Noise suppression for improved few-shot learning
IEEE International Conference on Acoustics, Speech, and Signal Processing (ICASSP)(2022)
摘要
Few-shot learning (FSL) aims to generalize from few labeled samples. Recently, metric-based methods have achieved surprising classification performance on many FSL benchmarks. However, those methods ignore the impact of noise, making the few-shot learning still tricky. In this work, we identify that noise suppression is important to improve the performance of FSL algorithms. Hence, we proposed a novel attention-based contrastive learning model with discrete cosine transform input (ACL-DCT), which can suppress the noise in input images, image labels, and learned features, respectively. ACL-IX:T takes the transformed frequency domain representations by IX:T as input and removes the high-frequency part to suppress the input noise. Besides, an attention-based alignment of the feature maps and a supervised contrastive loss are used to mitigate the feature and label noise. We evaluate our ACL-DCT by comparing previous methods on two widely used datasets for few-shot classification (i.e., miniImageNet and CUB). The results indicate that our proposed method outperforms the state-of-the-art methods.
更多查看译文
关键词
Few-shot learning,image classification,noise suppression,contrastive learning
AI 理解论文
溯源树
样例
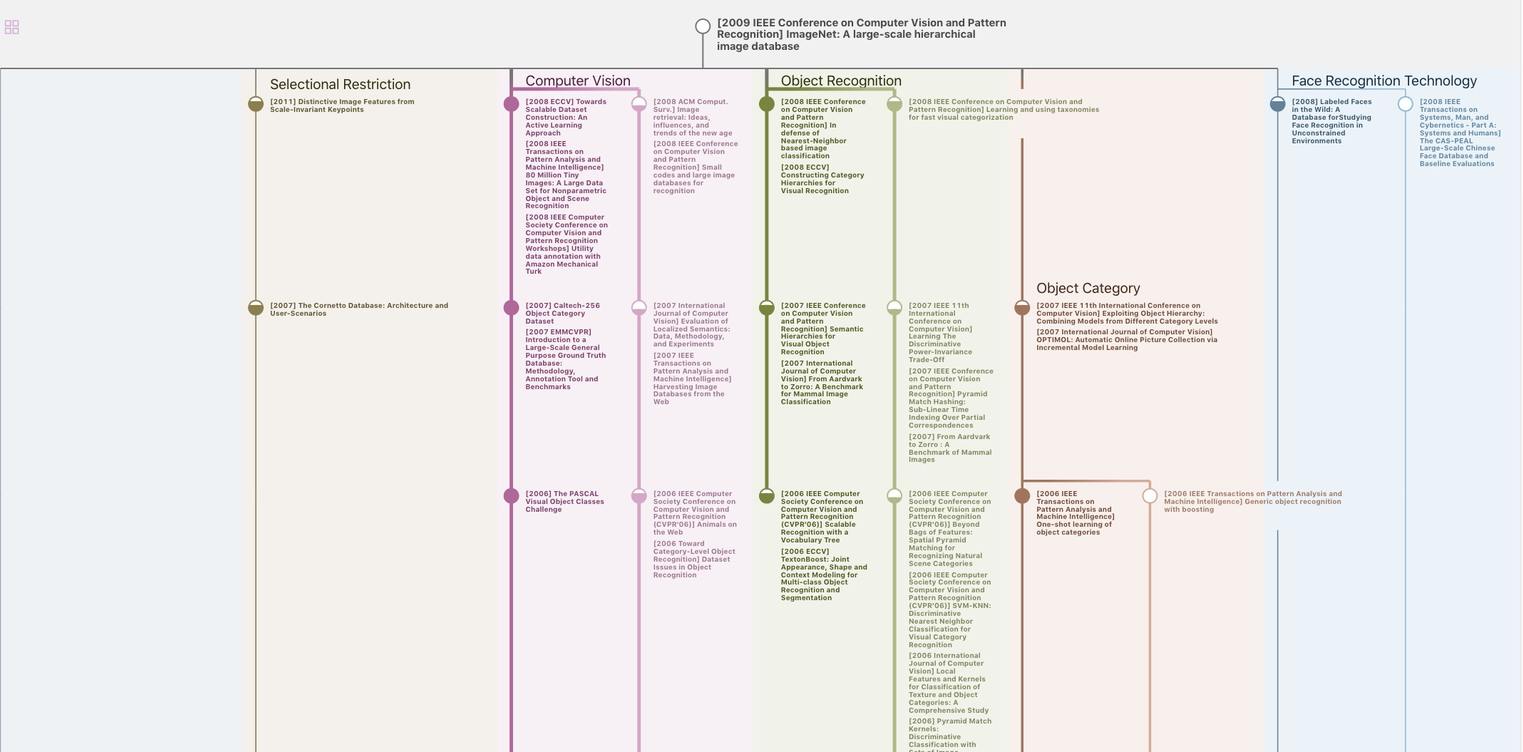
生成溯源树,研究论文发展脉络
Chat Paper
正在生成论文摘要