A Fair Performance Comparison Between Complex-Valued and Real-Valued Neural Networks for Disease Detection.
Diagnostics(2022)
Abstract
Our aim is to contribute to the classification of anomalous patterns in biosignals using this novel approach. We specifically focus on melanoma and heart murmurs. We use a comparative study of two convolution networks in the Complex and Real numerical domains. The idea is to obtain a powerful approach for building portable systems for early disease detection. Two similar algorithmic structures were chosen so that there is no bias determined by the number of parameters to train. Three clinical data sets, ISIC2017, PH2, and Pascal, were used to carry out the experiments. Mean comparison hypothesis tests were performed to ensure statistical objectivity in the conclusions. In all cases, complex-valued networks presented a superior performance for the Precision, Recall, F1 Score, Accuracy, and Specificity metrics in the detection of associated anomalies. The best complex number-based classifier obtained in the Receiving Operating Characteristic (ROC) space presents a Euclidean distance of 0.26127 with respect to the ideal classifier, as opposed to the best real number-based classifier, whose Euclidean distance to the ideal is 0.36022 for the same task of melanoma detection. The 27.46% superiority in this metric, as in the others reported in this work, suggests that complex-valued networks have a greater ability to extract features for more efficient discrimination in the dataset.
MoreTranslated text
Key words
complex-valued deep learning,complex-valued convolution neural networks,real-valued neural networks,complex numbers,fair performance comparison
AI Read Science
Must-Reading Tree
Example
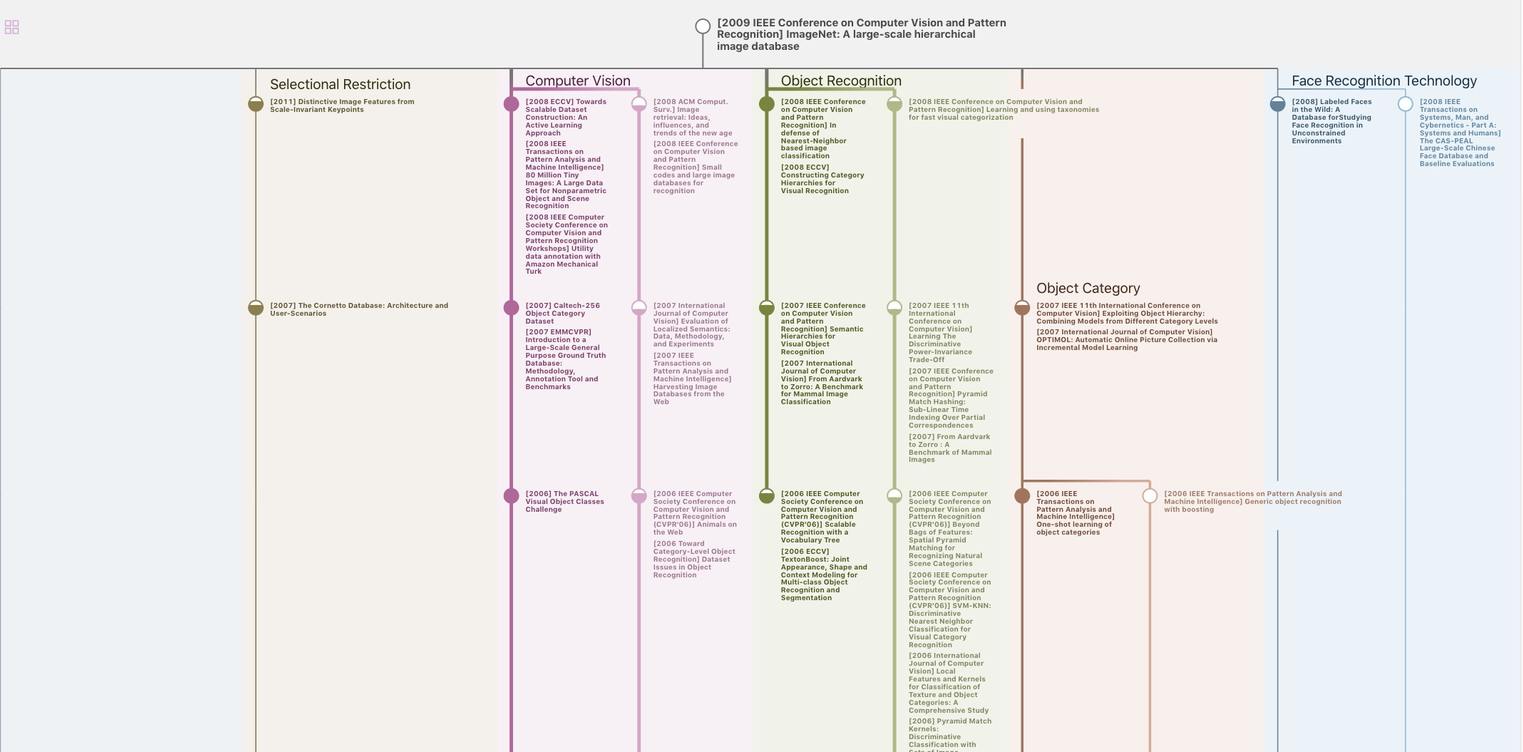
Generate MRT to find the research sequence of this paper
Chat Paper
Summary is being generated by the instructions you defined