehicle Pursuit (MVP) problem, when police cars cooperatively pursue suspected vehicles. Existing studies on the MVP problems tend to set evading vehicles to move randomly or in a fixed prescribed route. The opponent modeling method has proven considerable promise in tackling the non-stationary caused by the adversary agent. However, most of them focus on two-player competitive games and easy scenarios without the interference of environments. This paper considers a Team-to-Team Multi-vehicle Pursuit (T2TMVP) problem in the complicated urban traffic scene where the evading vehicles adopt the pre-trained dynamic strategies to execute decisions intelligently. To solve this problem, we propose an opponent-aware reinforcement learning via maximizing mutual information indicator (OARLM 2 I 2 ) method to improve pursuit efficiency in the complicated environment. First, a sequential encoding-based opponents joint strategy modeling (SEOJSM) mechanism is proposed to generate evading vehicles' joint strategy model, which assists the multi-agent decision-making process based on deep Q-network (DQN). Then, we design a mutual information-united loss, simultaneously considering the reward fed back from the environment and the effectiveness of opponents' joint strategy model, to update pursuing vehicles' decision-making process. Extensive experiments based on SUMO demonstrate our method outperforms other baselines by 21.48% on average in reducing pursuit time. The code is available at https://github.com/ANT-ITS/OARLM2I2.
更多查看译文
关键词
intelligent transportation,team-to-team multi-vehicle pursuit,multi-agent reinforcement pursuit
AI 理解论文
溯源树
样例
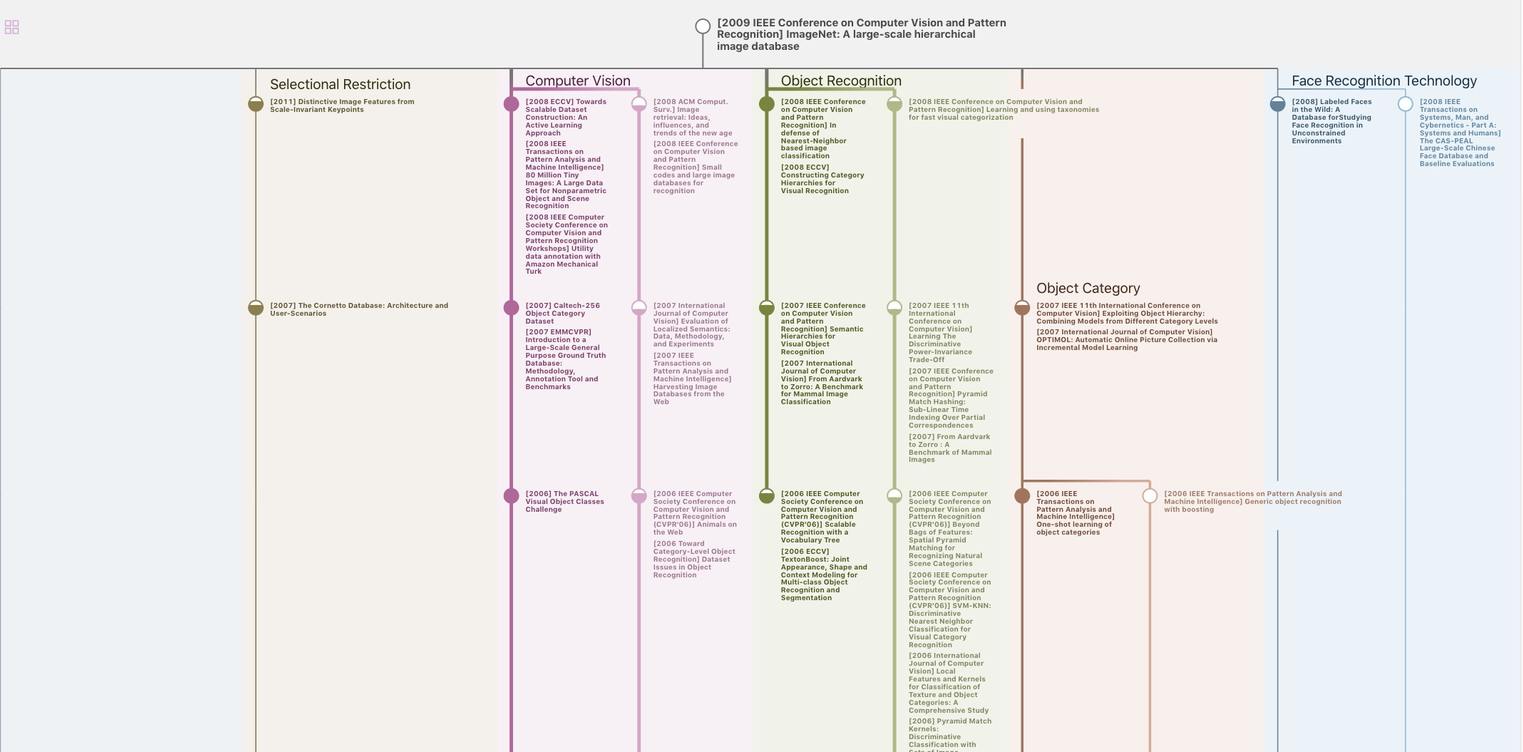
生成溯源树,研究论文发展脉络
Chat Paper
正在生成论文摘要