Text Characterization Toolkit
arxiv(2022)
摘要
In NLP, models are usually evaluated by reporting single-number performance scores on a number of readily available benchmarks, without much deeper analysis. Here, we argue that - especially given the well-known fact that benchmarks often contain biases, artefacts, and spurious correlations - deeper results analysis should become the de-facto standard when presenting new models or benchmarks. We present a tool that researchers can use to study properties of the dataset and the influence of those properties on their models' behaviour. Our Text Characterization Toolkit includes both an easy-to-use annotation tool, as well as off-the-shelf scripts that can be used for specific analyses. We also present use-cases from three different domains: we use the tool to predict what are difficult examples for given well-known trained models and identify (potentially harmful) biases and heuristics that are present in a dataset.
更多查看译文
关键词
text,characterization
AI 理解论文
溯源树
样例
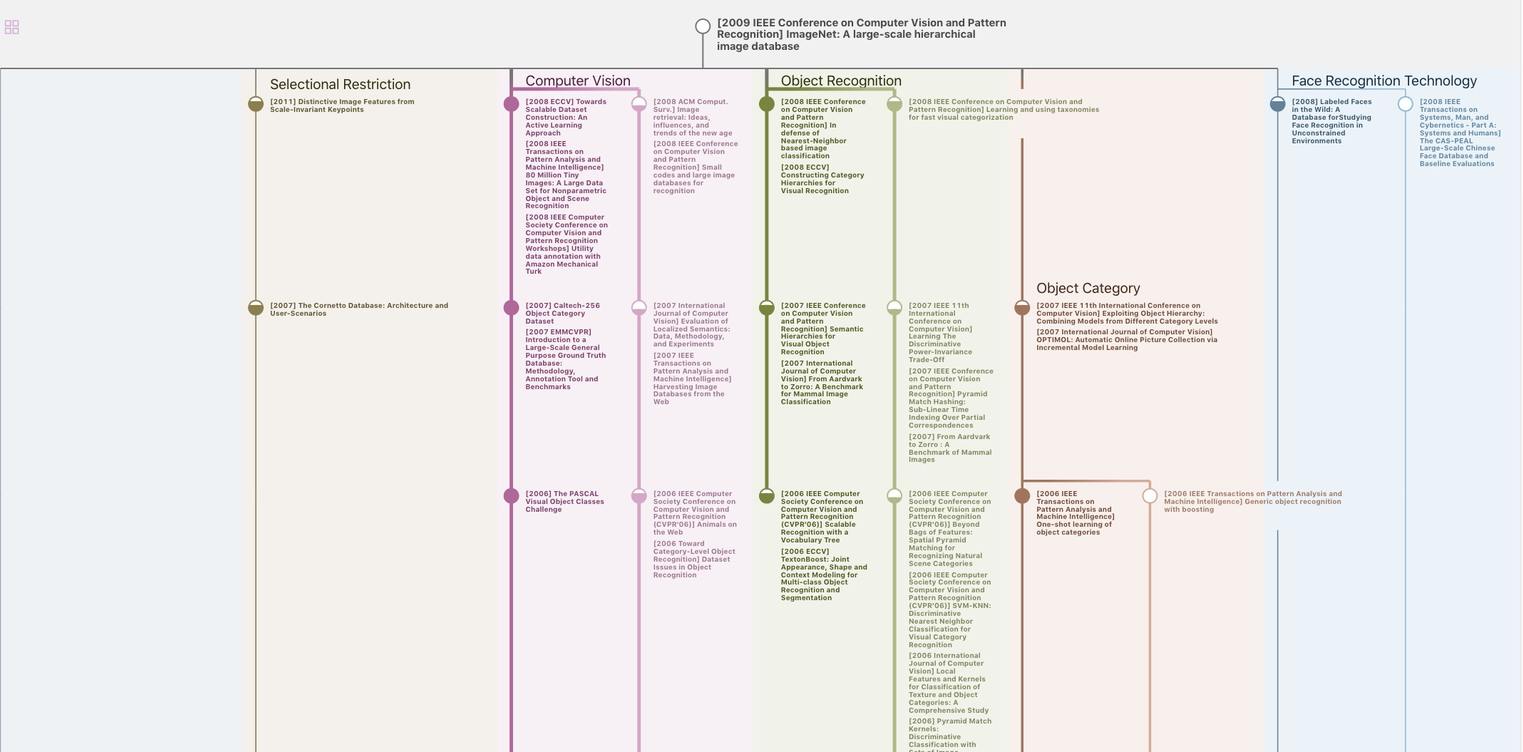
生成溯源树,研究论文发展脉络
Chat Paper
正在生成论文摘要