Disentangled Representation Learning from Videos for Facial Action Unit Detection
The International Conference on Image, Vision and Intelligent Systems (ICIVIS 2021)(2022)
摘要
Facial Action Unit (AU) detection is a challenging task for the reason that AU features extracted from videos always entangle other inevitable variations, including head posture motion characteristics, which is even much more intense than facial actions, and individual facial features because of race, age, gender or face shape. These AU-unrelated features would adverse the AU detection performance. To achieve better performance of AU detection, we proposed a novel Feature Disentangled Autoencoder (FDAE) to learn more discriminative facial action representation from large amounts of videos. Different from previous approaches, FDAE disentangled AU-related features, head posture motion characteristics and identity code characteristics to eliminate the impact of irrelevant factors. At the same time, we added two classifiers to discriminate the AU embedding and identity code embedding respectively to accelerate training process and make the model more stable and robust. Experiments on BP4D and DISFA demonstrated that the learned representation is discriminative, where FDAE outperformed or was comparable with existing representation learning method for AU detection.
更多查看译文
关键词
Action units (AUs), Facial action unit detection, Representation learning, Feature disentangled autoencoder (FDAE)
AI 理解论文
溯源树
样例
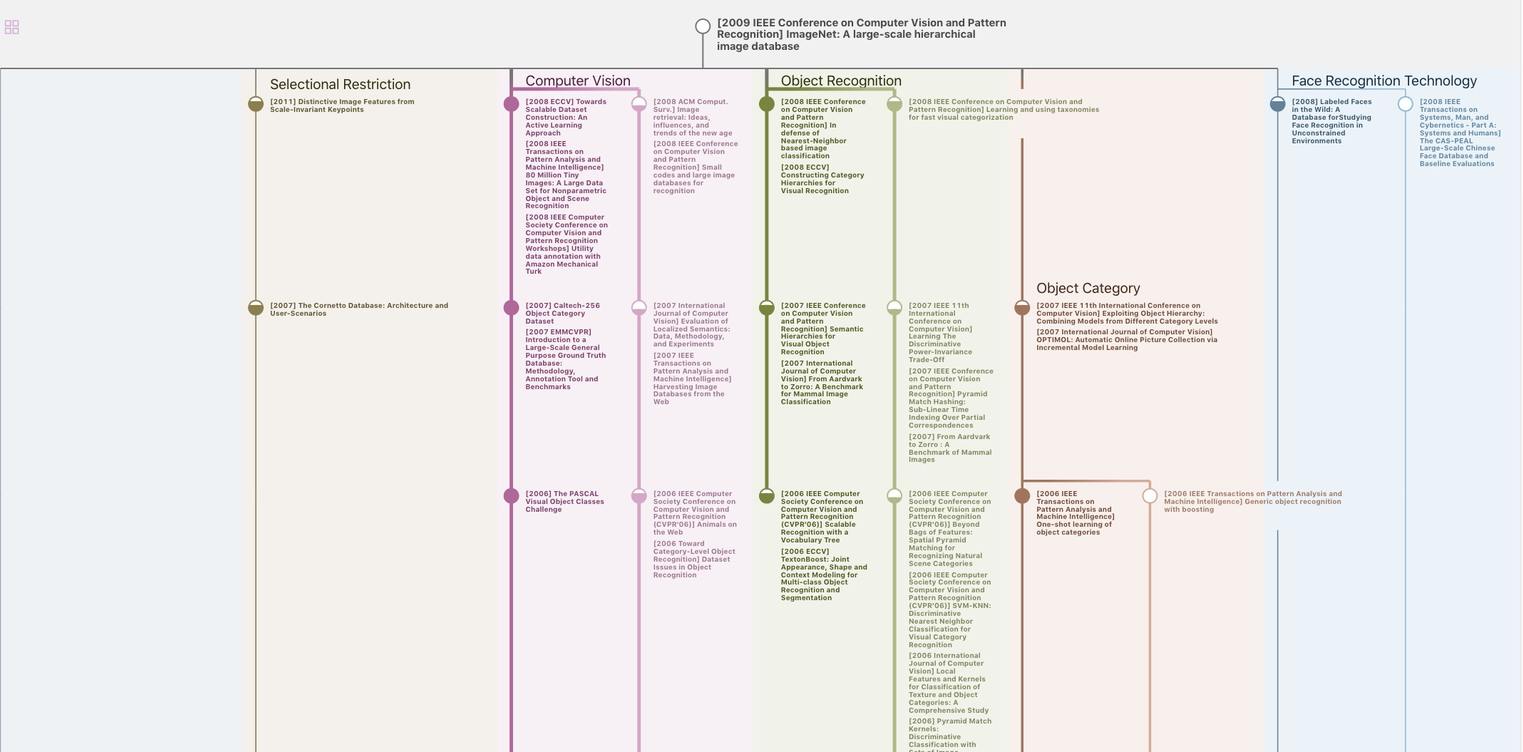
生成溯源树,研究论文发展脉络
Chat Paper
正在生成论文摘要