Self-supervised cycle-consistent learning for scale-arbitrary real-world single image super-resolution
Expert Systems with Applications(2023)
摘要
Whether conventional machine learning-based or current deep neural networks-based single image super -resolution (SISR) methods, they are generally trained and validated on synthetic datasets, in which low -resolution (LR) inputs are artificially produced by degrading high-resolution (HR) images based on a hand-crafted degradation model (e.g. , bicubic downsampling). One of the main reasons for this is that it is challenging to build a realistic dataset composed of real-world LR-HR image pairs. However, a domain gap exists between synthetic and real-world data because the degradations in real scenarios are more complicated, limiting the performance in practical applications of SISR models trained with synthetic data. To address these problems, we propose a Self-supervised Cycle-consistent Learning-based Scale-Arbitrary Super-Resolution framework (SCL-SASR) for real-world images. Inspired by the Maximum a Posteriori estimation, our SCL-SASR consists of a Scale-Arbitrary Super-Resolution Network (SASRN) and an inverse Scale-Arbitrary Resolution -Degradation Network (SARDN). SARDN and SASRN restrain each other with the bidirectional cycle consistency constraints as well as image priors, making SASRN adapt to the image-specific degradation well. Meanwhile, considering the lack of targeted training images and the complexity of realistic degradations, SCL-SASR is designed to be online optimized solely with the LR input prior to the SR reconstruction. Benefitting from the flexible architecture and the self-supervised learning manner, SCL-SASR can easily super-resolve new images with arbitrary integer or non-integer scaling factors. Experiments on real-world images demonstrate the high flexibility and good applicability of SCL-SASR, which achieves better reconstruction performance than state-of-the-art self-supervised learning-based SISR methods as well as several external dataset-trained SISR models.
更多查看译文
关键词
Real-world image,Super-resolution,Resolution-degradation,Self-supervised cycle-consistent learning,Arbitrary scaling factors,Convolutional neural networks
AI 理解论文
溯源树
样例
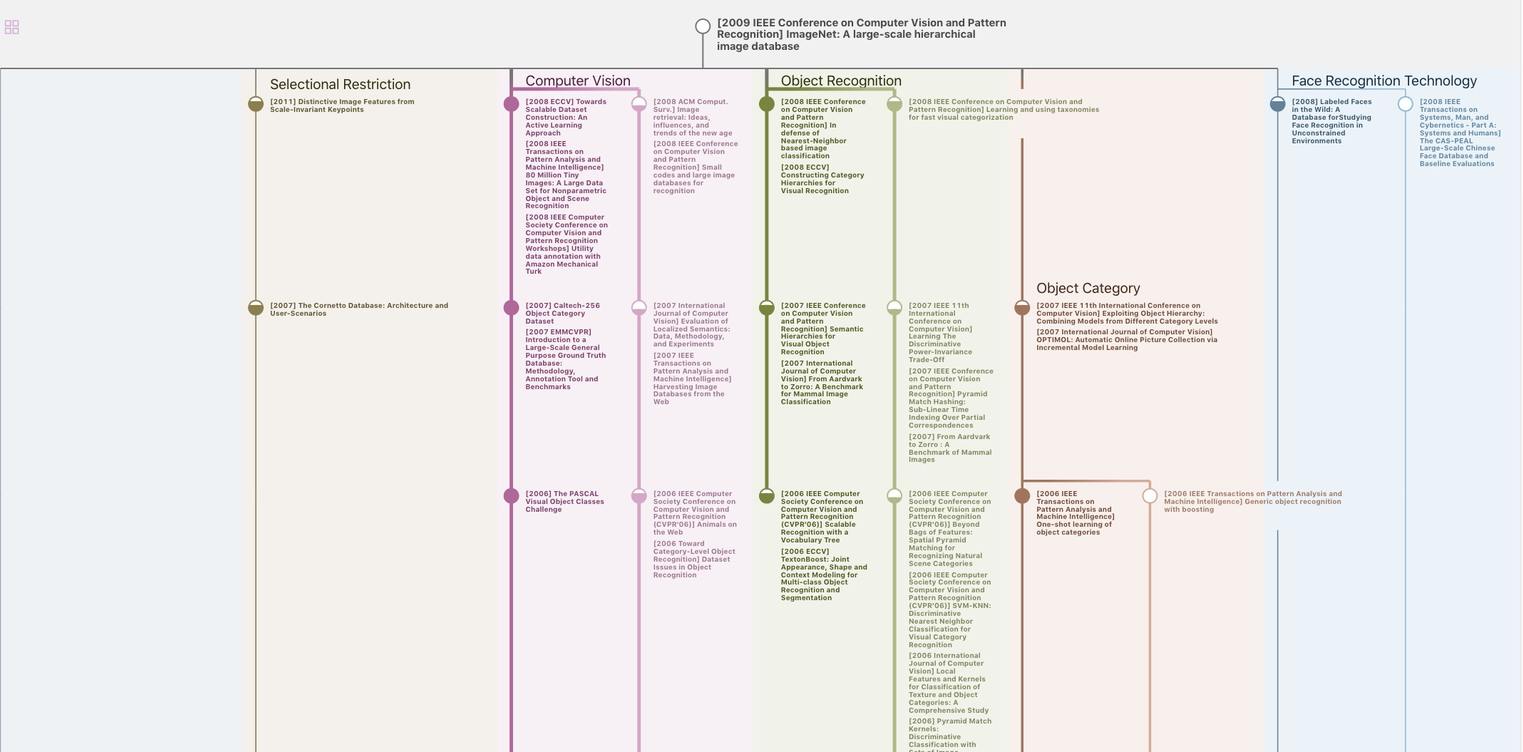
生成溯源树,研究论文发展脉络
Chat Paper
正在生成论文摘要