Tripletformer for Probabilistic Interpolation of Irregularly sampled Time Series
arxiv(2022)
摘要
Irregularly sampled time series data with missing values is observed in many
fields like healthcare, astronomy, and climate science. Interpolation of these
types of time series is crucial for tasks such as root cause analysis and
medical diagnosis, as well as for smoothing out irregular or noisy data. To
address this challenge, we present a novel encoder-decoder architecture called
"Tripletformer" for probabilistic interpolation of irregularly sampled time
series with missing values. This attention-based model operates on sets of
observations, where each element is composed of a triple of time, channel, and
value. The encoder and decoder of the Tripletformer are designed with attention
layers and fully connected layers, enabling the model to effectively process
the presented set elements. We evaluate the Tripletformer against a range of
baselines on multiple real-world and synthetic datasets and show that it
produces more accurate and certain interpolations. Results indicate an
improvement in negative loglikelihood error by up to 32
and 85
best model.
更多查看译文
关键词
Multivariate Time Series,Irregularly Sampled Time Series with Missing Values,Probabilistic Interpolation
AI 理解论文
溯源树
样例
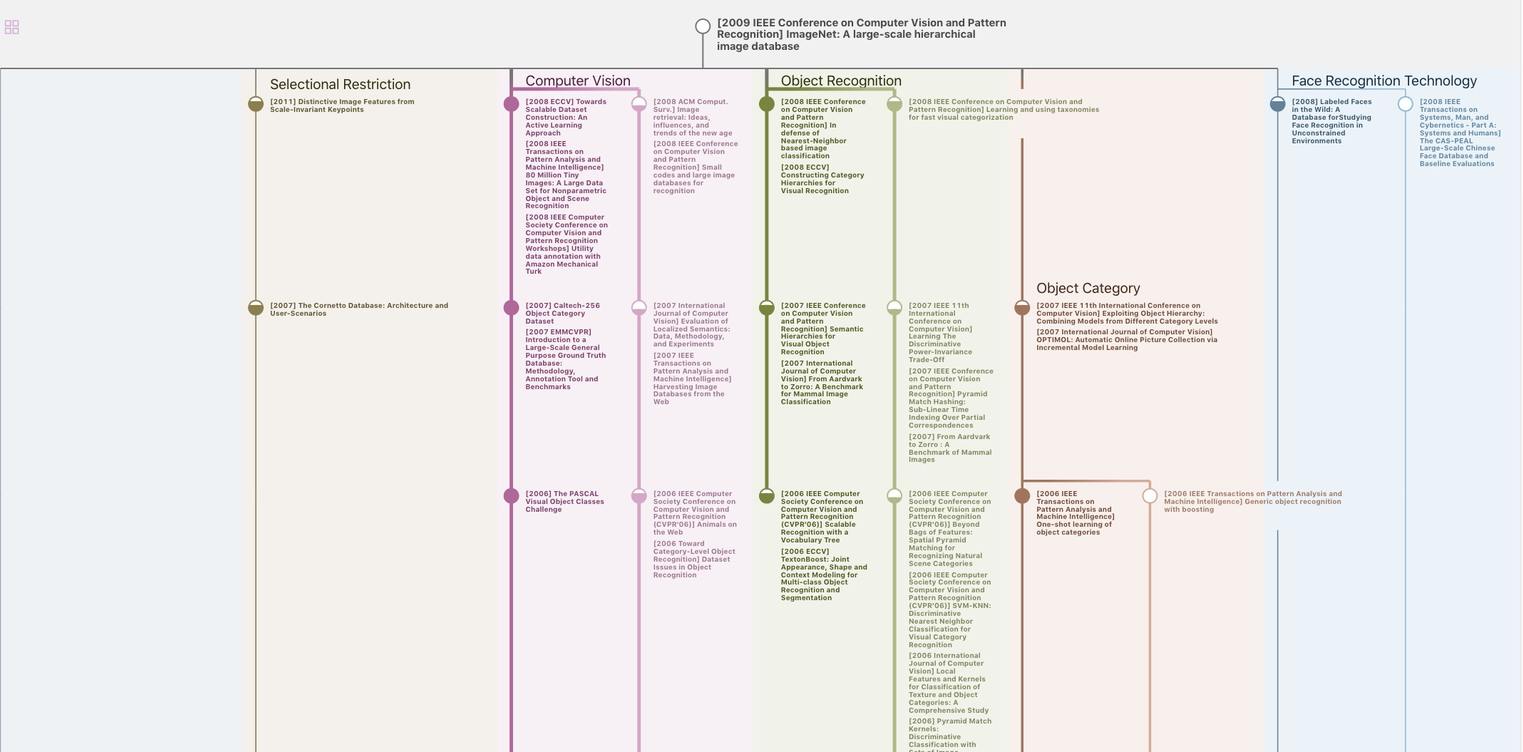
生成溯源树,研究论文发展脉络
Chat Paper
正在生成论文摘要