Poster Abstract: Adapting Pretrained Features for Efficient Unsupervised Acoustic Anomaly Detection
2022 21st ACM/IEEE International Conference on Information Processing in Sensor Networks (IPSN)(2022)
摘要
Faults in industrial equipment lead to significant costs due to down-time and unplanned maintenance interventions. Acoustic Internet of Things (IoT) sensors combined with machine learning offers the possibility of early fault detection to mitigate these costs. How-ever, prior approaches to Acoustic Anomaly Detection (AAD) are poorly suited to operating within the resource constraints of IoT systems as they require the acquisition of large volumes of data to train models from scratch for different machine types or oper-ating environments. To overcome the limitations, we introduce a system utilizing pretrained low-dimensional features and Gaussian mixture models. Preliminary results on real-world datasets show that our proposed approach outperforms state-of-the-art solutions based on the area under the curve (AUC) score with an average of 4 different machine types. Furthermore, our approach requires far less training data, making it more suitable to operate within the power and network constraints of IoT devices.
更多查看译文
关键词
adapting pretrained features,efficient unsupervised Acoustic Anomaly Detection,industrial equipment lead,significant costs,unplanned maintenance interventions,Acoustic Internet,Things sensors,machine learning,early fault detection,prior approaches,resource constraints,IoT systems,oper-ating environments,system utilizing,low-dimensional features,Gaussian mixture models,4 different machine types,training data,IoT devices
AI 理解论文
溯源树
样例
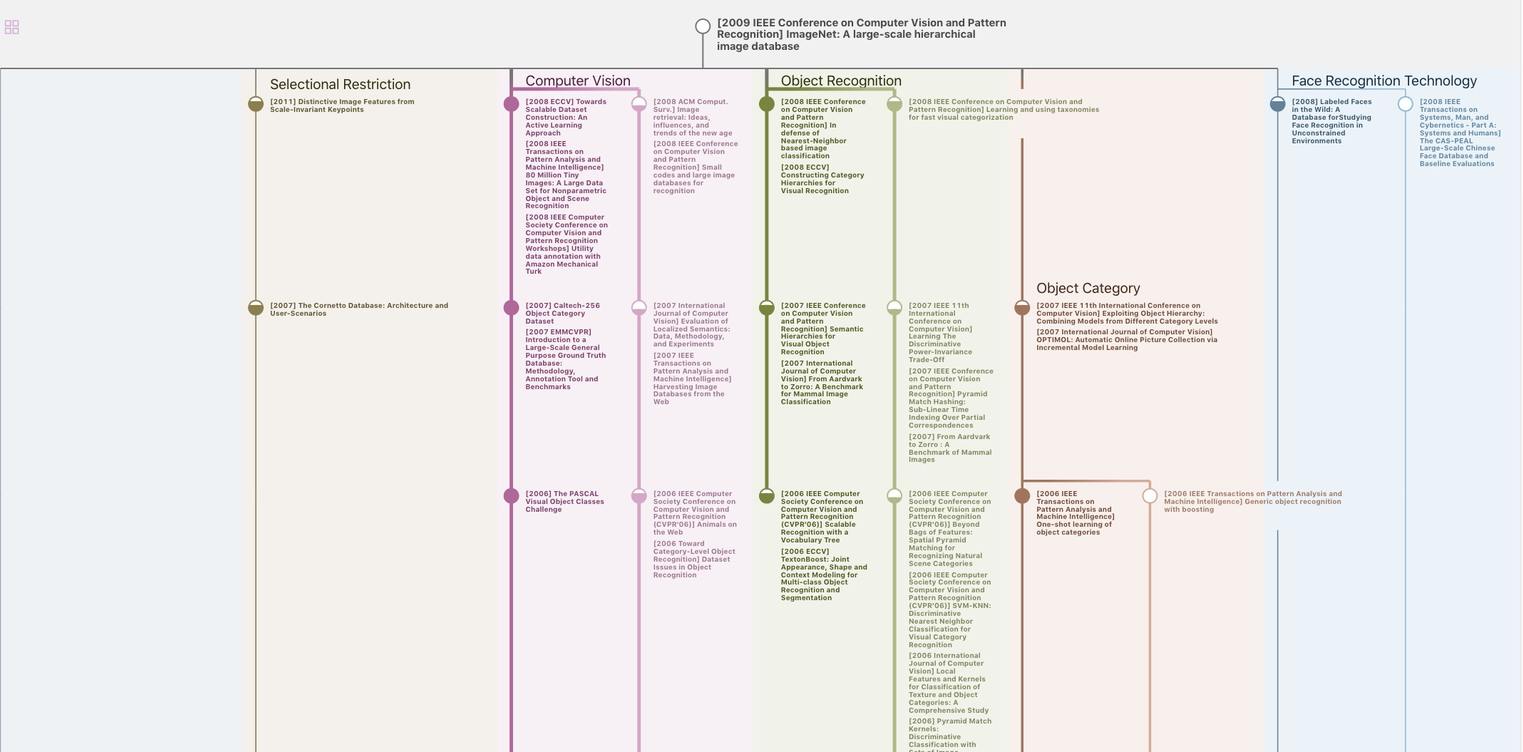
生成溯源树,研究论文发展脉络
Chat Paper
正在生成论文摘要