Reduction of artifacts and noise in small electrogram datasets without manual annotation using transfer machine learning
European Heart Journal(2022)
摘要
Abstract Background/Introduction Mapping AF is challenging. Monophasic action potentials (MAPs) show that most of the recorded signals are not representing the atrial activity, and arise from far-field or other artifacts. Thus, reducing noise in electrophysiological signals is essential, yet it can be difficult for cross-talk from other chambers and pacing. Strategies to reduce noise include template matching, averaging, and smoothing, but all of them have major limitations. Furthermore, expert interpretation requires knowledge to discriminate signals from noise, but is subjective. Purpose We hypothesised a) that atrial and ventricular electrograms with varying artifact and noise can be denoised using autoencoder neural networks (NNs) without requiring manual annotation and in a reproducible manner, and b) we could train these NNs on a large available dataset ventricular signals, then apply transfer learning to the original smaller atrial dataset. We applied this approach to MAPs, which have interpretable shapes and would help identifying local from far-field signals helping in diagnosis, mapping and ablation. Methods We first trained with 5706 left and right ventricular MAPs from 42 patients with ischemic cardiomyopathy (age 65±13y; Fig. 1A), with 60% for training, 20% (validation) and 20% (testing). Transfer learning and parameter-tuning were then used to apply this NN to a smaller sample of atrial MAPs (N=641 from 21 patients, 67±5y, 13 women; Fig. 2B, D, F). The autoencoder was used to eliminate pacing artifacts in ventricular MAPs (Fig. 1B, C) and denoise atrial MAPs (Fig. 2C, E, G) by reconstructing key learned features. The accuracy of the reconstruction was evaluated using Pearson Correlation Coefficient (PCC) and a novel similarity coefficient (SC). No manual annotation was required to identify noisy signals. Results The trained NN encoder learned key features of ventricular MAPs and reconstructed these clean signals with a SC=0.91±0.16 and PCC=0.99±0.01 (Fig. 1A). With this training, the NN was able to denoise ventricular MAPs with pacing artifact (Fig. 1B, C). After fine-tuning, the NN learned key signal features (upstroke, triangular descent, terminus) and thus reduced diverse noise without specific training or manual annotation. Namely, it was able to reconstruct atrial MAPs eliminating ventricular noise, high frequency noise and truncated signals (Fig. 2). Conclusions Machine learned encoder-decoders are powerful tools that can learn essential features of atrial and ventricular signals and hence isolate noise. Transfer learning is effective when large datasets are unavailable for training. This approach can separate atrial beats from far-field ventricular beats and other sources of noise. The ability to eliminate a diverse range of noise improves this approach over existing techniques and may have far-reaching applications in electrophysiology, such as mapping and ablation. Funding Acknowledgement Type of funding sources: Public grant(s) – National budget only. Main funding source(s): NIH
更多查看译文
AI 理解论文
溯源树
样例
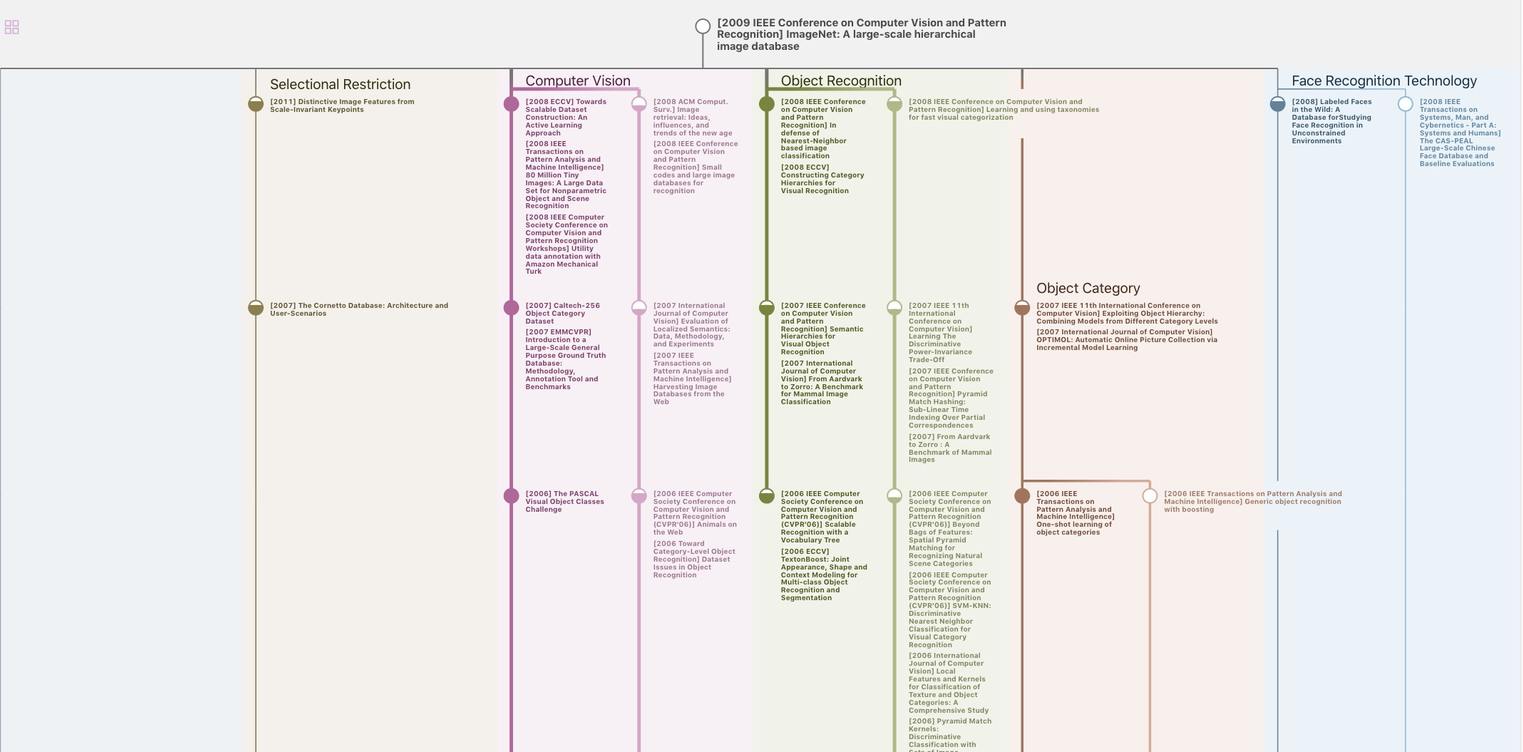
生成溯源树,研究论文发展脉络
Chat Paper
正在生成论文摘要