Interactive Video Corpus Moment Retrieval Using Reinforcement Learning
PROCEEDINGS OF THE 30TH ACM INTERNATIONAL CONFERENCE ON MULTIMEDIA, MM 2022(2022)
关键词
Interactive search,video corpus moment retrieval,reinforcement learning,user simulation
AI 理解论文
溯源树
样例
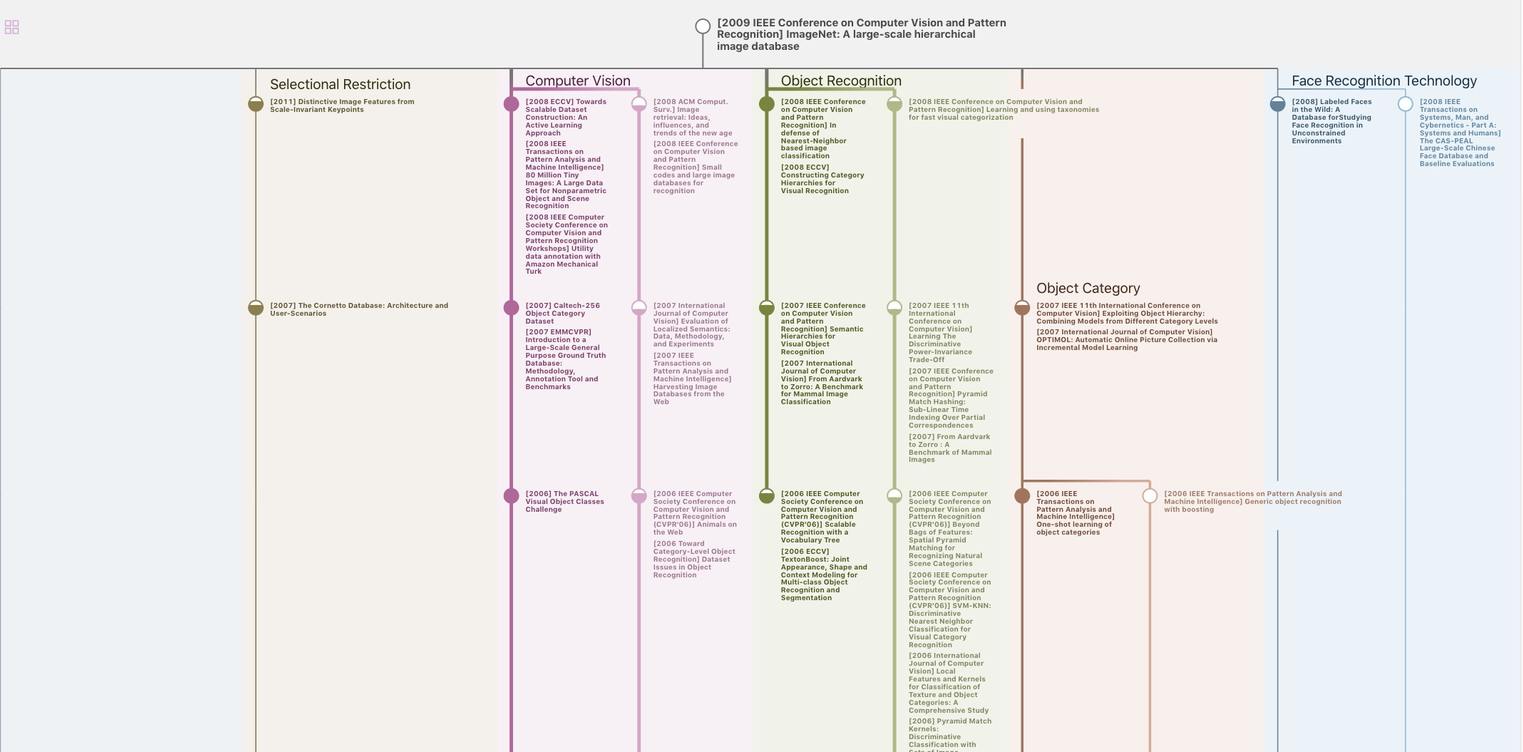
生成溯源树,研究论文发展脉络
Chat Paper
正在生成论文摘要