Sentiment-aware multimodal pre-training for multimodal sentiment analysis
Knowledge-Based Systems(2022)
摘要
Pre-trained models, together with fine-tuning on downstream labeled datasets, have demonstrated great success in various tasks, including multimodal sentiment analysis. However, most most multimodal pre-trained models focus on learning general lexical and/or visual information, while ignoring sentiment signals. To address this problem, we propose a sentiment-aware multimodal pre-training (SMP) framework for multimodal sentiment analysis. In particular, we design a cross-modal contrastive learning module based on the interactions between visual and textual information, and introduce additional sentiment-aware pre-training objectives (e,g., fine-grained sentiment labeling) to capture fine-grained sentiment information from sentiment-rich datasets. We adopt two objectives (i.e., masked language modeling and masked auto-encoders) to capture semantic information from text and images. We conduct a series of experiments on sentence-level and target-oriented multimodal sentiment classification tasks, wherein the results of our SMP model exceeds the state-of-the-art results. Additionally, ablation studies and case studies are conducted to verify the effectiveness of our SMP model.
更多查看译文
关键词
Multimodal,Sentiment analysis,Pretraining
AI 理解论文
溯源树
样例
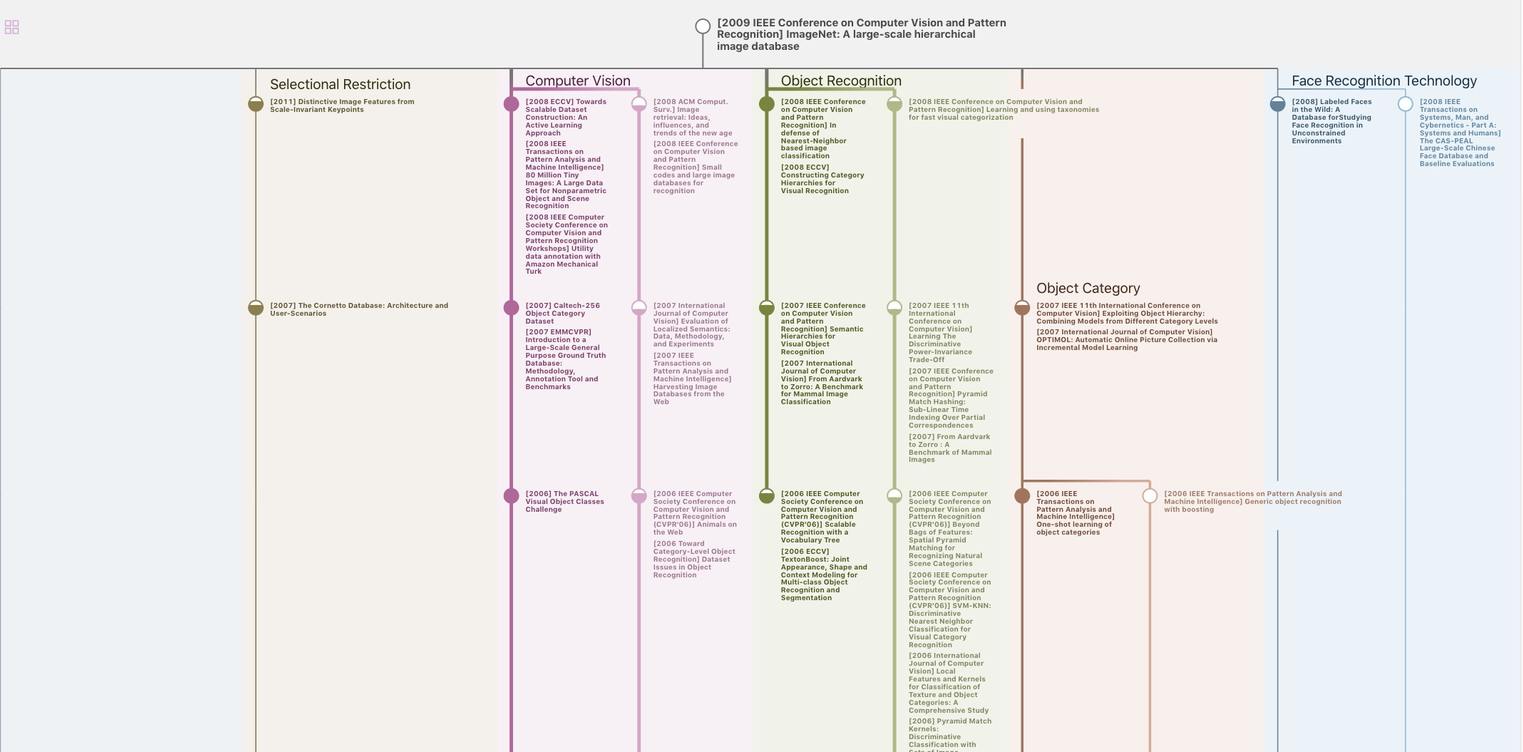
生成溯源树,研究论文发展脉络
Chat Paper
正在生成论文摘要