FedEWA: Federated Learning with Elastic Weighted Averaging
2022 International Joint Conference on Neural Networks (IJCNN)(2022)
摘要
Federated Learning (FL) offers a novel distributed machine learning context whereby a global model is collaboratively learned through edge devices without violating data privacy. However, intrinsic data heterogeneity in the federated network can induce model heterogeneity, thus posing a great challenge to the server-side model aggregation performance. Existing FL algorithms widely adopt model-wise weighted averaging for client models to generate the new global model, which emphasizes the importance of the holistic model but ignores the importance of distinctions between internal parameters of various client models. In this paper, we propose a novel parameter-wise elastic weighted averaging aggregation approach to realize the rapid fusion of heterogeneous client models. Specifically, each client evaluates the importance of model internal parameters in the model update and obtains the corresponding parameter importance coefficient vector; the server implements the parameter-wise weighted averaging for each parameter based on their importance coefficient vectors, thereby aggregating a new global model. Extensive experiments on MNIST and CIFAR-10 datasets with diverse network architectures and hyper-parameter combinations show that our proposed algorithm outperforms the existing state-of-the-art FL algorithms on the performance of heterogeneous model fusion.
更多查看译文
关键词
distributed learning,federated learning,data heterogeneity,Non-IID data,heterogeneous model fusion
AI 理解论文
溯源树
样例
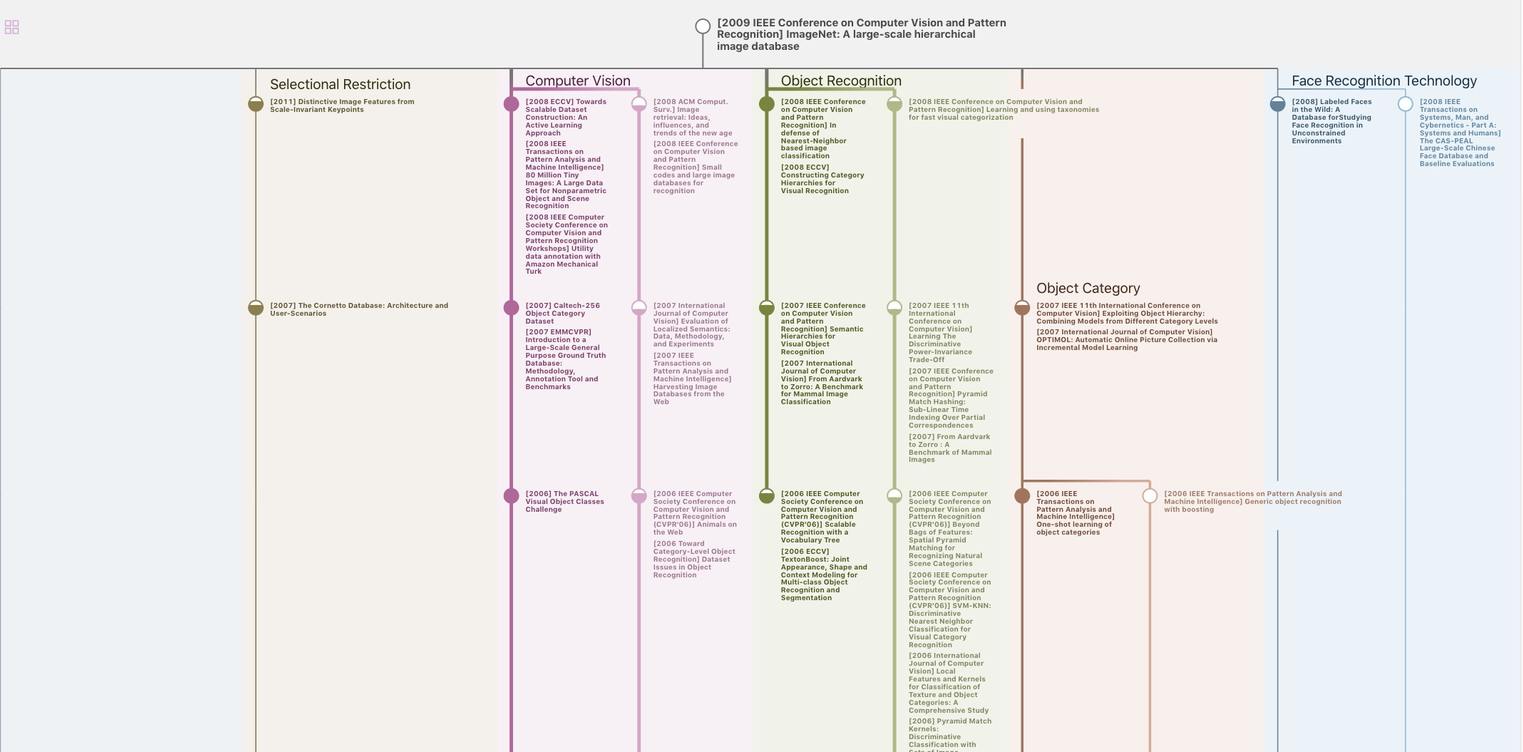
生成溯源树,研究论文发展脉络
Chat Paper
正在生成论文摘要