NVRadarNet: Real-Time Radar Obstacle and Free Space Detection for Autonomous Driving
2023 IEEE INTERNATIONAL CONFERENCE ON ROBOTICS AND AUTOMATION, ICRA(2023)
摘要
Detecting obstacles is crucial for safe and efficient autonomous driving. To this end, we present NVRadarNet, a deep neural network (DNN) that detects dynamic obstacles and drivable free space using automotive RADAR sensors. The network utilizes temporally accumulated data from multiple RADAR sensors to detect dynamic obstacles and compute their orientation in a top-down bird's-eye view (BEV). The network also regresses drivable free space to detect unclassified obstacles. Our DNN is the first of its kind to utilize sparse RADAR signals in order to perform obstacle and free space detection in real time from RADAR data only. The network has been successfully used for perception on our autonomous vehicles in real self-driving scenarios. The network runs faster than real time on an embedded GPU and shows good generalization across geographic regions.
更多查看译文
关键词
automotive RADAR sensors,autonomous vehicles,deep neural network,detecting obstacles,DNN,drivable free space,dynamic obstacles,efficient autonomous driving,free space detection,multiple RADAR sensors,NVRadarNet,RADAR data,real-time radar obstacle,safe driving,sparse RADAR signals,unclassified obstacles
AI 理解论文
溯源树
样例
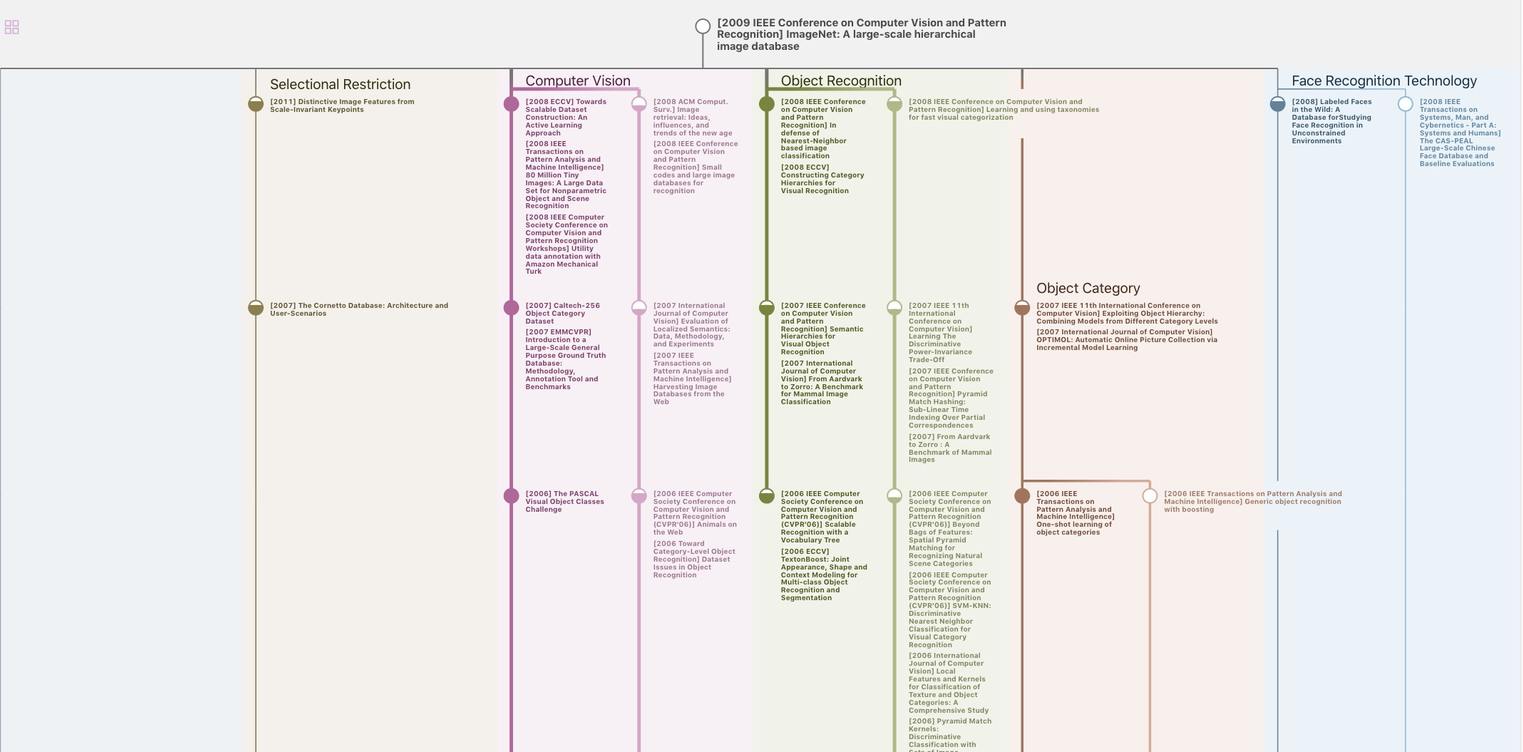
生成溯源树,研究论文发展脉络
Chat Paper
正在生成论文摘要