Stock Selection via Expand-excite Conv Attention Autoencoder and Layer Sparse Attention Transformer: A Classification Approach Inspire Time Series Sequence Recognition
IEEE International Joint Conference on Neural Network (IJCNN)(2022)
摘要
It is a very complicated task to quantify stock fluctuations and use them to generate profits. Despite the rapid progress in Deep Learning, the effect in predicting stock markets with high randomness and high noise is still not good. Therefore, how to filter out the noise in the stock market is very important for stock price prediction. In the past, we usually used feature engineering to denoise the original stock data. However, with the advent of Deep Learning, neural networks can now automatically perform feature engineering. The question of how to design a reasonable neural network for highly noisy data and perform effective signal extraction is therefore the problem we discuss in this paper. The main novelty of our work is that we transform the stock prediction problem into a classification problem in a time period. Through the layer-by-layer transformation, the model pays attention to different levels of detail each time to achieve automatic construction of different levels. The purpose of the feature is that the model can recognize more valuable information. On this basis, this article proposes the model TS-ECLST. TS-ECLST is the abbreviation of Time Series Expand-excite Cony Attention Autoencoder Layer Sparse Attention Transformer. Using experiments on two markets with six years of data, we show that the TS-ECLST model is better than the current mainstream model and even better than the latest graph neural model in terms of profitability. We also investigate the importance of the layer-transformer structure by ablation. The results show that this hierarchical attention structure is indeed better than the non-hierarchical structure and it is also better than various models based on LSTM or transformer.
更多查看译文
关键词
time-series,classification,autoencoder,convolutional,transformer,sparse attention
AI 理解论文
溯源树
样例
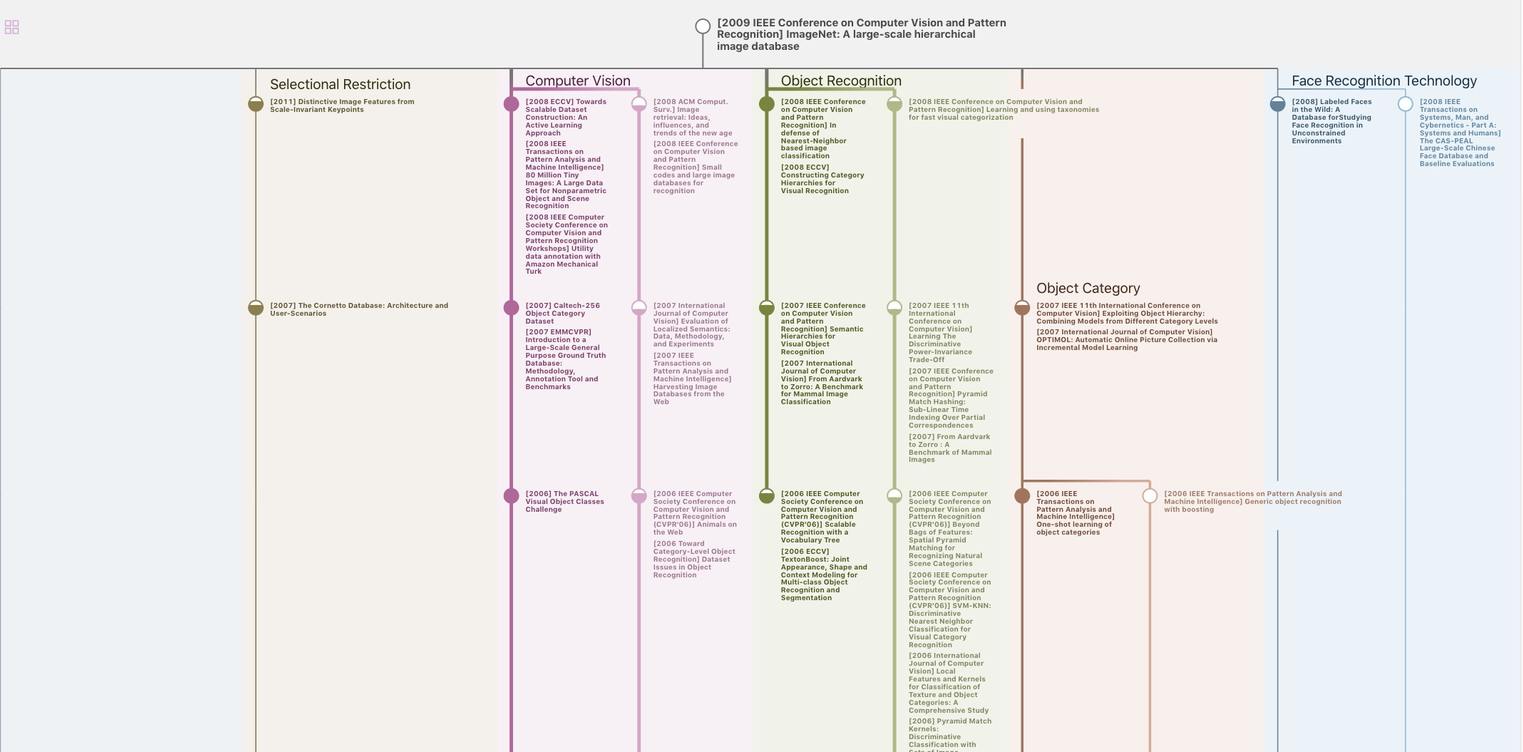
生成溯源树,研究论文发展脉络
Chat Paper
正在生成论文摘要