Deep Reinforcement Learning for Dynamic Opportunistic Maintenance of Multi-Component Systems With Load Sharing
IEEE TRANSACTIONS ON RELIABILITY(2023)
摘要
Opportunistic maintenance (OM), which shows its superiority on complex multi-component systems by integrating the maintenance activities of multiple components to reduce the maintenance cost, has been widely studied over the past decade. To our knowledge, most of the existing OM works are developed based on fixed maintenance thresholds without fully utilizing the health state of the multi-component system. This article presents an OM optimization problem of multi-component systems with load sharing, solved by a modified proximal policy optimization approach based on deep reinforcement learning algorithm. The load sharing effect is reflected in the hazard rate function, which further changes the failure probability of the components. Meanwhile, the health states can be recovered by executing imperfect maintenance and corrective maintenance. The optimization problem is formulated as an infinite-horizon MDP with mixed discrete and continuous state and action space to maximize the total discounted reward, taking into account the system reliability and the maintenance cost. The difficulty caused by the mixed action space is solved by designing a parameterized action space structure and multi-task reinforcement learning framework. The effectiveness of the proposed algorithm is tested on a four-component system and a real-world scenario configured with the high-pressure feedwater heater system in the nuclear power plant. The results show that the performance of the algorithm is stable when facing large-scale problems. The algorithm proposed in this study also contributes to the imperfect maintenance optimization with state-of-the-art optimization techniques.
更多查看译文
关键词
Maintenance engineering,Inspection,Load modeling,Costs,Optimization,Degradation,Hazards,Deep reinforcement learning (DRL),imperfect maintenance,load-sharing,mixed action space,opportunistic maintenance (OM)
AI 理解论文
溯源树
样例
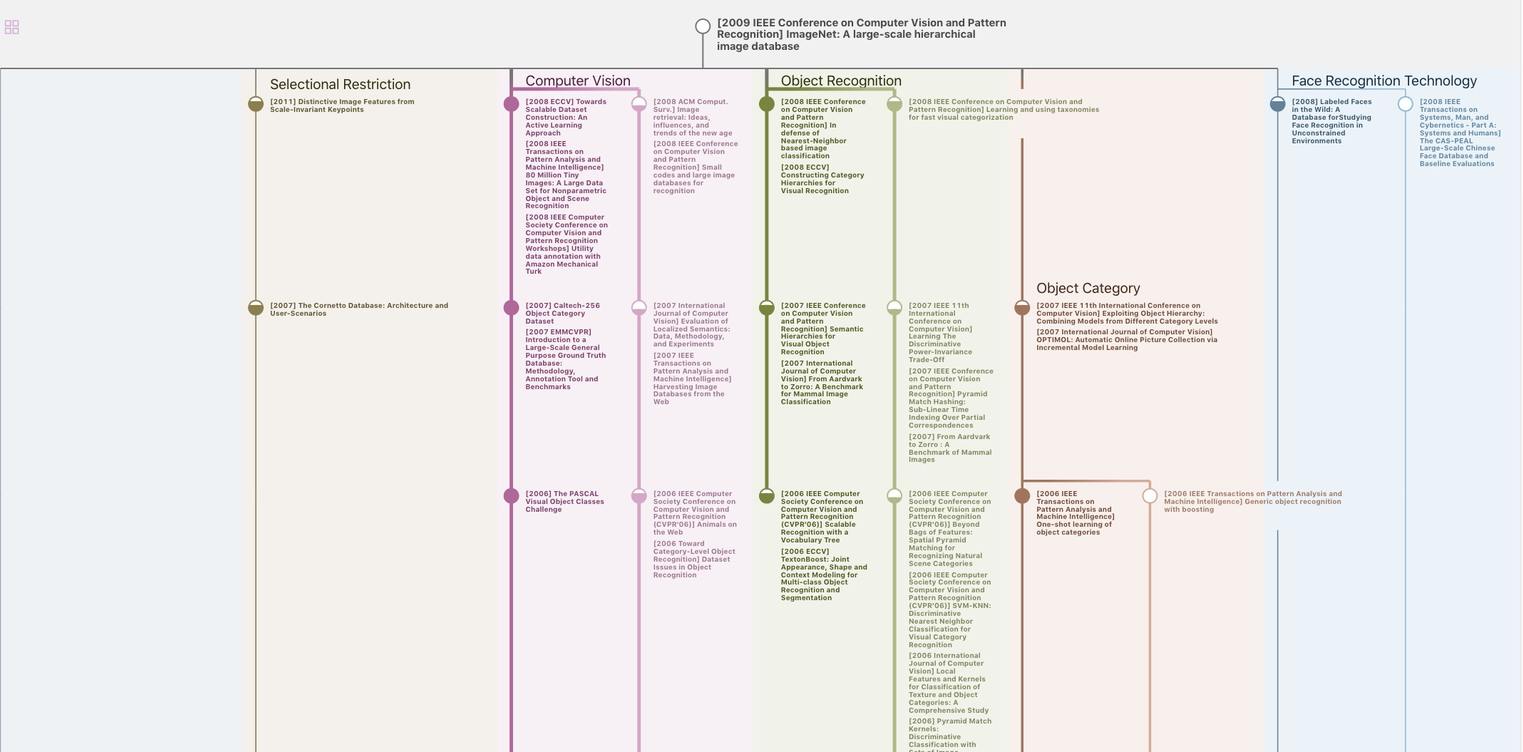
生成溯源树,研究论文发展脉络
Chat Paper
正在生成论文摘要