Integrating Pose and Mask Predictions for Multi-person in Videos.
2022 IEEE/CVF Conference on Computer Vision and Pattern Recognition Workshops (CVPRW)(2022)
摘要
In real-world applications for video editing, humans are arguably the most important objects. When editing videos of humans, the efficient tracking of fine-grained masks and body joints is the fundamental requirement. In this paper, we propose a simple and efficient system for jointly tracking pose and segmenting high-quality masks for all humans in the video. We design a pipeline that globally tracks pose and locally segments fine-grained masks. Specifically, CenterTrack is first employed to track human poses by viewing the whole scene, and then the proposed local segmentation network leverages the pose information as a powerful query to carry out high-quality segmentation. Furthermore, we adopt a highly light-weight MLP-Mixer layer within the segmentation network that can efficiently propagate the query pose throughout the region of interest with minimal overhead. For the evaluation, we collect a new benchmark called KineMask which includes various appearances and actions. The experimental results demonstrate that our method has superior fine-grained segmentation performance. Moreover, it runs at 33 fps, achieving a great balance of speed and accuracy compared to the prevailing online Video Instance Segmentation methods.
更多查看译文
关键词
mask predictions,multiperson,real-world applications,video editing,important objects,editing videos,efficient tracking,body joints,simple system,high-quality masks,globally tracks pose,segments fine-grained masks,human poses,local segmentation network leverages,pose information,powerful query,high-quality segmentation,highly light-weight MLP-Mixer layer,query pose,fine-grained segmentation performance,prevailing online Video Instance Segmentation methods
AI 理解论文
溯源树
样例
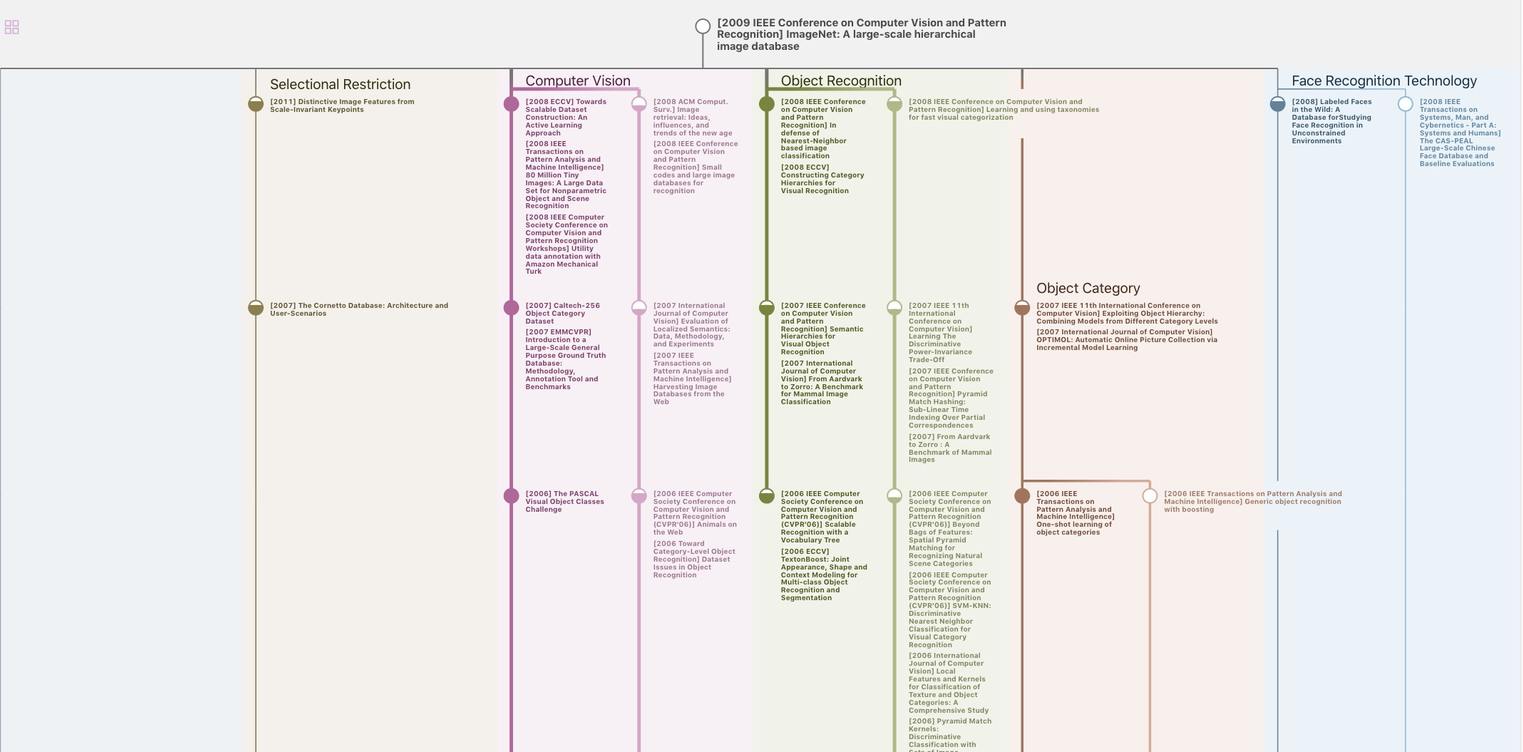
生成溯源树,研究论文发展脉络
Chat Paper
正在生成论文摘要