Hardening Hardware Accelerartor Based CNN Inference Phase Against Adversarial Noises
2022 IEEE INTERNATIONAL SYMPOSIUM ON HARDWARE ORIENTED SECURITY AND TRUST (HOST)(2022)
摘要
Recent research has shown that Convolution Neu-ral Networks (CNNs) are vulnerable to adversarial examples. Many defense techniques like gradient masking etc have been proposed against adversarial attacks. However, these techniques are limited to training methods and do not offer generalizability. Similarly, in a Horizontal Collaborative Environment (HCE) where trained CNN models are partitioned into different layers, models deployed are also subjected to attacks by adversarial inputs. In this work, we develop a defense strategy to harden CNNs in an HCE against adversarial examples through the detection of adversarial inputs. We propose the notion that by obtaining model prediction at different layers of the CNN and noting the prediction inconsistency, an adversarial noise could be detected. In this work, adversarial noises are generated using the Fast Gradient Sign Method (FGSM), Salt and Pepper (S&P), and Gaussian Noise perturbation (GNP) methodologies. We compare predictions at different layers of a CNN and obtain final prediction via coherence in predicted class of the CNN model. The hardware synthesis results on FPGA, validated proposed method showing that obtaining such accuracy inconsistency require reasonable hardware overhead.
更多查看译文
关键词
edge intelligence,CNN,adversarial attack
AI 理解论文
溯源树
样例
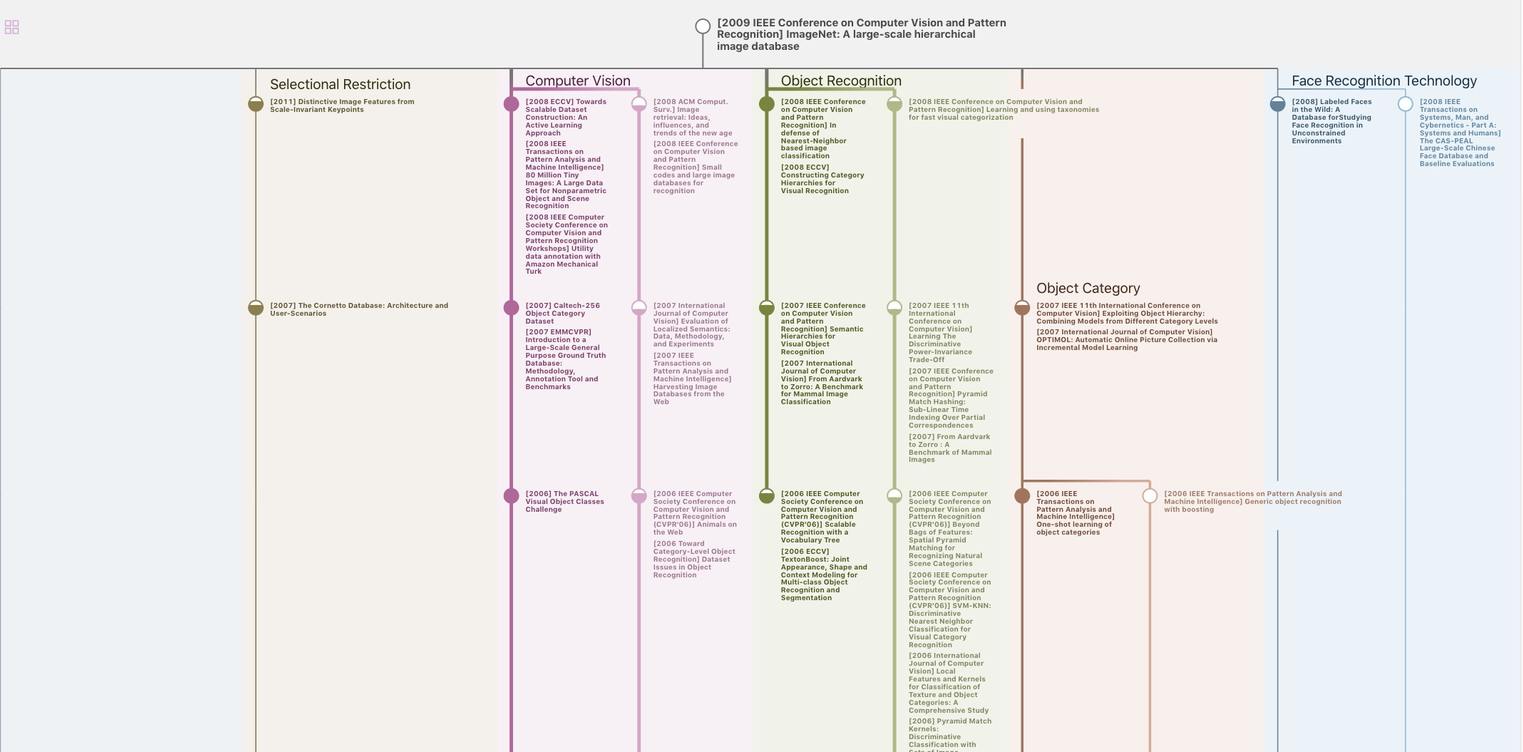
生成溯源树,研究论文发展脉络
Chat Paper
正在生成论文摘要