Unsupervised Clustering of Marine Vessel Trajectories in Historical AIS Database
2022 25TH INTERNATIONAL CONFERENCE ON INFORMATION FUSION (FUSION 2022)(2022)
摘要
This paper presents results on unsupervised clustering of marine vessel trajectories contained in a database of historical AIS messages. The historical AIS database is a collection of individual AIS messages from multiple vessels over a specific time period. Inherent in these messages are vessel trajectories that define a set of marine traffic patterns. Clustering these trajectories in an unsupervised manner help obtaining clustered datasets pertaining to unique maritime traffic lanes, which serve as an input to the situational awareness system of marine vessels. The clustering algorithm employed is non parametric and proceeds in three stages i) Trajectory extraction that pre-processes the AIS database to extract vessel trajectories, ii) Trajectory shrinking that uses Manifold-blurring Mean-Shift algorithm in combination with Principal Component Analysis applied locally to the dataset, in order to increase similarity between trajectories belonging to the same cluster, and iii) Trajectory Clustering that clusters the shrunk trajectories using the Longest Common Sub-sequence trajectory similarity measure and Agglomerative Hierarchical Clustering algorithm. The clustering algorithm exploits the inherent structure between the position and course over ground measurements contained within the AIS messages and its efficacy are demonstrated using historical AIS data collected over Trondheimsfjord. Further, the utility of the clustered dataset is demonstrated through a case-study of long term trajectory prediction of a target vessel.
更多查看译文
关键词
marine vessel trajectories
AI 理解论文
溯源树
样例
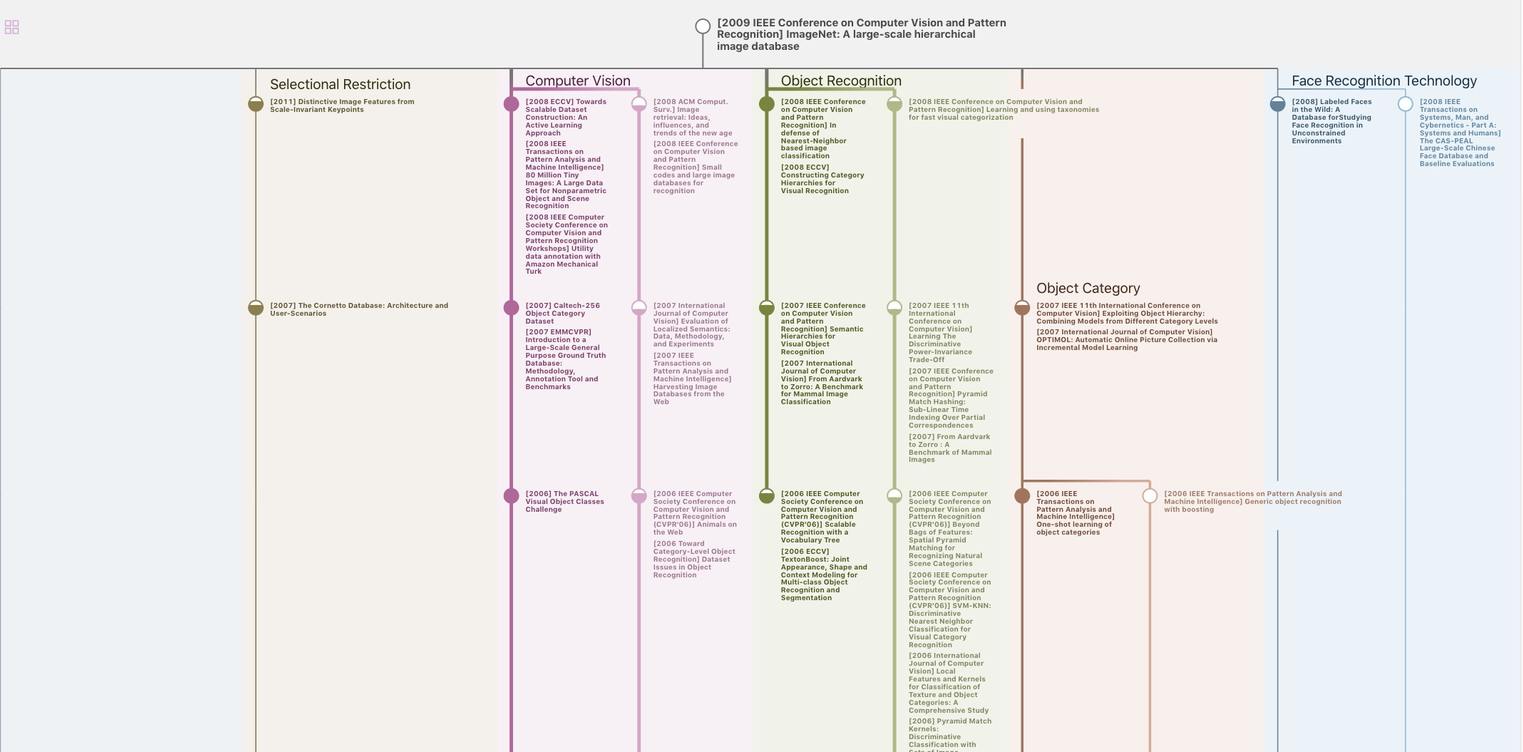
生成溯源树,研究论文发展脉络
Chat Paper
正在生成论文摘要