EVALUATING PIXEL-BASED AND OBJECT-BASED APPROACHES FOR FOREST ABOVE-GROUND BIOMASS ESTIMATION USING A COMBINATION OF OPTICAL, SAR, AND AN EXTREME GRADIENT BOOSTING MODEL
XXIV ISPRS CONGRESS: IMAGING TODAY, FORESEEING TOMORROW, COMMISSION III(2022)
Abstract
The above-ground biomass (AGB) estimation monitoring provides a powerful tool for the assessment of carbon emission and sequestration. Using remote sensing technique is an environmentally friendly way of biomass estimation. Thus, this paper investigated optical (i.e. Landsat 8 OLI and Sentinel-2), synthetic aperture radar (SAR) (global phased array type L-band SAR (PALSAR/PALSAR-2) and Sentinel-1), and their integration for AGB estimation of the Pack demonstration forest in Warrensburg, NY. Importantly, a LiDAR AGB raster of the study area was used as reference data for training/testing purposes. Then, an extreme gradient boosting (Xgboost) machine learning model was used to predict biomass values. The major goal of this study was to compare the performance of pixel-based and object-based image analysis (OBIA) for the AGB estimation. Results indicated that the object-based approach improved the RMSE of AGB prediction about 6.28 Mg/ha for optical + SAR, 6.17 Mg/ha for SAR, and 5.6 Mg/ha for optical data in comparison to the pixel-based approach. Moreover, the combination of optical and SAR data increased the prediction accuracy regardless of feature extraction approach.
MoreTranslated text
Key words
Extreme gradient boosting (Xgboost), forest biomass, Object-based image analysis (OBIA), Synthetic aperture radar (SAR), Machine learning, Airborne light detection and ranging (LiDAR)
AI Read Science
Must-Reading Tree
Example
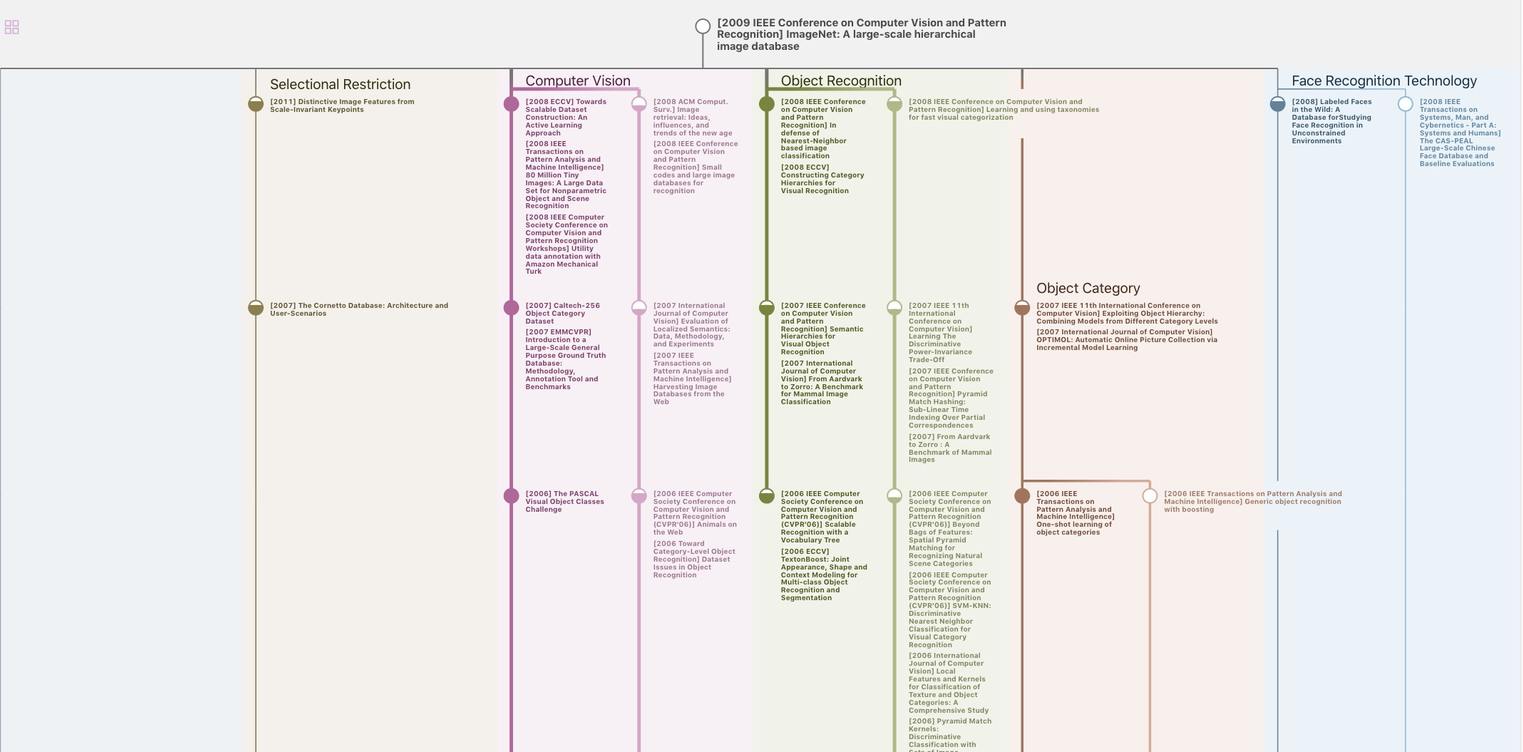
Generate MRT to find the research sequence of this paper
Chat Paper
Summary is being generated by the instructions you defined