Extraction-based Deep Learning Reconstruction of Interior Tomography
arxiv(2022)
摘要
Interior tomography is a typical strategy for radiation dose reduction in computed tomography, where only a certain region-of-interest (ROI) is scanned. However, given the truncated projection data, ROI reconstruction by conventional analytical algorithms may suffer from severe cupping artifacts. In this paper, we proposed a new extraction-based deep learning method for the reconstruction of interior tomography. Our approach works in dual domains where a sinogram-domain network (SDNet) estimates the contribution of the exterior region to the truncated projection and an image-domain network (IDNet) further mitigates artifacts. Unlike the previous extrapolation-based methods, SDNet is intended to obtain a complete ROI-only sinogram via extraction instead of a fully non-truncated sinogram for both the ROI and exterior regions. Our experiments validated the proposed method and the results indicate that the proposed method can disclose more reliable structures. It achieved better image quality with better generalization performance than extrapolation-based methods.
更多查看译文
关键词
interior tomography,deep learning reconstruction,deep learning,extraction-based
AI 理解论文
溯源树
样例
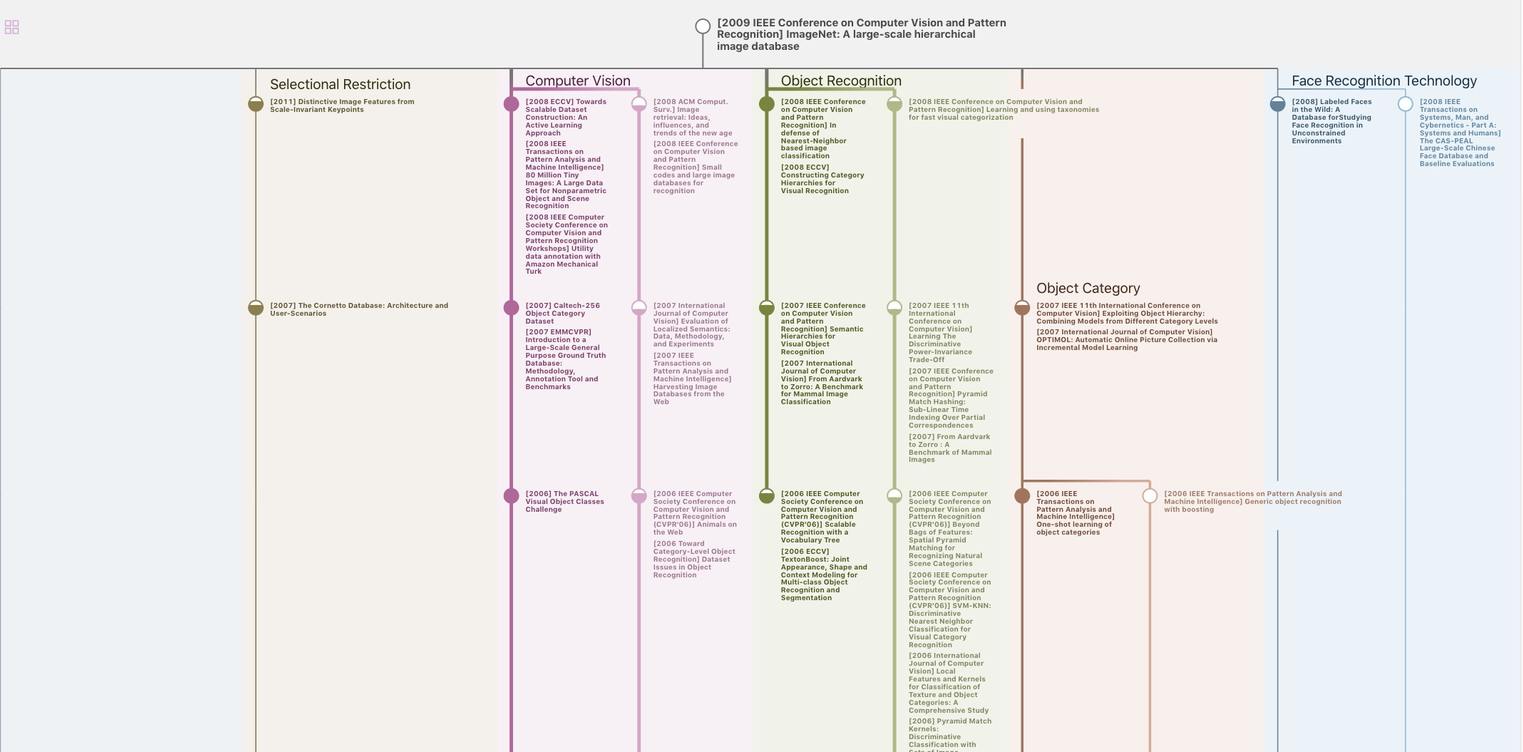
生成溯源树,研究论文发展脉络
Chat Paper
正在生成论文摘要