CNN-based Shape Estimation for Extended Object Tracking using Point Cloud Measurements
2022 25TH INTERNATIONAL CONFERENCE ON INFORMATION FUSION (FUSION 2022)(2022)
摘要
Simultaneously estimating the kinematic properties and the spatial extent of an object based on noisy point clouds is a ubiquitous problem in a variety of environment perception applications. For this purpose, we propose a novel approach for elliptical extended objects that employs a Kalman filter to estimate the kinematic state and a neural network architecture to infer the axes length. The key idea is to transform the point cloud measurement to a two-channel image, which serves as the input to a convolutional neural network. This input image incorporates uncertainties and is recursively updated as new measurements become available. The network is trained with simulated data using varying measurement noise levels, where measurements are assumed to be uniformly distributed across the elliptical object's surface. Noisy simulated data is used to evaluate the proposed method, and it is found to perform competitively with state-of-the-art methods. In the considered challenging scenarios with low measurement rate, it is even capable of outperforming them.
更多查看译文
关键词
Convolutional Neural Networks,Bayesian Filtering,Extended Object Tracking
AI 理解论文
溯源树
样例
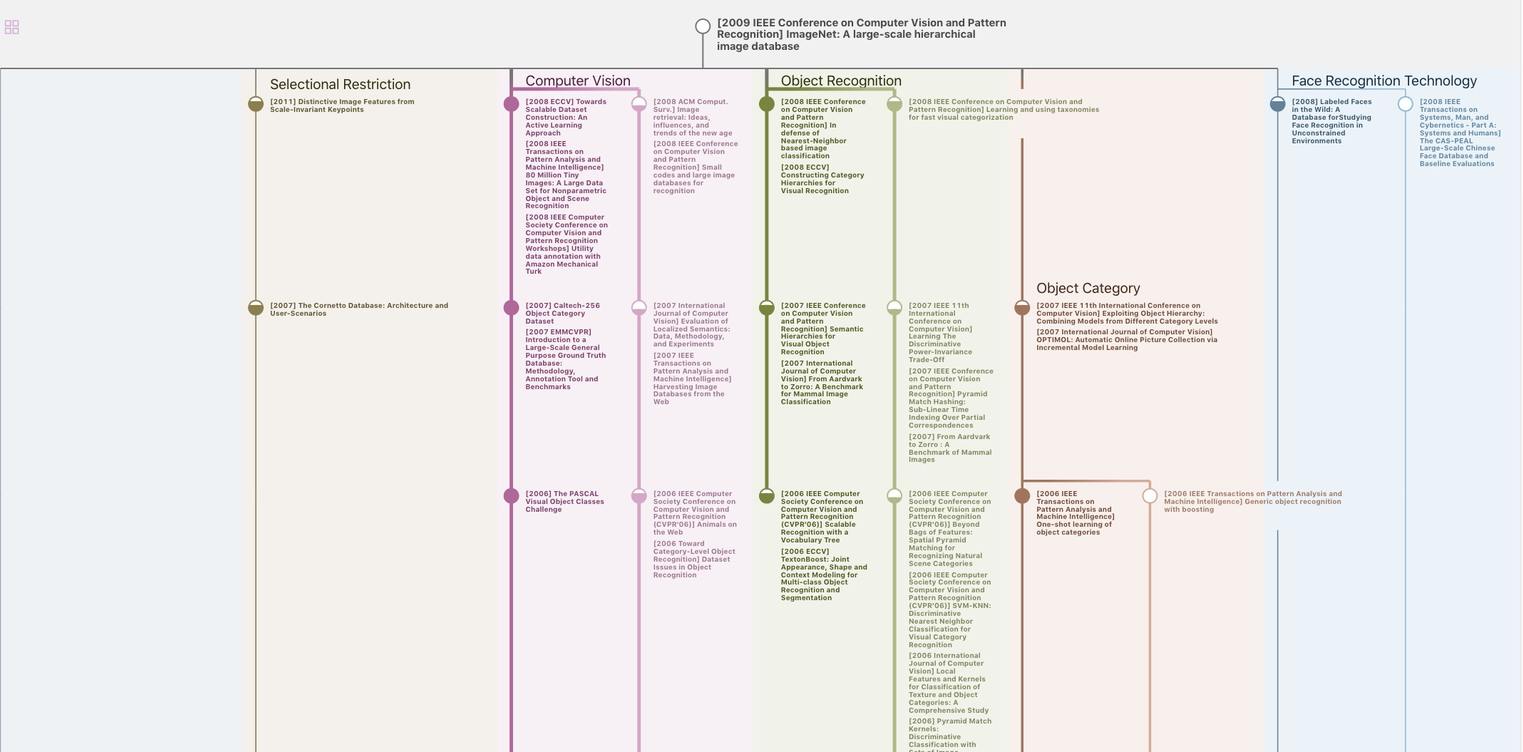
生成溯源树,研究论文发展脉络
Chat Paper
正在生成论文摘要