A Kernel Extreme Learning Machine-Grey Wolf Optimizer (KELM-GWO) Model to Predict Uniaxial Compressive Strength of Rock
APPLIED SCIENCES-BASEL(2022)
摘要
Uniaxial compressive strength (UCS) is one of the most important parameters to characterize the rock mass in geotechnical engineering design and construction. In this study, a novel kernel extreme learning machine-grey wolf optimizer (KELM-GWO) model was proposed to predict the UCS of 271 rock samples. Four parameters namely the porosity (P-n, %), Schmidt hardness rebound number (SHR), P-wave velocity (V-p, km/s), and point load strength (PLS, MPa) were considered as the input variables, and the UCS is the output variable. To verify the effectiveness and accuracy of the KELM-GWO model, extreme learning machine (ELM), KELM, deep extreme learning machine (DELM) back-propagation neural network (BPNN), and one empirical model were established and compared with the KELM-GWO model to predict the UCS. The root mean square error (RMSE), determination coefficient (R-2), mean absolute error (MAE), prediction accuracy (U-1), prediction quality (U-2), and variance accounted for (VAF) were adopted to evaluate all models in this study. The results demonstrate that the proposed KELM-GWO model was the best model for predicting UCS with the best performance indices. Additionally, the identified most important parameter for predicting UCS is the porosity by using the mean impact value (MIV) technique.
更多查看译文
关键词
uniaxial compressive strength (UCS),grey wolf optimizer (GWO),kernel extreme learning machine (KELM),mean impact value (MIV)
AI 理解论文
溯源树
样例
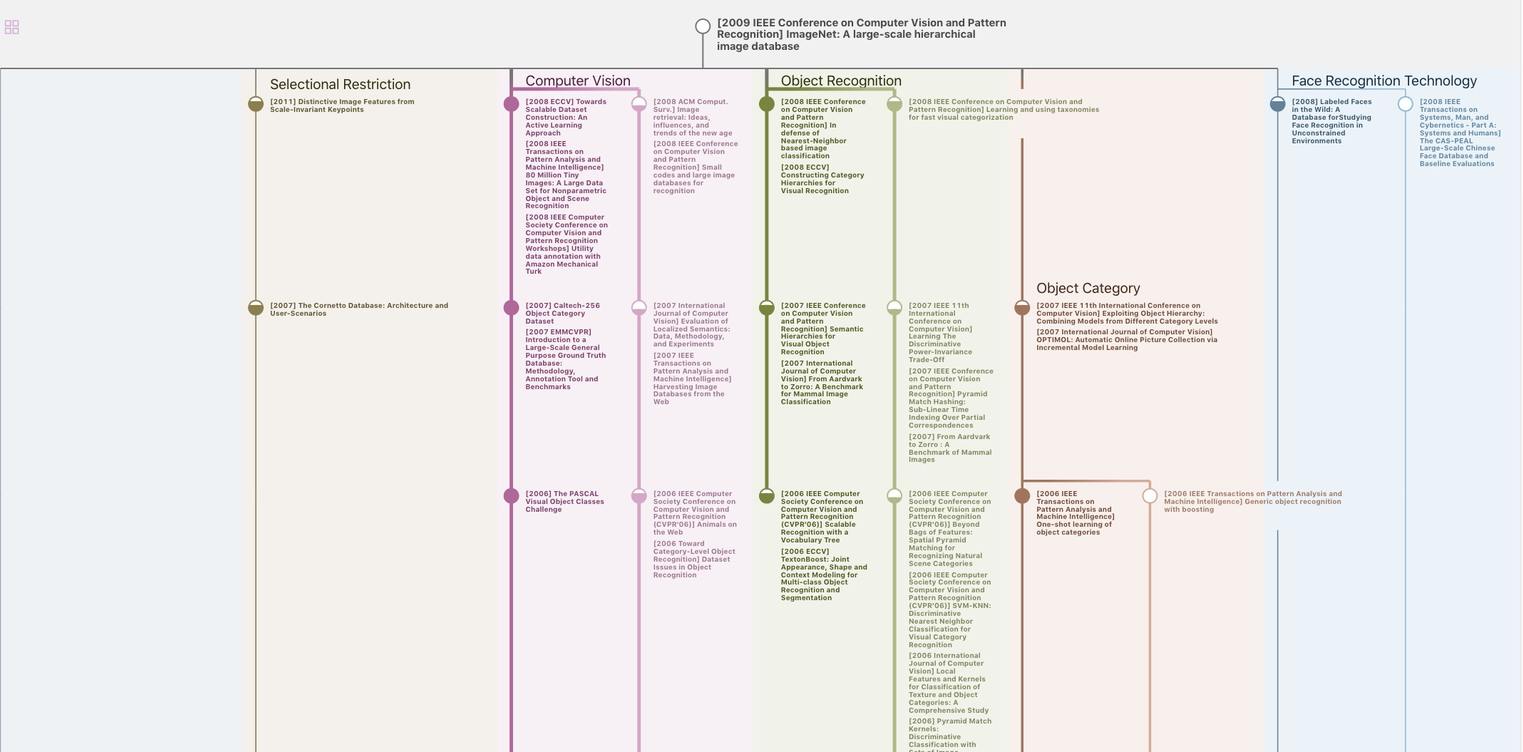
生成溯源树,研究论文发展脉络
Chat Paper
正在生成论文摘要