Self-Optimization for Parsing Floor Plans.
Journal of computing in civil engineering(2022)
摘要
Floor plan vectorization is an emerging research area in geographic information science and computer vision. However, automated recognition of building elements remains a challenge. This work proposes a method that combines the advantages of classical graphics with deep learning. Specifically, a morphological template is introduced to optimize topological relations, enhance completeness, and suppress conflicts. Bezier curves are utilized to represent irregularity contributing to improving visual effects and experimental accuracy. Thus, the proposed method can be directly practiced to boost performance and correct pseudo-samples in self-training. Experiments demonstrate that the proposed method achieves a considerable improvement in CVC-FP and R2V benchmarks. Additionally, our approach outputs instances with consistent topology, enabling direct modeling into Industry Foundation Classes (IFC) or City Geography Markup Language (CityGML). Hopefully, this work can serve as a new baseline for further study. (C) 2022 American Society of Civil Engineers.
更多查看译文
AI 理解论文
溯源树
样例
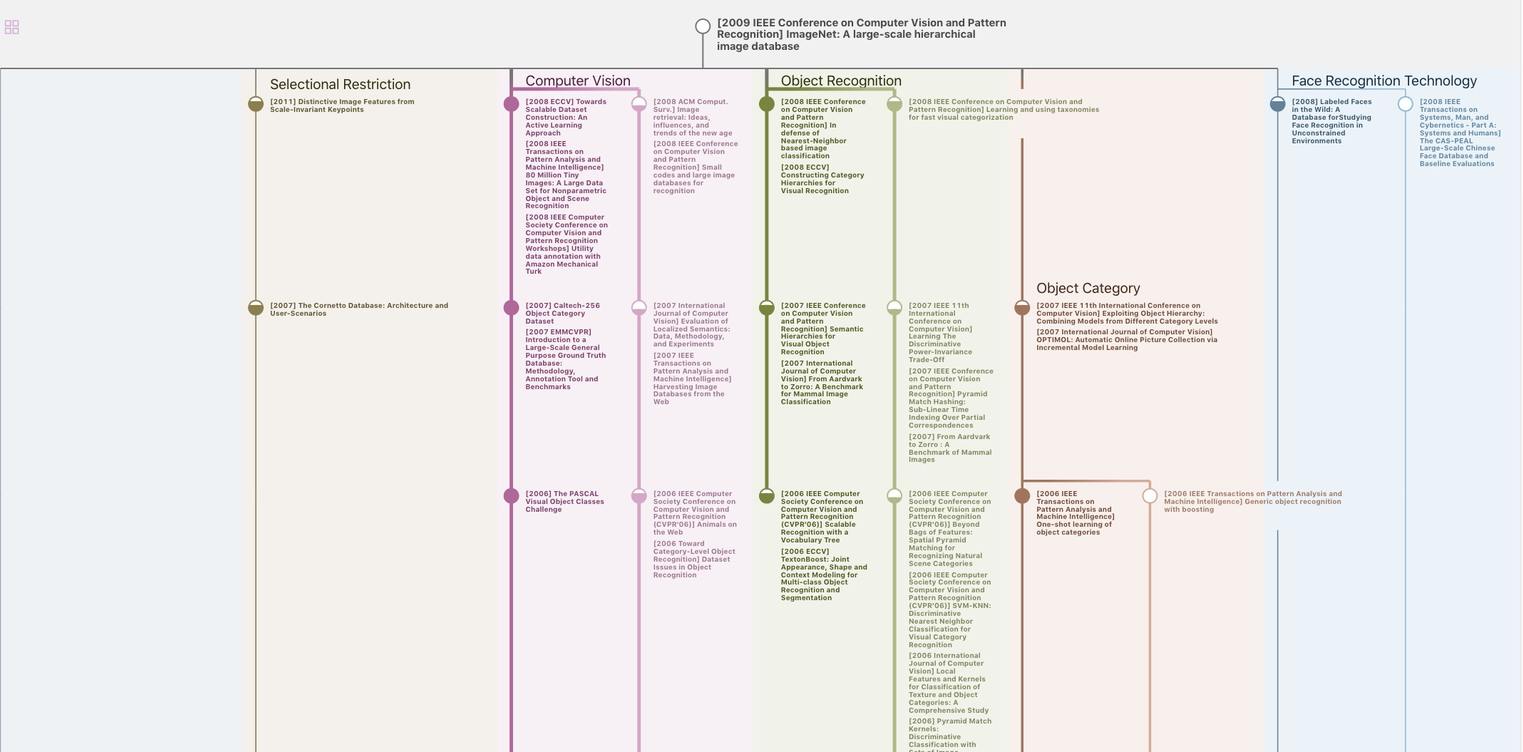
生成溯源树,研究论文发展脉络
Chat Paper
正在生成论文摘要