Neural Correlates of Learning in a Linear Discriminant Analysis Brain-Computer Interface Paradigm.
Journal of neural engineering(2022)
摘要
Objective.With practice, the control of brain-computer interfaces (BCI) would improve over time; the neural correlate for such learning had not been well studied. We demonstrated here that monkeys controlling a motor BCI using a linear discriminant analysis (LDA) decoder could learn to make the firing patterns of the recorded neurons more distinct over a short period of time for different output classes to improve task performance.Approach.Using an LDA decoder, we studied two Macaque monkeys implanted with microelectrode arrays as they controlled the movement of a mobile robotic platform. The LDA decoder mapped high-dimensional neuronal firing patterns linearly onto a lower-dimensional linear discriminant (LD) space, and we studied the changes in the spatial coordinates of these neural signals in the LD space over time, and their correspondence to trial performance. Direction selectivity was quantified with permutation feature importance (FI).Main results.We observed that, within individual sessions, there was a tendency for the points in the LD space encoding different directions to diverge, leading to fewer misclassification errors, and, hence, improvement in task accuracy. Accuracy was correlated with the presence of channels with strong directional preference (i.e. high FI), as well as a varied population code (i.e. high variance in FI distribution).Significance.We emphasized the importance of studying the short-term/intra-sessional variations in neural representations during the use of BCI. Over the course of individual sessions, both monkeys could modulate their neural activities to create increasingly distinct neural representations.
更多查看译文
关键词
brain-computer interface,neural implant,BCI,BMI,neuroprosthesis,cortical implant,brain-machine interface
AI 理解论文
溯源树
样例
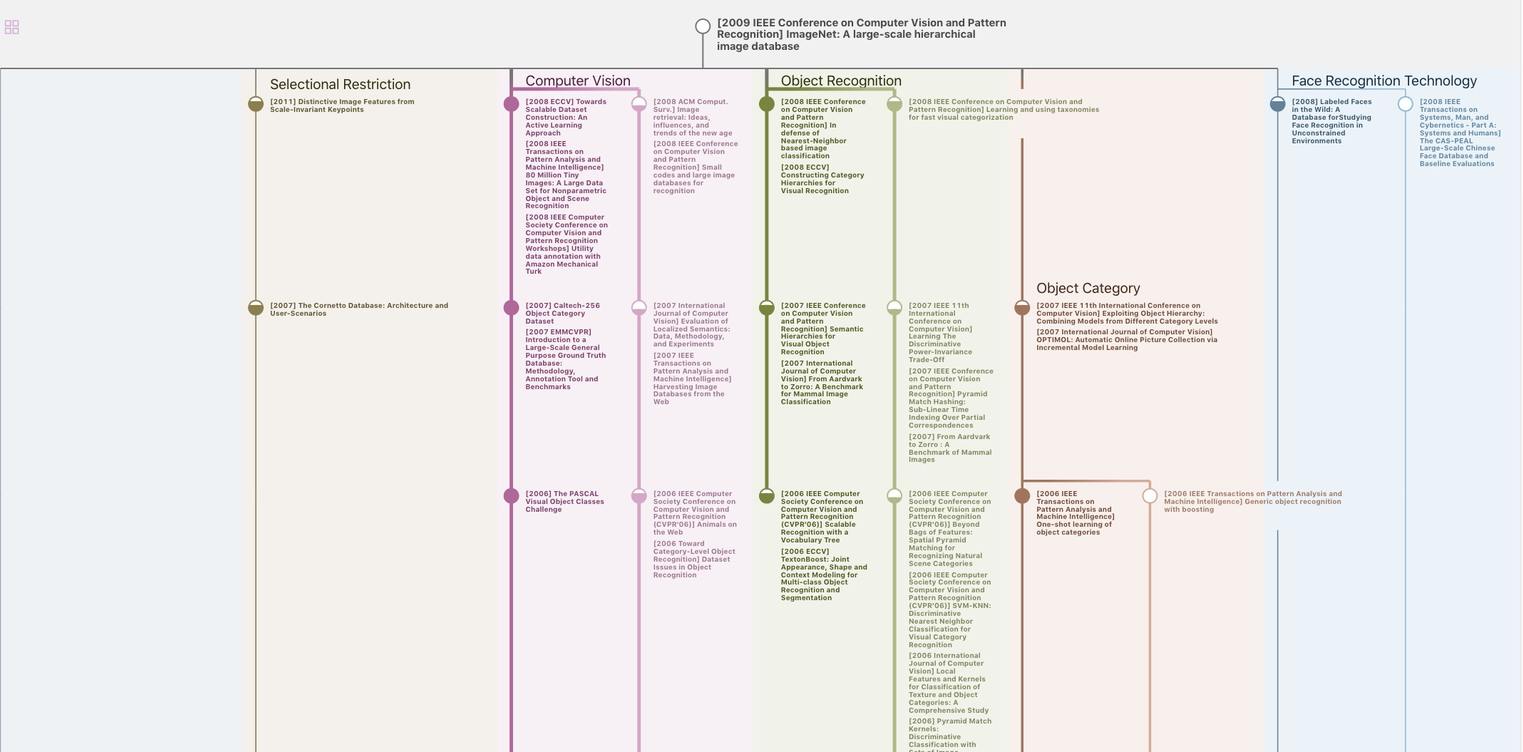
生成溯源树,研究论文发展脉络
Chat Paper
正在生成论文摘要