Deep Learning Based Online Metallic Surface Defect Detection Method for Wire and Arc Additive Manufacturing.
Robotics and computer-integrated manufacturing(2023)
摘要
Wire and arc additive manufacturing (WAAM) is an emerging manufacturing technology that is widely used in different manufacturing industries. To achieve fully automated production, WAAM requires a dependable, efficient, and automatic defect detection system. Although machine learning is dominant in the object detection domain, classic algorithms have defect detection difficulty in WAAM due to complex defect types and noisy detection environments. This paper presents a deep learning-based novel automatic defect detection solution, you only look once (YOLO)-attention, based on YOLOv4, which achieves both fast and accurate defect detection for WAAM. YOLO-attention makes improvements on three existing object detection models: the channel-wise attention mechanism, multiple spatial pyramid pooling, and exponential moving average. The evaluation on the WAAM defect dataset shows that our model obtains a 94.5 mean average precision (mAP) with at least 42 frames per second. This method has been applied to additive manufacturing of single-pass, multi-pass deposition and parts. It demonstrates its feasibility in practical industrial applications and has potential as a vision-based methodology that can be implemented in real-time defect detection systems.
更多查看译文
关键词
Wire and arc additive manufacturing,Defect detection,Online,Deep learning
AI 理解论文
溯源树
样例
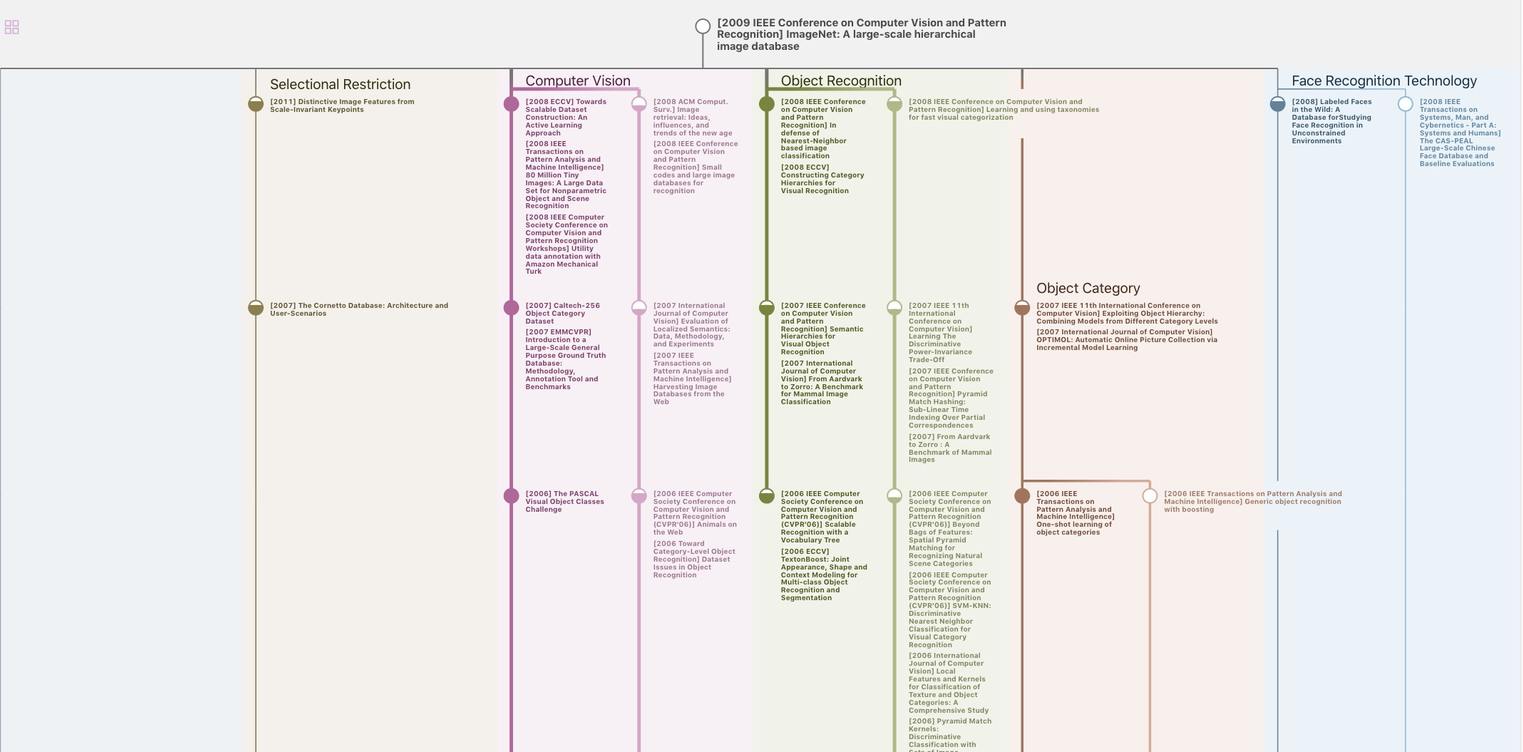
生成溯源树,研究论文发展脉络
Chat Paper
正在生成论文摘要