Towards Neural Network Interpretability Using Commonsense Knowledge Graphs.
IEEE International Semantic Web Conference(2022)
摘要
Convolutional neural networks (CNNs) classify images by learning intermediate representations of the input throughout many layers. In recent work, latent representations of CNNs have been aligned with semantic concepts. However, for generating such alignments, the majority of existing methods predominantly rely on large amounts of labeled data, which is hard to acquire in practice. In this work, we address this limitation by presenting a framework for mapping hidden units from CNNs to semantic attributes of classes extracted from external commonsense knowledge repositories. We empirically demonstrate the effectiveness of our framework on copy-paste adversarial image classification and generalized zero-shot learning tasks.
更多查看译文
关键词
Interpretability,Image classification,Knowledge graphs
AI 理解论文
溯源树
样例
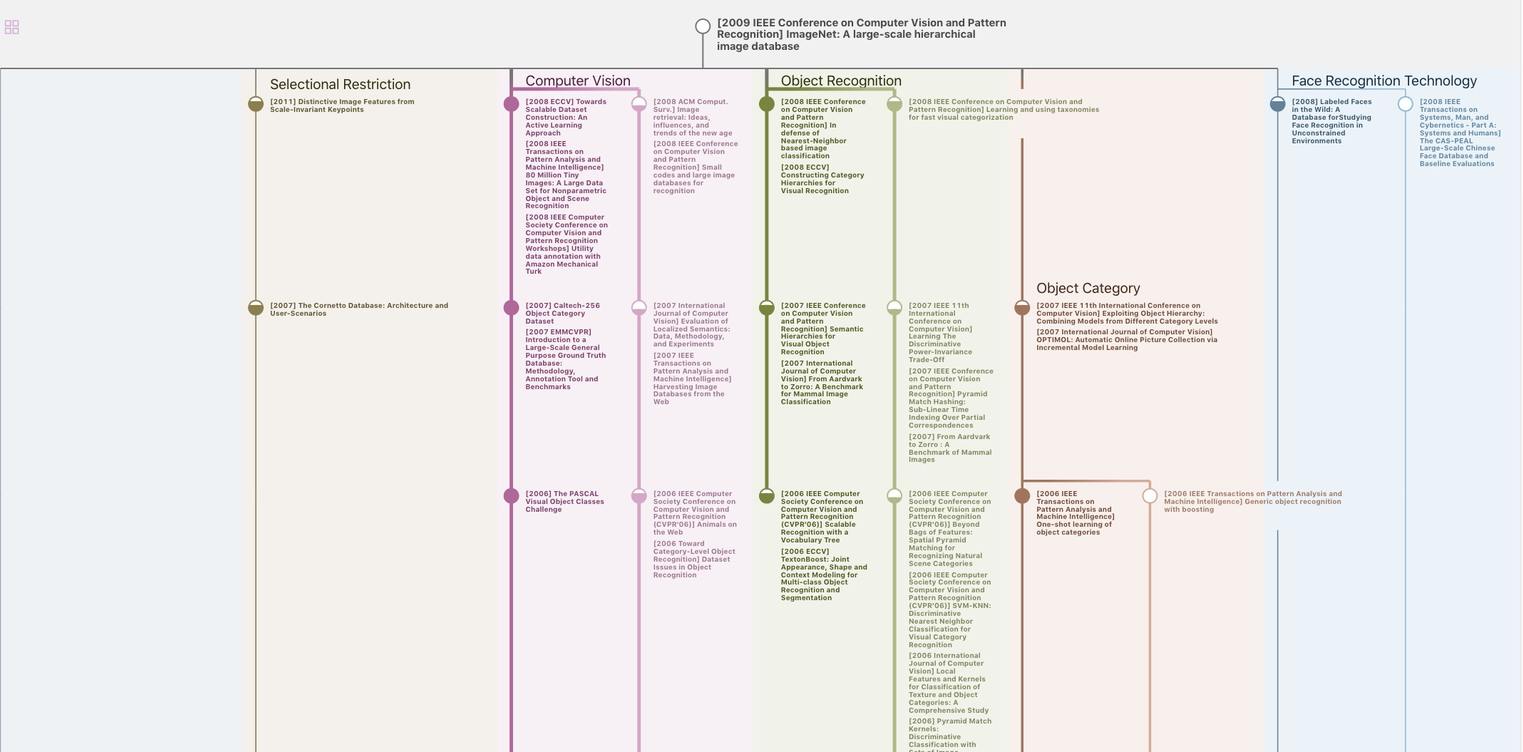
生成溯源树,研究论文发展脉络
Chat Paper
正在生成论文摘要