Huber inversion-based reverse-time migration with de-primary imaging condition and curvelet-domain sparse constraint
Petroleum Science(2022)
摘要
Least-squares reverse-time migration (LSRTM) formulates reverse-time migration (RTM) in the least-squares inversion framework to obtain the optimal reflectivity image. It can generate images with more accurate amplitudes, higher resolution, and fewer artifacts than RTM. However, three problems still exist: (1) inversion can be dominated by strong events in the residual; (2) low-wavenumber artifacts in the gradient affect convergence speed and imaging results; (3) high-wavenumber noise is also amplified as iteration increases. To solve these three problems, we have improved LSRTM: firstly, we use Huber-norm as the objective function to emphasize the weak reflectors during the inversion; secondly, we adapt the de-primary imaging condition to remove the low-wavenumber artifacts above strong reflectors as well as the false high-wavenumber reflectors in the gradient; thirdly, we apply the L1-norm sparse constraint in the curvelet-domain as the regularization term to suppress the high-wavenumber migration noise. As the new inversion objective function contains the non-smooth L1-norm, we use a modified iterative soft thresholding (IST) method to update along the Polak-Ribière conjugate-gradient direction by using a preconditioned non-linear conjugate-gradient (PNCG) method. The numerical examples, especially the Sigsbee2A model, demonstrate that the Huber inversion-based RTM can generate high-quality images by mitigating migration artifacts and improving the contribution of weak reflection events.
更多查看译文
关键词
Least-squares reverse-time migration,Huber-norm,Sparse constraint,Curvelet transform,Iterative soft thresholding
AI 理解论文
溯源树
样例
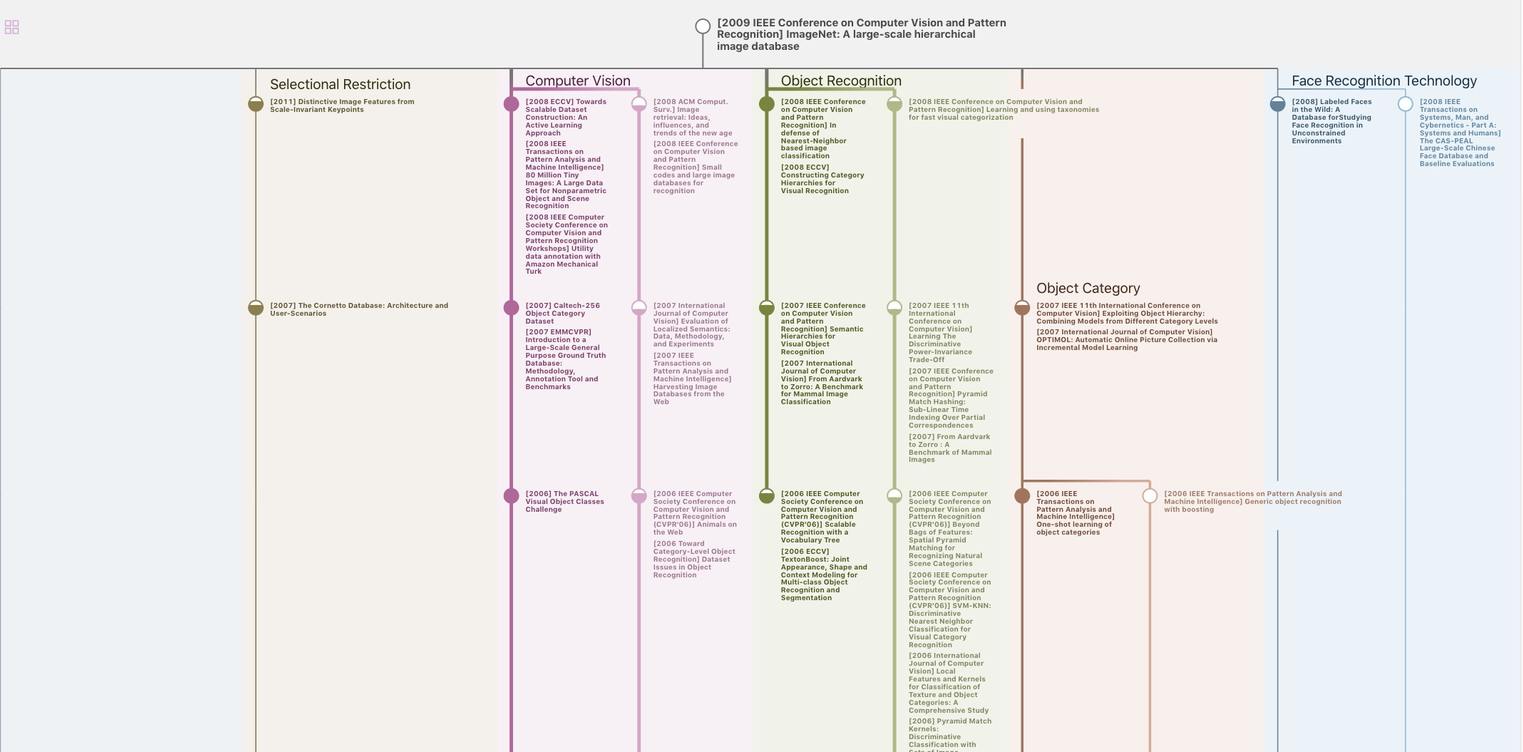
生成溯源树,研究论文发展脉络
Chat Paper
正在生成论文摘要