AV-casNet: Fully Automatic Arteriole-Venule Segmentation and Differentiation in OCT Angiography.
IEEE transactions on medical imaging(2023)
摘要
Automatic segmentation and differentiation of retinal arteriole and venule (AV), defined as small blood vessels directly before and after the capillary plexus, are of great importance for the diagnosis of various eye diseases and systemic diseases, such as diabetic retinopathy, hypertension, and cardiovascular diseases. Optical coherence tomography angiography (OCTA) is a recent imaging modality that provides capillary-level blood flow information. However, OCTA does not have the colorimetric and geometric differences between AV as the fundus photography does. Various methods have been proposed to differentiate AV in OCTA, which typically needs the guidance of other imaging modalities. In this study, we propose a cascaded neural network to automatically segment and differentiate AV solely based on OCTA. A convolutional neural network (CNN) module is first applied to generate an initial segmentation, followed by a graph neural network (GNN) to improve the connectivity of the initial segmentation. Various CNN and GNN architectures are employed and compared. The proposed method is evaluated on multi-center clinical datasets, including ${3} \times {3}$ mm2 and ${6} \times {6}$ mm2 OCTA. The proposed method holds the potential to enrich OCTA image information for the diagnosis of various diseases.
更多查看译文
关键词
Image segmentation,Retina,Deep learning,Convolutional neural networks,Classification algorithms,Topology,Photography,Vessel segmentation,optical coherence tomography angiography,arteriole-venule differentiation,graph neural network
AI 理解论文
溯源树
样例
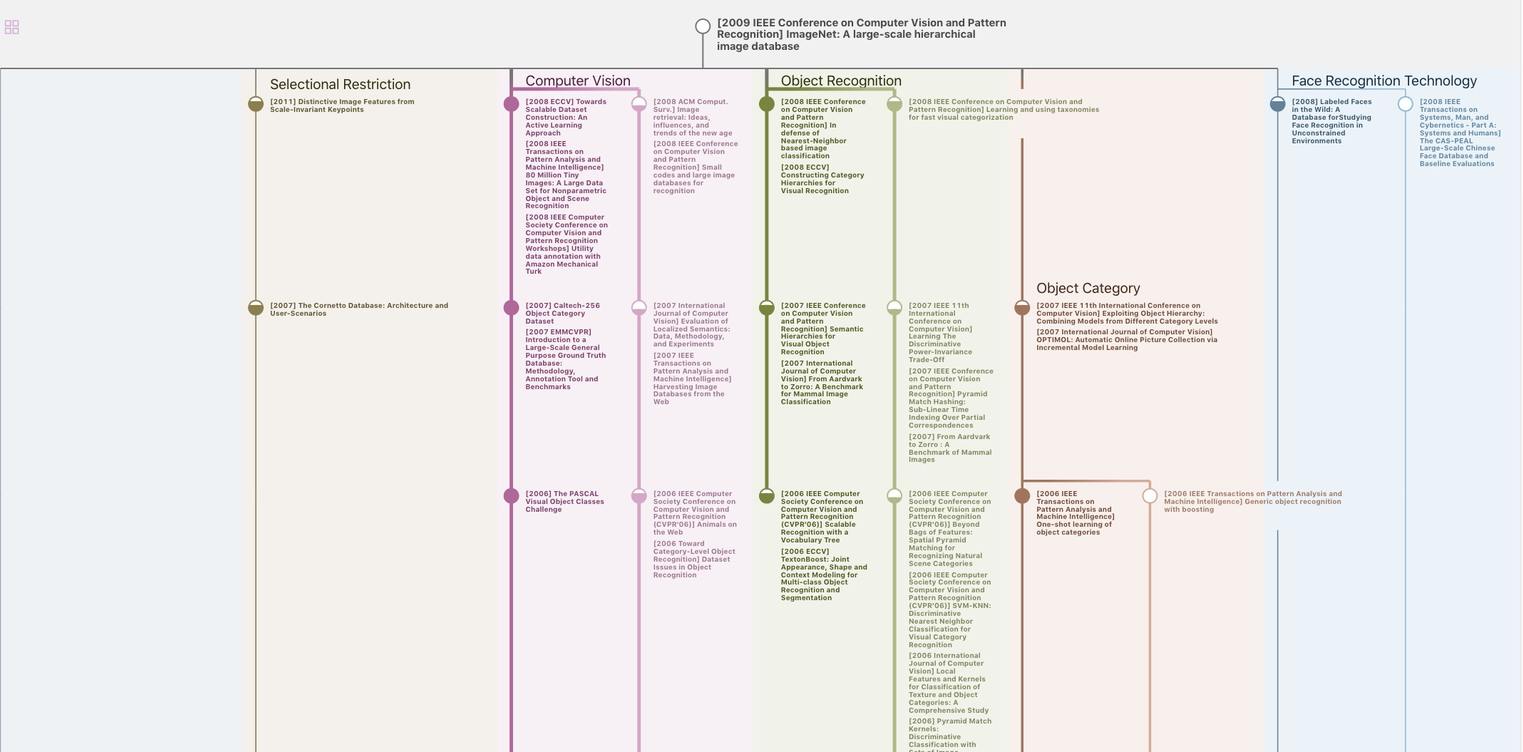
生成溯源树,研究论文发展脉络
Chat Paper
正在生成论文摘要