Experimental Design for Multi-Channel Imaging Via Task-Driven Feature Selection
ICLR(2024)
摘要
This paper presents a data-driven, task-specific paradigm for experimentaldesign, to shorten acquisition time, reduce costs, and accelerate thedeployment of imaging devices. Current approaches in experimental design focuson model-parameter estimation and require specification of a particular model,whereas in imaging, other tasks may drive the design. Furthermore, suchapproaches often lead to intractable optimization problems in real-worldimaging applications. Here we present a new paradigm for experimental designthat simultaneously optimizes the design (set of image channels) and trains amachine-learning model to execute a user-specified image-analysis task. Theapproach obtains data densely-sampled over the measurement space (many imagechannels) for a small number of acquisitions, then identifies a subset ofchannels of prespecified size that best supports the task. We propose a method:TADRED for TAsk-DRiven Experimental Design in imaging, to identify the mostinformative channel-subset whilst simultaneously training a network to executethe task given the subset. Experiments demonstrate the potential of TADRED indiverse imaging applications: several clinically-relevant tasks in magneticresonance imaging; and remote sensing and physiological applications ofhyperspectral imaging. Results show substantial improvement over classicalexperimental design, two recent application-specific methods within the newparadigm, and state-of-the-art approaches in supervised feature selection. Weanticipate further applications of our approach. Code is available:https://github.com/sbb-gh/experimental-design-multichannel
更多查看译文
关键词
Experimental Design,Supervised Feature Selection,Multi-Channel Imaging,Hyperspectral Imaging,Magnetic Resonance Imaging (MRI),Task-based Image Channel Selection
AI 理解论文
溯源树
样例
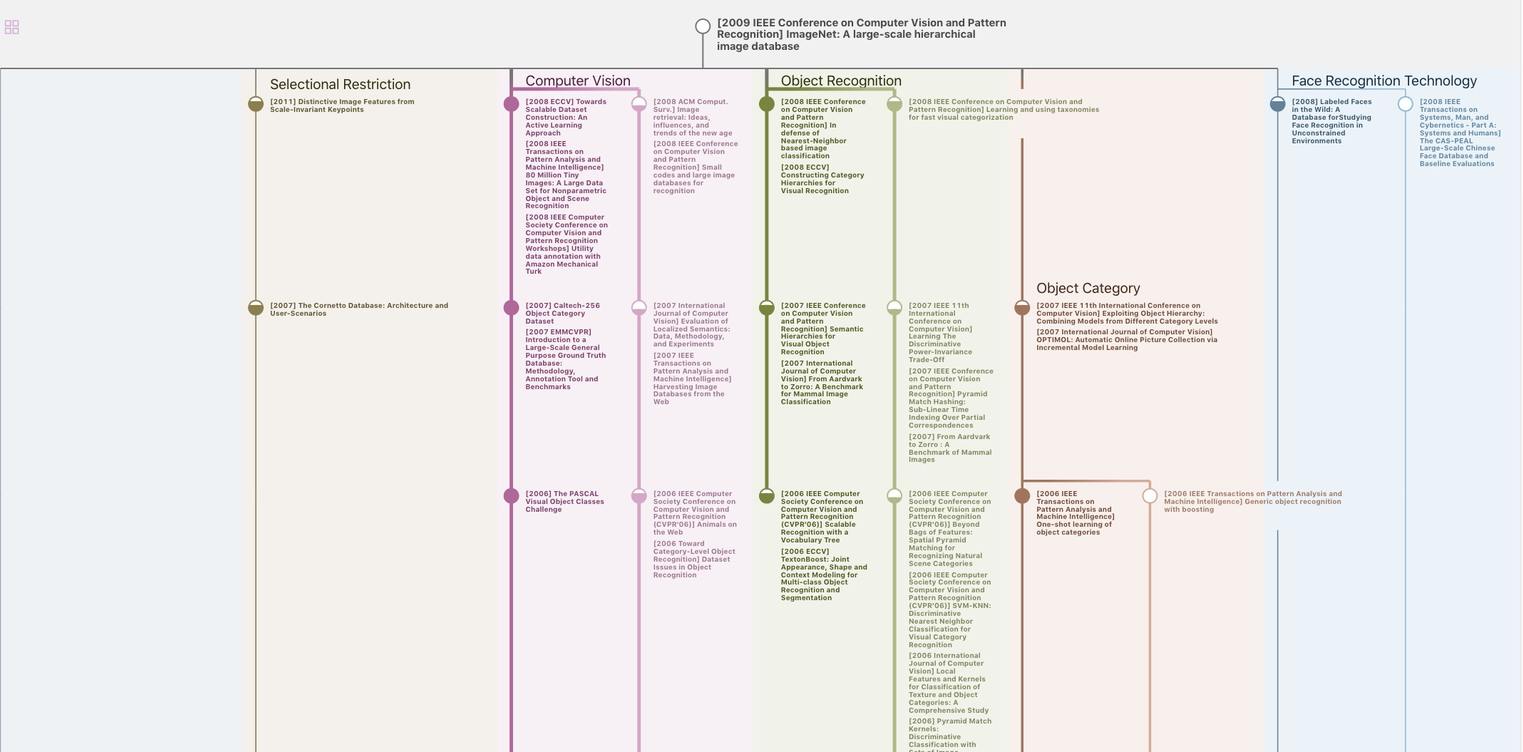
生成溯源树,研究论文发展脉络
Chat Paper
正在生成论文摘要