Exploring Class-Agnostic Pixels for Scribble-Supervised High-Resolution Salient Object Detection.
Neural computing & applications(2022)
摘要
Successful salient object detection is largely dependent on large-scale fine-grained annotated datasets. However, pixel-level annotation is a laborious process compared with weak labels and scant research has been done on high-resolution images. To mitigate these drawbacks, we propose a distinctive network to explore salient object in high-resolution images under scribble-supervised and relabel a previous high-resolution dataset with scribbles, namely Scr-HRSOD, in which each image is labelled in a few seconds. Since scribble labels lack structural information about objects, a boundary structure maintenance branch with shallow layers is introduced to capture low-level spatial details. Within the constraint of boundary branches, a lightweight contextual semantic branch process compressed inputs to obtain high-level semantic context and iteratively propagates the partially annotated pixels to surrounding similar regions, which are then employed as pseudo-labels to supervise the network. Extensive evaluations on five datasets illustrate the effectiveness of our introduced method. On HRSOD datasets, we achieve higher 0.861 F max and 0.887 S m values, which outperforms the existing foremost weakly supervised methods and even the fully supervised methods.
更多查看译文
关键词
High-resolution,Salient object detection,Scribble-supervised,Boundary structure,Semantic context,Lightweight
AI 理解论文
溯源树
样例
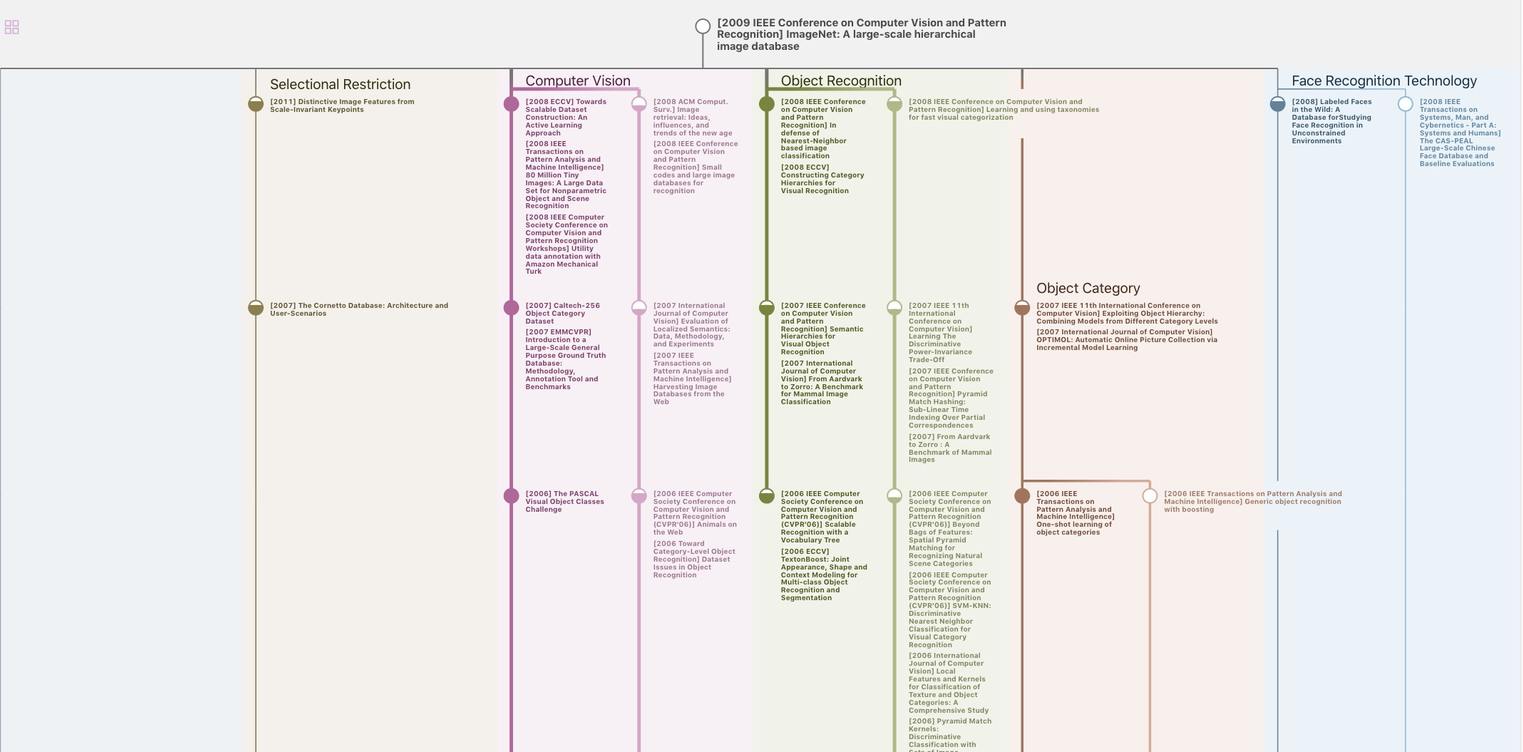
生成溯源树,研究论文发展脉络
Chat Paper
正在生成论文摘要