MetaASSIST: Robust Dialogue State Tracking with Meta Learning
emnlp 2022(2022)
摘要
Existing dialogue datasets contain lots of noise in their state annotations. Such noise can hurt model training and ultimately lead to poor generalization performance. A general framework named ASSIST has recently been proposed to train robust dialogue state tracking (DST) models. It introduces an auxiliary model to generate pseudo labels for the noisy training set. These pseudo labels are combined with vanilla labels by a common fixed weighting parameter to train the primary DST model. Notwithstanding the improvements of ASSIST on DST, tuning the weighting parameter is challenging. Moreover, a single parameter shared by all slots and all instances may be suboptimal. To overcome these limitations, we propose a meta learning-based framework MetaASSIST to adaptively learn the weighting parameter. Specifically, we propose three schemes with varying degrees of flexibility, ranging from slot-wise to both slot-wise and instance-wise, to convert the weighting parameter into learnable functions. These functions are trained in a meta-learning manner by taking the validation set as meta data. Experimental results demonstrate that all three schemes can achieve competitive performance. Most impressively, we achieve a state-of-the-art joint goal accuracy of 80.10% on MultiWOZ 2.4.
更多查看译文
关键词
robust dialogue state tracking,metaassist learning
AI 理解论文
溯源树
样例
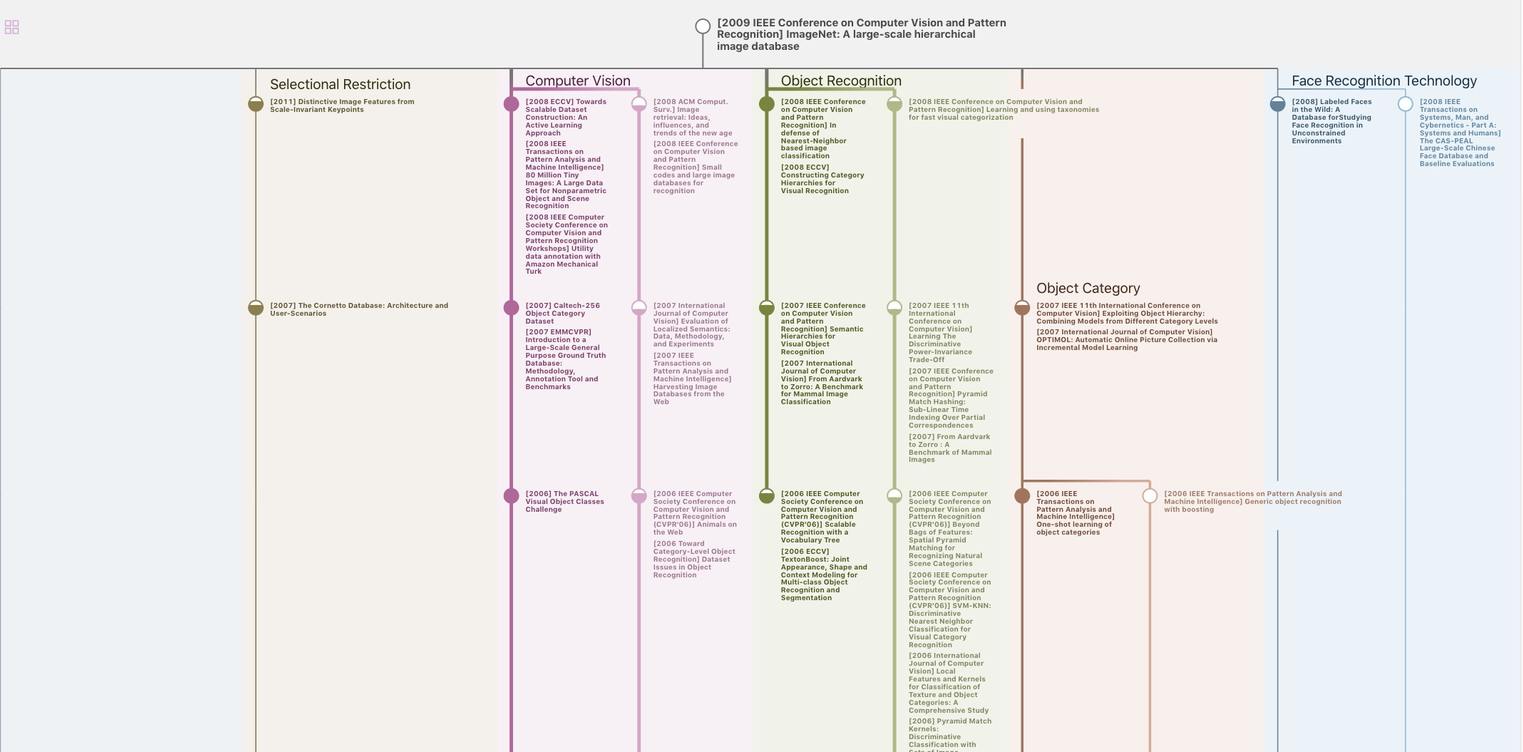
生成溯源树,研究论文发展脉络
Chat Paper
正在生成论文摘要