Dynamic traffic prediction for urban road network with the interpretable model
Physica A: Statistical Mechanics and its Applications(2022)
摘要
Dynamic traffic prediction is an important section of the urban intelligent transportation system. Although there have been many studies in this area, it is still a challenge for the urban road network considering the complexity of urban traffic and the lack of high-quality traffic data. Electronic Registration Identification of Vehicles (ERI) is an emerging traffic information acquisition technology based on Radio Frequency Identification (RFID). It can identify each vehicle accurately and generate high-quality traffic data. We employ ERI data to realize the dynamic prediction of traffic density and travel time for the urban road network. First of all, we study the temporal characteristics model of traffic through the Markov chain. Secondly, combining the Expectation–Maximization algorithm and logistic regression classifier, we classify the training data into different traffic scenes and build the spatial characteristics model for each traffic scene. The model parameters are obtained by the particle swarm optimization algorithm. Then, the trained temporal and spatial models are combined to conduct dynamic traffic prediction. Finally, the real data of Chongqing is utilized to verify the proposed method. The experimental results show that the proposed method has a good prediction accuracy and is suitable for all kinds of roads in the road network. Besides, the constructed model has good interpretability for real traffic.
更多查看译文
关键词
Electronic Registration Identification of vehicles,Travel time,Traffic density,Particle swarm optimization,Logistic regression classifier
AI 理解论文
溯源树
样例
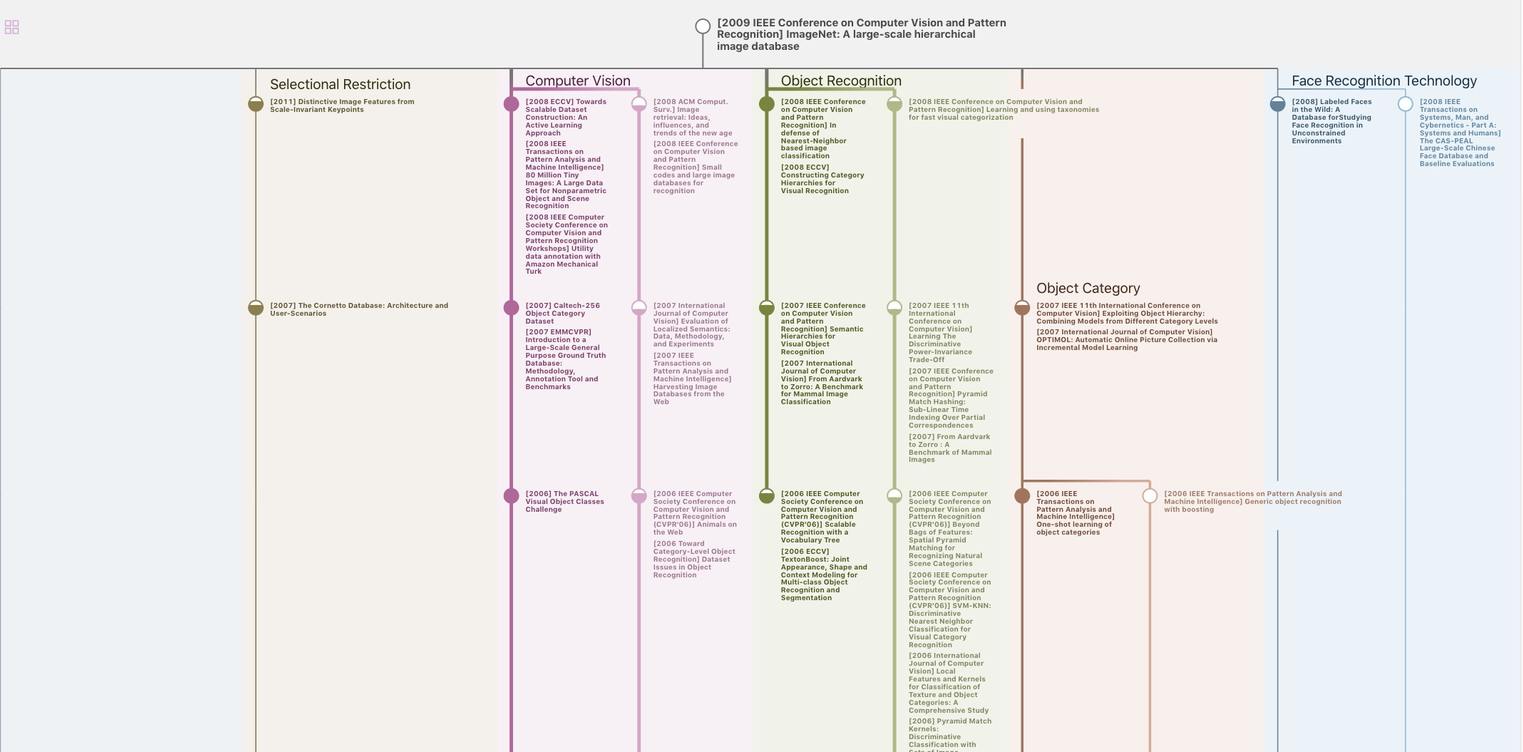
生成溯源树,研究论文发展脉络
Chat Paper
正在生成论文摘要