Machine learnt patterns in rhodium-catalysed asymmetric Michael addition using chiral diene ligands
Results in Chemistry(2022)
摘要
Interpretable featurisation allows Quantitative Structure-Property Relationships (QSPR) between chiral diene ligand structures and product stereoselectivities in rhodium-catalysed asymmetric Michael additions (RhCASA). The machine learning approach developed herein is simple to implement yet provides instructive insights and useful predictions (q2/R2 0.7–0.8). These simple interpretable features are found to give comparable predictive ability to both commercial (Dragon) and molecular signature descriptor library approaches. Strong insights into the specific areas of the chiral diene ligand that engender improved RhCASA process enantioselectivity are achieved. These were more interpretable, to synthetic chemists, than existing ML approaches, greatly facilitating design of new chiral diene-ligands.
更多查看译文
AI 理解论文
溯源树
样例
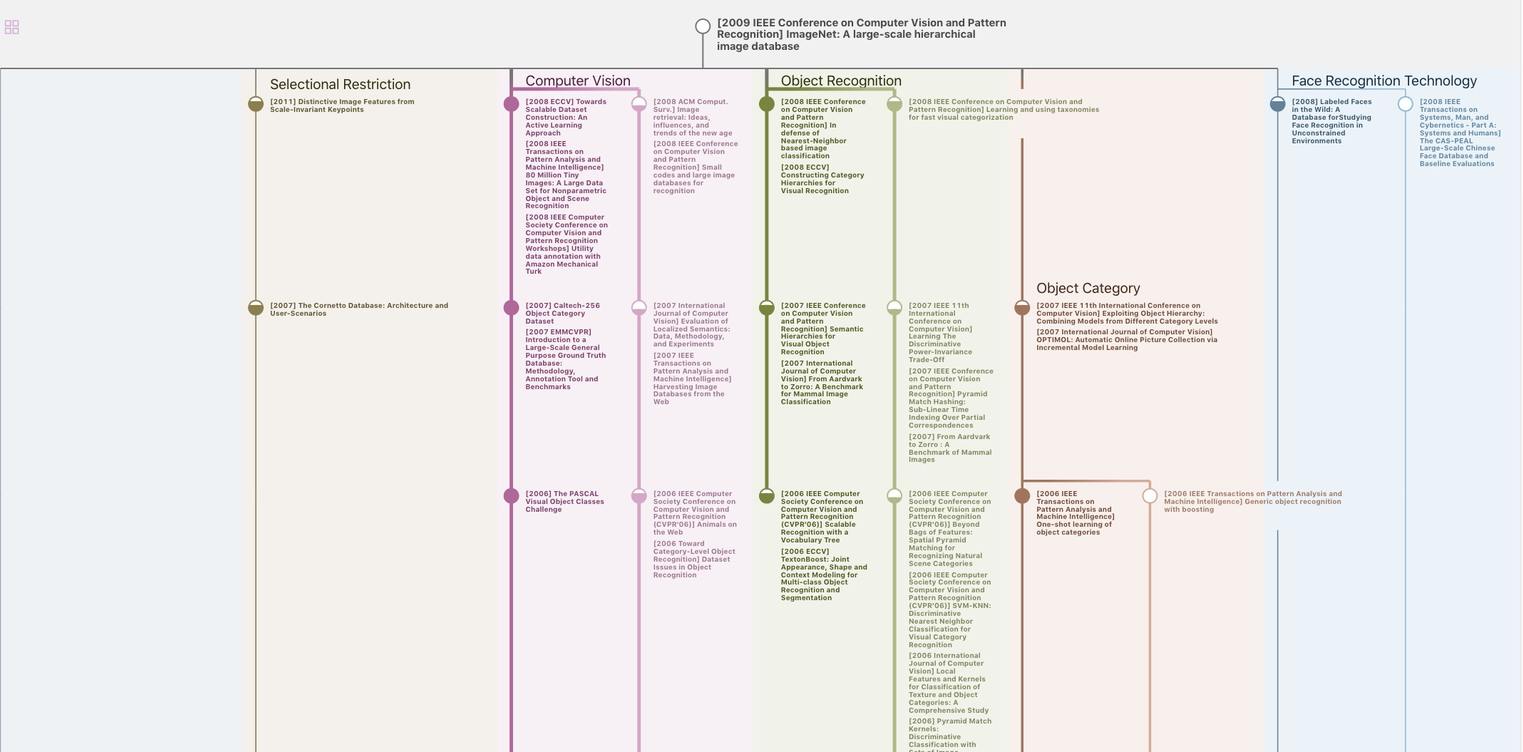
生成溯源树,研究论文发展脉络
Chat Paper
正在生成论文摘要