SpikeSEE: an Energy-Efficient Dynamic Scenes Processing Framework for Retinal Prostheses.
Neural Networks(2022)
摘要
Intelligent and low-power retinal prostheses are highly demanded in this era, where wearable and implantable devices are used for numerous healthcare applications. In this paper, we propose an energy-efficient dynamic scenes processing framework (SpikeSEE) that combines a spike representation encoding technique and a bio-inspired spiking recurrent neural network (SRNN) model to achieve intelligent processing and extreme low-power computation for retinal prostheses. The spike representation encoding technique could interpret dynamic scenes with sparse spike trains, decreasing the data volume. The SRNN model, inspired by the human retina's special structure and spike processing method, is adopted to predict the response of ganglion cells to dynamic scenes. Experimental results show that the Pearson correlation coefficient of the proposed SRNN model achieves 0.93, which outperforms the state-of-the-art processing framework for retinal prostheses. Thanks to the spike representation and SRNN processing, the model can extract visual features in a multiplication-free fashion. The framework achieves 8 times power reduction compared with the convolutional recurrent neural network (CRNN) processing-based framework. Our proposed SpikeSEE predicts the response of ganglion cells more accurately with lower energy consumption, which alleviates the precision and power issues of retinal prostheses and provides a potential solution for wearable or implantable prostheses.
更多查看译文
关键词
Retinal prostheses,Dynamic vision sensor,Spiking recurrent neural network,Wearable devices
AI 理解论文
溯源树
样例
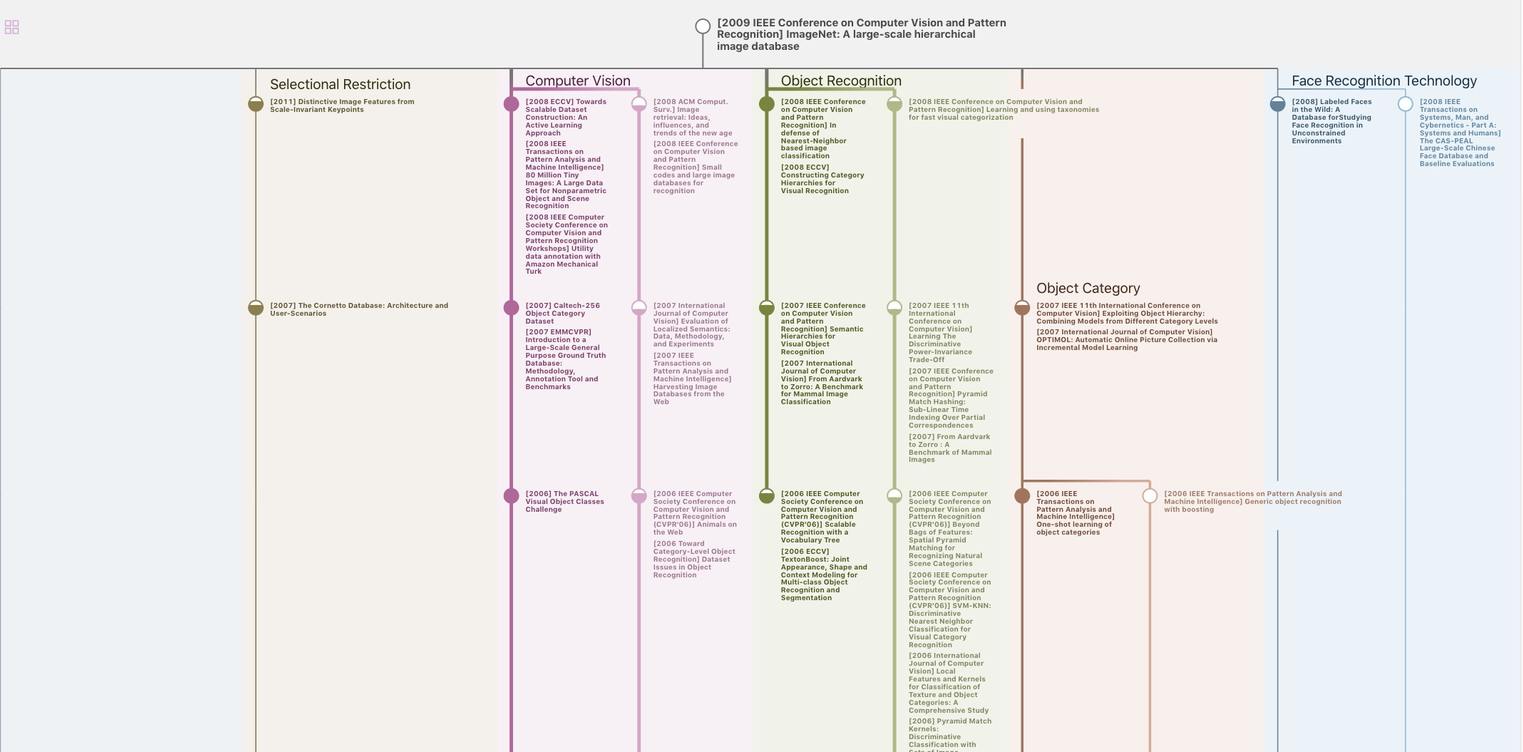
生成溯源树,研究论文发展脉络
Chat Paper
正在生成论文摘要