Restoring Lesions in Low-Dose Computed Tomography Images of COVID-19 Using Deep Learning
6th Kuala Lumpur International Conference on Biomedical Engineering 2021(2022)
摘要
The use of Low-dose Computed Tomography (LDCT) in clinical medicine for diagnosis and treatment planning is widespread due to the minimal exposure of patients to radiation. Also, recent studies have confirmed that LDCT is a feasible medical imaging modality for diagnosing COVID-19 cases. In general, X-ray tube current is being reduced to acquire the LDCT images. Reduction of the X-ray flux introduces the Quantum noise into the generated LDCT images and, as a result, it produces visually low-quality CT images. Therefore, it is challenging to differentiate the lesions in the diagnosis of COVID-19 patients using the LDCT images due to low contrast and failure to preserve the subtle structures. Therefore, in this study, we proposed a Deep Learning (DL) model based on the Generative Adversarial Network (GAN) for post-processing the LDCT images to enhance their visual quality. In this proposed model, the generator network is designed as a U-net to generate the restored CT images by filter out the noise. Also, the discriminator network follows a patch-GAN model to discriminate the real and generated images while preserving the texture details. The quantitative and qualitative results demonstrated the effectiveness of noise suppression and structure preservation of the proposed DL method. Hence, it provides an acceptable quality improvement for LDCT images to discriminate the lesions for diagnosing the COVID-19 positive cases.
更多查看译文
关键词
Low-dose computed tomography,Generative adversarial network,Lesion discrimination,LDCT denoising
AI 理解论文
溯源树
样例
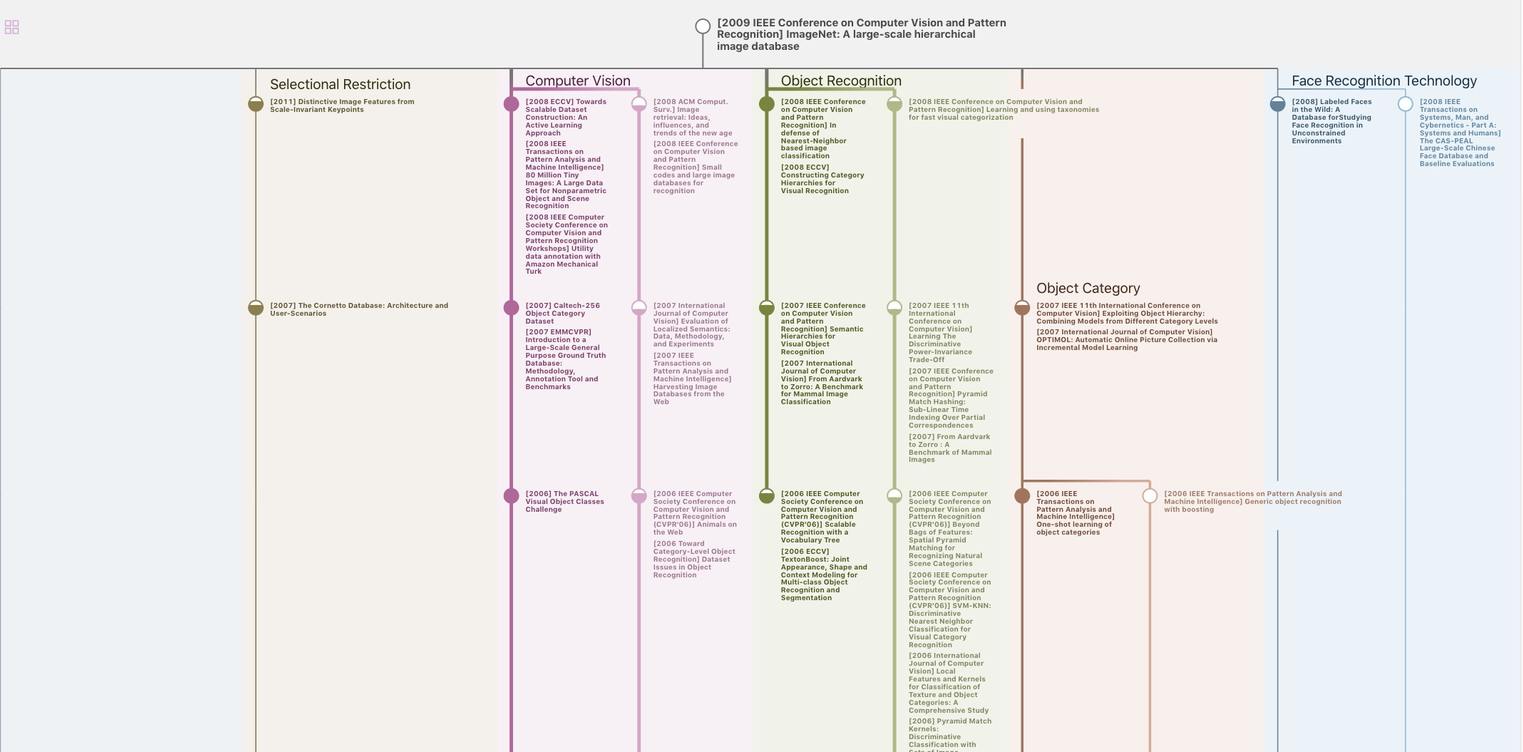
生成溯源树,研究论文发展脉络
Chat Paper
正在生成论文摘要