Estimating forces from cross-sectional data in the wake of flows past a plate using theoretical and data-driven models
Physics of Fluids(2022)
摘要
We report a comparative study of theoretical and data-driven models for estimating forces from velocity data in the wake of three-dimensional flows past a plate. The datasets with a range of angles of attack are calculated using the immersed boundary method. First, we develop a theoretical model to estimate forces on a flat plate from cross-sectional velocity data in the far wake. This algebraic model incorporates the local momentum deficit and pressure variation. Second, we develop several data-driven models based on the convolutional neural network (CNN) for force estimation by regarding the velocity field on a series of cross sections as images. In particular, we design three CNN architectures for integrating physical information or attention mechanism, and use different training datasets for interpolation and extrapolation tasks. The model performances indicate that the optimized CNN can identify important flow regions and learn empirical physical laws. The theoretical and CNN models are assessed by multiple criteria. In general, both models are accurate (with errors less than 10%), robust, and applicable to complex wake flows. The theoretical model is superior to the CNN model in terms of the completeness, cost, and interpretability, and the CNN model with the appropriate training data and optimized CNN architecture has better description and accuracy.
更多查看译文
关键词
flows,forces,plate,wake,cross-sectional,data-driven
AI 理解论文
溯源树
样例
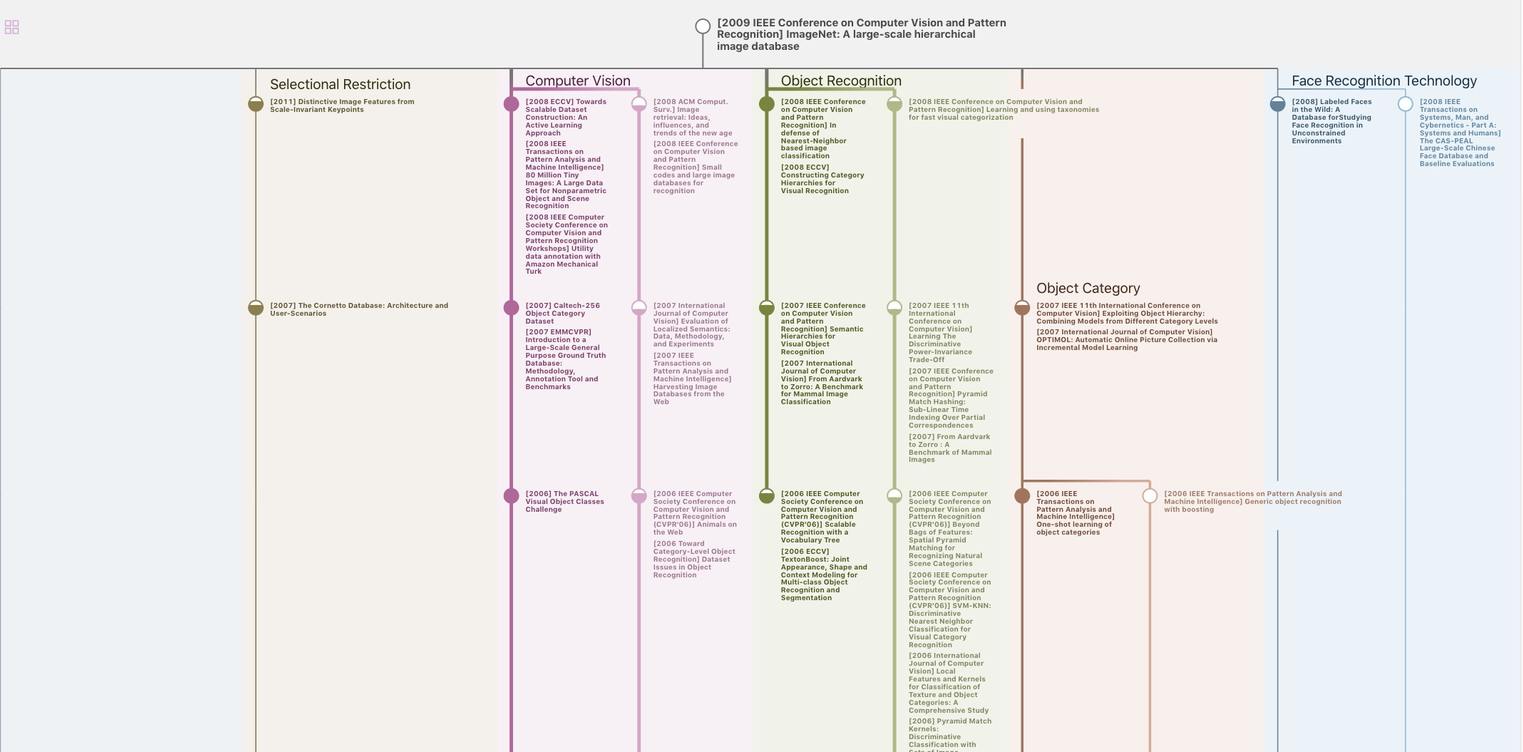
生成溯源树,研究论文发展脉络
Chat Paper
正在生成论文摘要