Hierarchical Attention-Based Context-Aware Network for Long-Term Forecasting of Chlorophyll
Applied intelligence(2022)
摘要
Chlorophyll forecasting is helpful for understanding red tides’ characteristics, thus enabling early warning. It is usually formulated as a multivariate time series forecasting problem, which aims to predict the future chlorophyll concentration with the observation of relevant exogenous factors (e.g., PH, turbidity, etc.) and historical chlorophyll. However, existing methods are difficult to satisfy the demand of chlorophyll forecasting because the complicated and changeable interaction existed in observed data. In this work, we propose a fine-grained hierarchical attention-based context-aware network (HACN). To adaptively extract significant influential factors, HACN consists of two branch networks, with one named EF-net focusing on exogenous factors and the other named TF-net executing on chlorophyll. In EF-net, factor-level attention and sequence-level attention are respectively leveraged to learn exogenous factors and time steps that are beneficial to prediction. In TF-net, a contextual long short-term memory network (Con-LSTM) is designed to nest a context-aware attention and introduce a filtration gate. Context-aware attention can capture the influence of exogenous factors series on historical chlorophyll. Filtration gate can suppress noisy information of external impact. Finally, we employ a gated fusion network to fuse the outputs of EF-net and TF-net. Experiments on two real-world datasets reveal that HACN not only outperforms state-of-the-art methods, but also provides interpretability for chlorophyll prediction.
更多查看译文
关键词
Multivariate time series,Red tide forecasting,Hierarchical attention,Deep neural network,Long-term forecasting
AI 理解论文
溯源树
样例
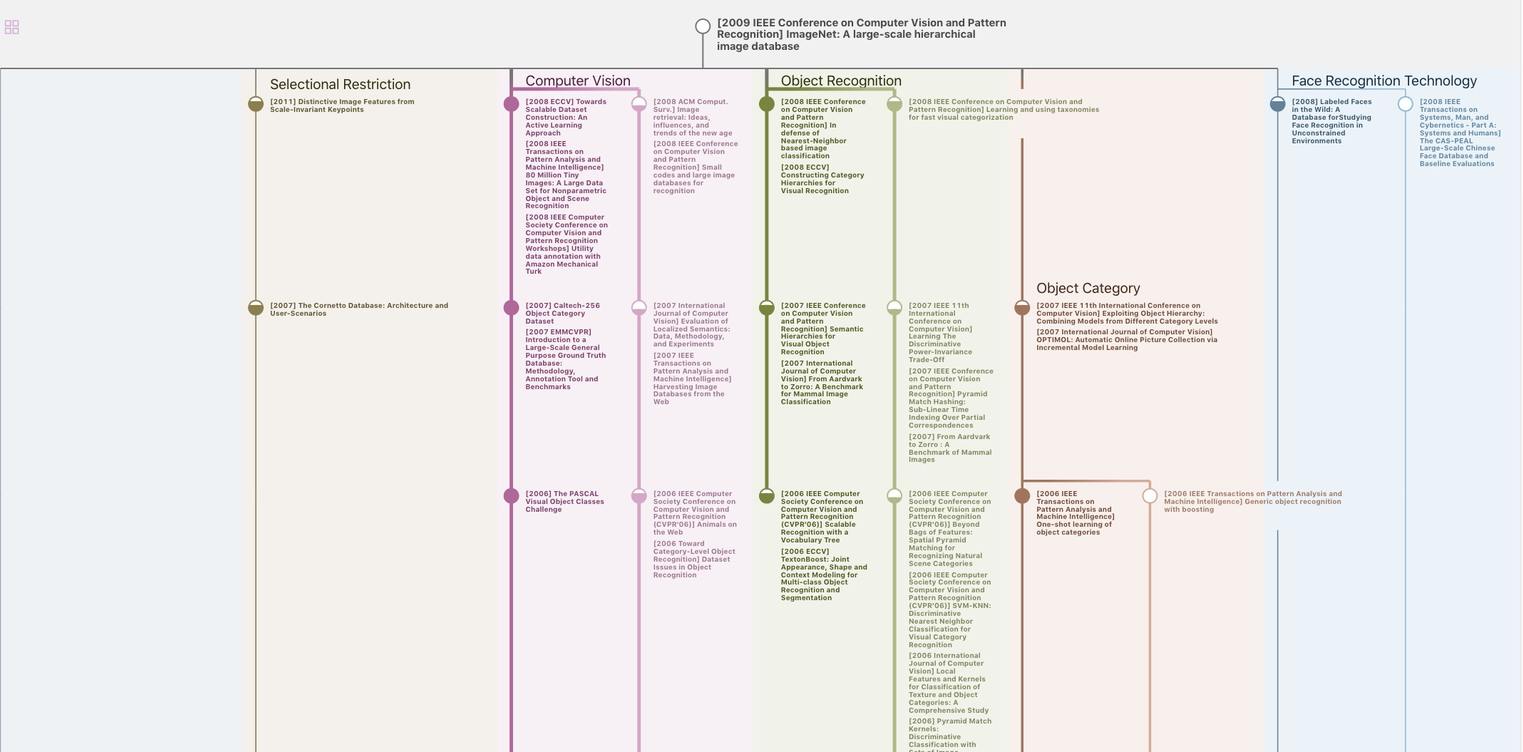
生成溯源树,研究论文发展脉络
Chat Paper
正在生成论文摘要