A Parallel Multi-Scale Time-Frequency Block Convolutional Neural Network Based on Channel Attention Module for Motor Imagery Classification.
Biomedical signal processing and control(2023)
摘要
The motor imagery brain-computer interface (MI-BCI) based on electroencephalography (EEG) enables direct communication between the human brain and external devices. In this paper, the MTFB-CNN, a parallel multiscale time-frequency block convolutional neural network based on the channel attention module, is proposed for EEG signals decoding, which can adaptively extract the time, frequency, and time-frequency domain features through parallel multi-scale time-frequency blocks, and then fuses and filters the features through attention mechanism and residual module. Experimental results based on the BCI Competition IV 2a and 2b datasets and the high gamma dataset show that the model achieves the highest average accuracy and kappa compared with existing baseline models. The MTFB-CNN is a novel and effective end-to-end model for decoding EEG signals without complex signals pre-processing operations, which has multi-scale feature extraction capability, making it successful in MI-BCI applications.
更多查看译文
关键词
Brain -computer interface,Motor imagery,Deep learning,Convolutional Neural Networks,Attention mechanism
AI 理解论文
溯源树
样例
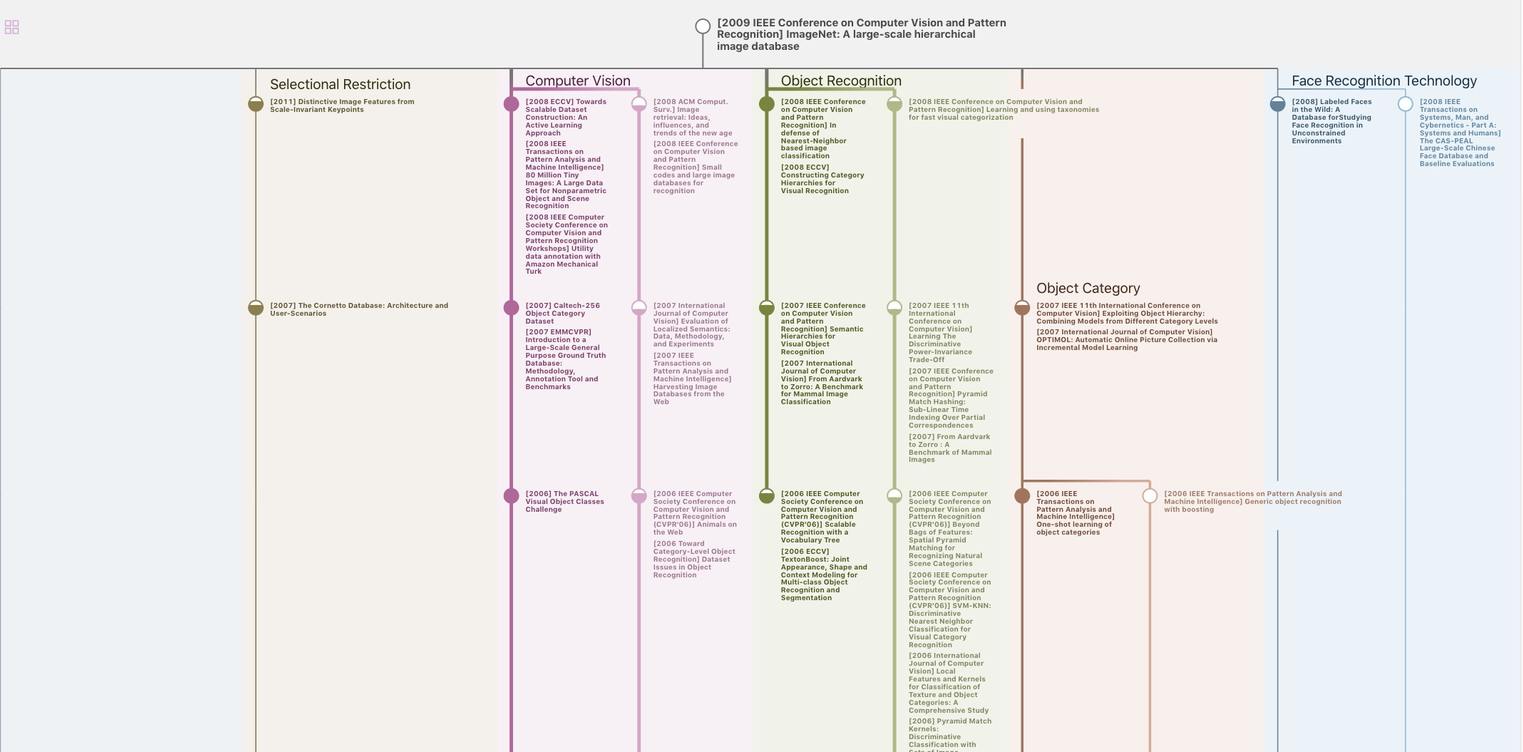
生成溯源树,研究论文发展脉络
Chat Paper
正在生成论文摘要