Algorithms for learning a mixture of linear classifiers
International Conference on Algorithmic Learning Theory (ALT)(2022)
摘要
Linear classifiers are a basic model in supervised learning. We study the problem of learning a mixture of linear classifiers over Gaussian marginals. Despite significant interest in this problem, including in the context of neural networks, basic questions like efficient learnability and identifiability of the model remained open. In this paper, we design algorithms for recovering the parameters of the mixture of k linear classifiers. We obtain two algorithms which both have polynomial dependence on the ambient dimension n, and incur an exponential dependence either on the number of the components k or a natural separation parameter ∆ > 0. These algorithmic results in particular settle the identifiability question under provably minimal assumptions.
更多查看译文
AI 理解论文
溯源树
样例
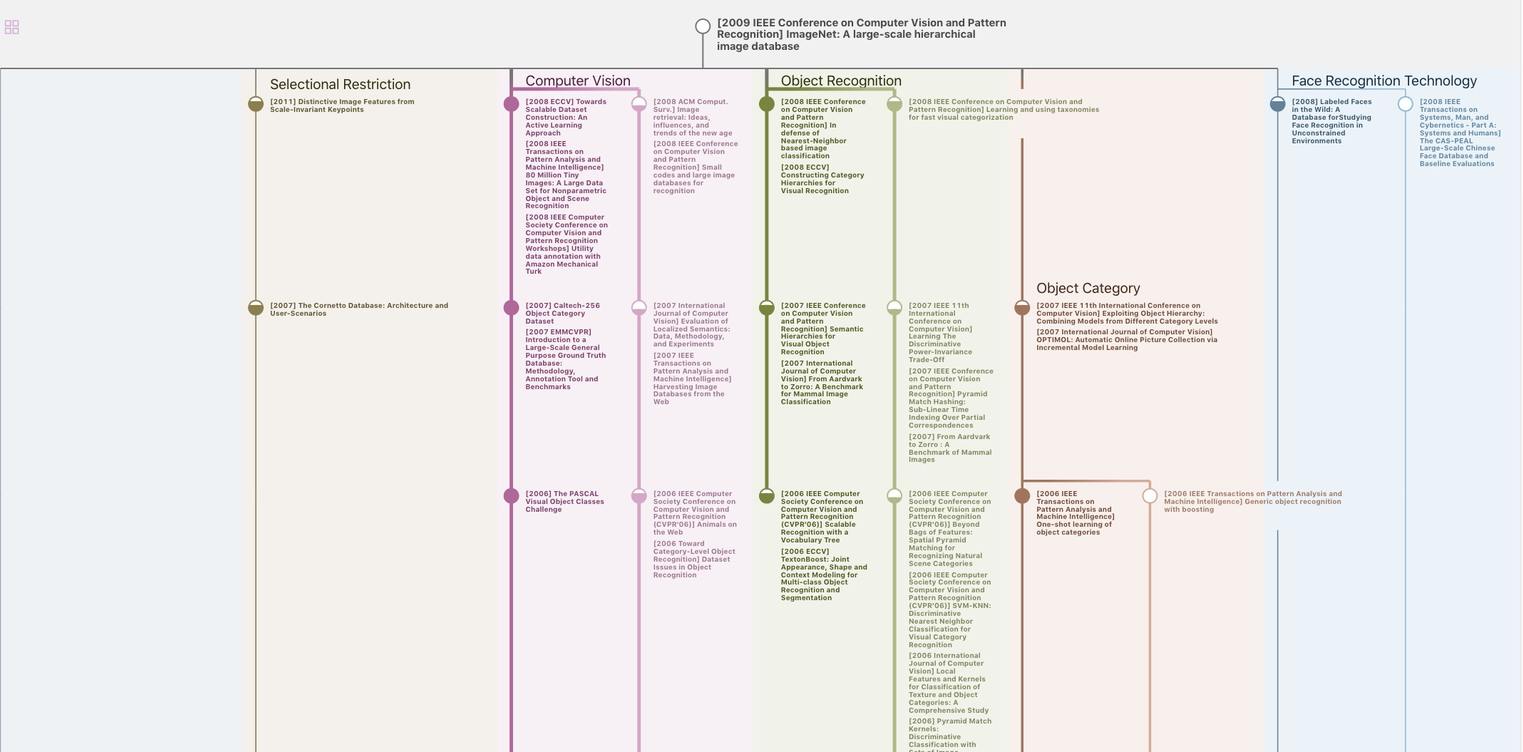
生成溯源树,研究论文发展脉络
Chat Paper
正在生成论文摘要