SSML: Spectral-Spatial Mutual-Learning-Based Framework for Hyperspectral Pansharpening.
Remote Sensing(2022)
摘要
This paper considers problems associated with the large size of the hyperspectral pansharpening network and difficulties associated with learning its spatial-spectral features. We propose a deep mutual-learning-based framework (SSML) for spectral-spatial information mining and hyperspectral pansharpening. In this framework, a deep mutual-learning mechanism is introduced to learn spatial and spectral features from each other through information transmission, which achieves better fusion results without entering too many parameters. The proposed SSML framework consists of two separate networks for learning spectral and spatial features of HSIs and panchromatic images (PANs). A hybrid loss function containing constrained spectral and spatial information is designed to enforce mutual learning between the two networks. In addition, a mutual-learning strategy is used to balance the spectral and spatial feature learning to improve the performance of the SSML path compared to the original. Extensive experimental results demonstrated the effectiveness of the mutual-learning mechanism and the proposed hybrid loss function for hyperspectral pan-sharpening. Furthermore, a typical deep-learning method was used to confirm the proposed framework’s capacity for generalization. Ideal performance was observed in all cases. Moreover, multiple experiments analysing the parameters used showed that the proposed method achieved better fusion results without adding too many parameters. Thus, the proposed SSML represents a promising framework for hyperspectral pansharpening.
更多查看译文
关键词
deep learning,image fusion,hyperspectral pansharpening,deep mutual learning
AI 理解论文
溯源树
样例
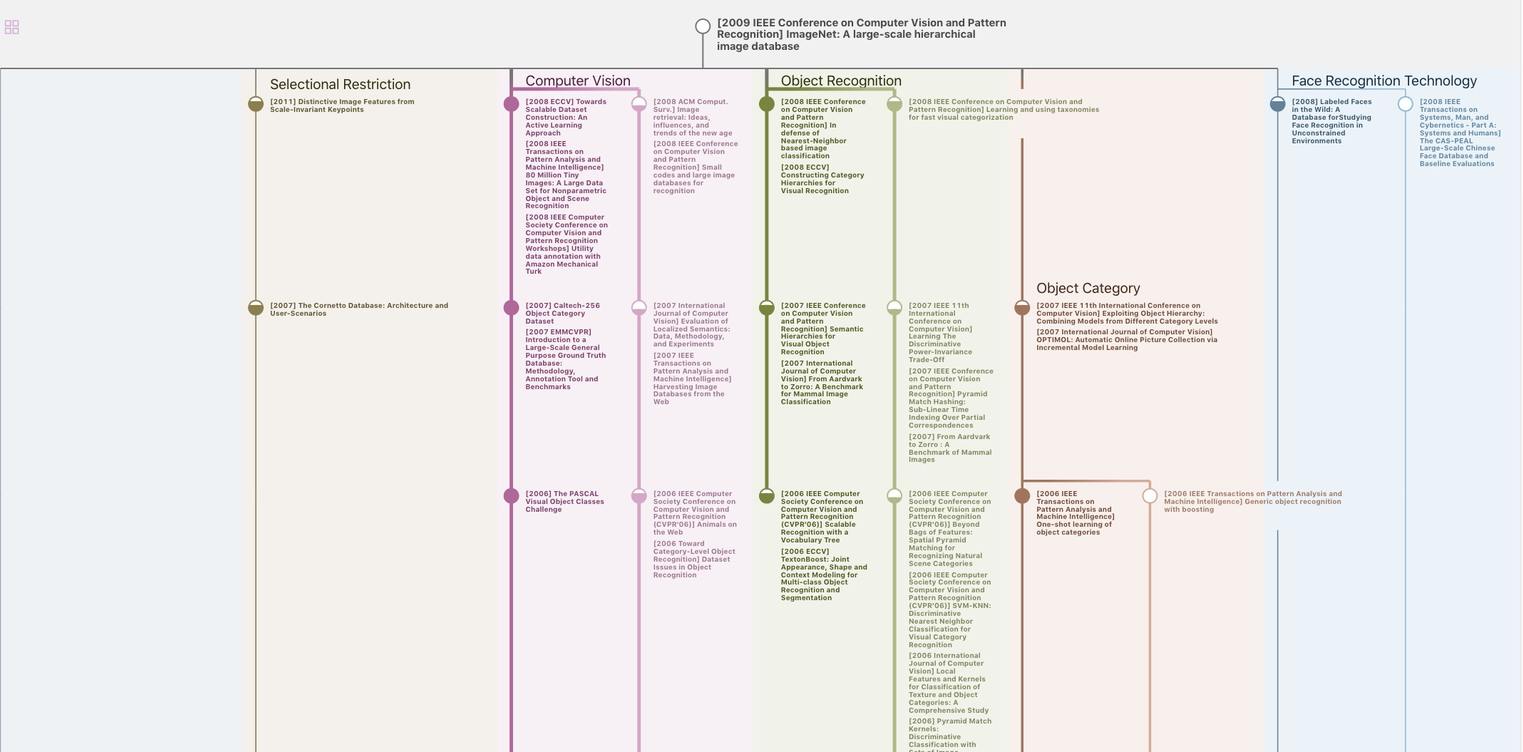
生成溯源树,研究论文发展脉络
Chat Paper
正在生成论文摘要