Novel Composite Feature Fusion for Diabetes Diagnosis Using Artificial Neural Network
2022 International Conference on Decision Aid Sciences and Applications (DASA)(2022)
摘要
Diabetes is a chronic disease that causes excess blood sugar and can harm vital organs if untreated. The conventional diagnosis methods require special laboratory equipment and medical experts. For alleviating these issues, the paper presents an early fusion of novel features using exploratory data analysis for automated diabetes diagnosis. Evaluations are performed using the Pima Indian Diabetes Diagnosis dataset. Firstly, inter-attribute correlation analysis identifies the redundancy in attribute pairs. Moderately correlated attribute pairs form the novel five continuous composite features by either multiplying or dividing depending on diagnosis computational aid and individual features values. Novel composite threshold-based linear separators are used to generate eleven binary features. Dataset attributes and composite features are fused with four earlier reported features calculated by principal component analysis to generate a feature vector. A cross-validated multi-layer perceptron neural network resulted in the best accuracy of 93.51%. Comparative analysis with several earlier reported diabetes diagnoses showed the proposed work’s superiority.
更多查看译文
关键词
Artificial neural network,diabetes diagnosis,early fusion,principal component analysis
AI 理解论文
溯源树
样例
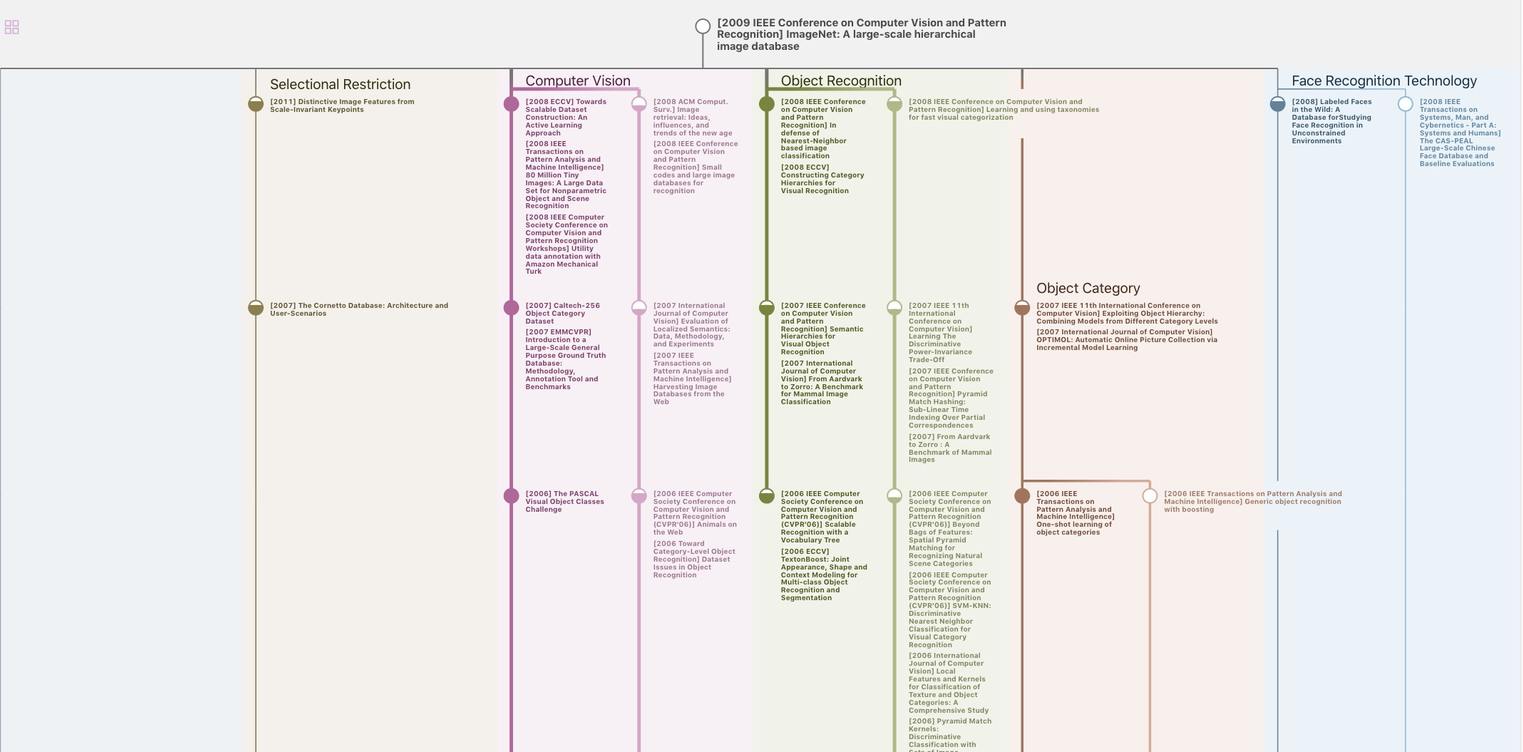
生成溯源树,研究论文发展脉络
Chat Paper
正在生成论文摘要