Production System Efficiency Optimization Using Sensor Data, Machine Learning-based Simulation and Genetic Algorithms
Procedia CIRP(2022)
摘要
In modern industries, there is a significant repository of sensor data, which contains a large amount of information. Unfortunately, this rich source of information is undervalued and underutilized, and its full potential is not fully exploited by modern day manufacturers. In the Industry 4.0 era, exploiting these powerful datasets is becoming critical for manufacturers’ survival and competitiveness in the age of artificial intelligence. Cooperative and mutual efforts between academia and the industrial sector to take advantage of these rich datasets have the potential to reap extraordinary benefits for business, the economy and society. Applying the latest artificial intelligence methods could increase production efficiencies and reduce environmental impacts. In view of the availability of large amounts of sensor data and its lack of full utilization, this research proposes an artificial intelligence solution that combines data envelopment analysis (DEA), machine learning-based simulation and genetic algorithms to optimize the efficiency of production systems through recommendations of the optimal model settings. First, DEA is used to identify the efficient and inefficient states of a production system, this information is input to the second step to build a machine learning model that makes predictions through simulations and production efficiency scenarios. Then, a genetic algorithm proposes an optimal scenario with the corresponding settings. The main research contribution of this proposed solution is its unique combination of DEA with machine learning models and genetic algorithms.
更多查看译文
关键词
Industry 4.0,Machine Learning,Data Envelopment Analysis,Genetic Algorithims
AI 理解论文
溯源树
样例
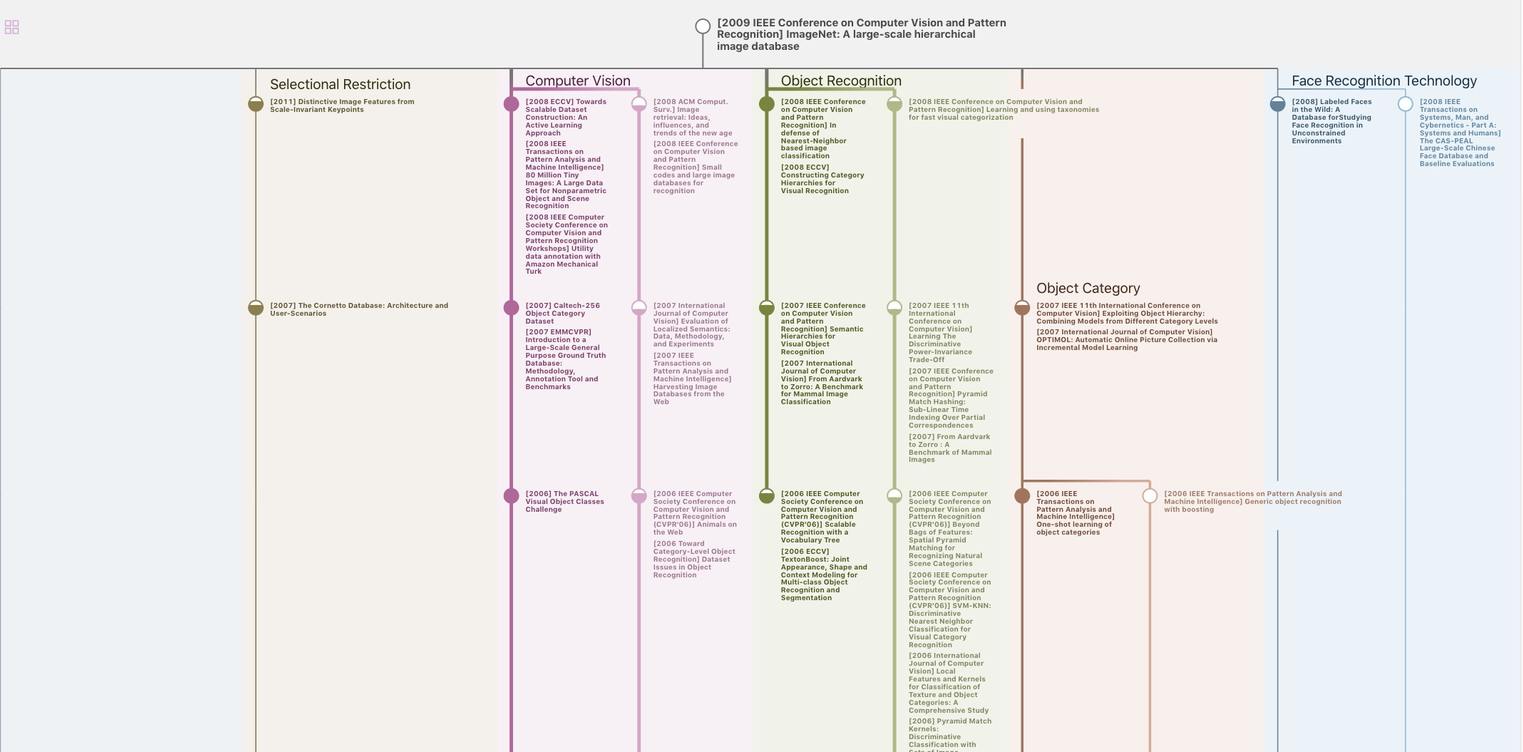
生成溯源树,研究论文发展脉络
Chat Paper
正在生成论文摘要