Self-Supervised Feature Extraction Via Time-Frequency Contrast for Intelligent Fault Diagnosis of Rotating Machinery
Social Science Research Network(2022)
摘要
Data-driven intelligent fault diagnosis requires a large amount of data. However, collecting sufficient labeled data from the field is generally difficult because mechanical devices are not allowed to operate under fault conditions for long periods of time. In addition, the working conditions of rotating machinery are often subject to change, which makes it hard for fault diagnosis. In this paper, a self-supervised Contrastive Learning model combining Frequency-domain and Time-domain information (CLFT) is proposed for pre-training. This model fully considers the time–frequency characteristics of vibration signals and reduces the dependence on the training data. Based on CLFT, a two-stage fault diagnosis pipeline applicable to the rotating machinery is constructed, which uses pre-training and fine-tuning strategies to accomplish specific diagnostic tasks. Experiments indicate that CLFT can effectively extract generalization features from unlabeled vibration signals, which makes the fault diagnosis method perform well under the condition of few samples and variable working conditions.
更多查看译文
关键词
Intelligent fault diagnosis,Contrastive learning,Time-frequency contrast,Self-supervised pre-training,Fourier transform
AI 理解论文
溯源树
样例
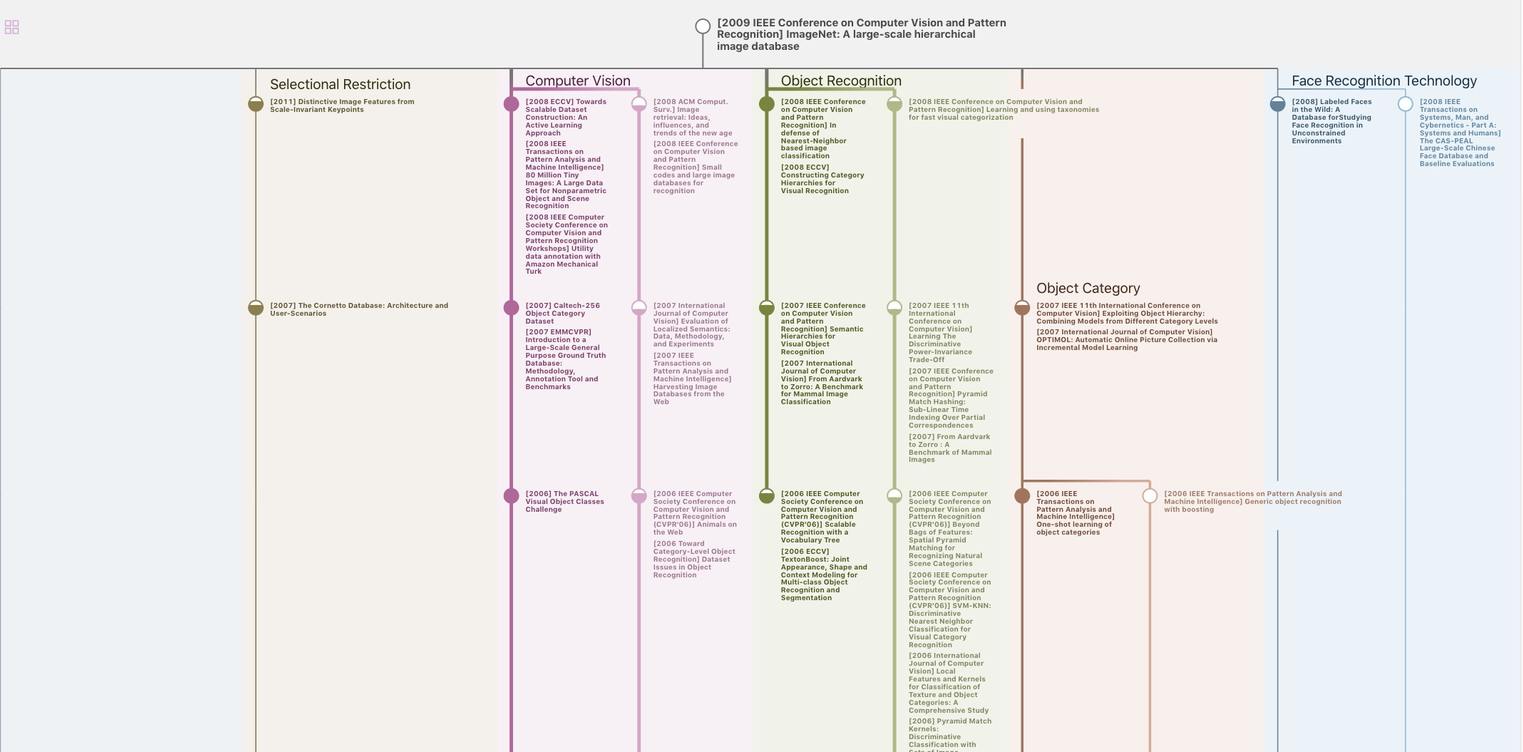
生成溯源树,研究论文发展脉络
Chat Paper
正在生成论文摘要